Identifiability and inference for copula-based semiparametric models for random vectors with arbitrary marginal distributions
arxiv(2023)
摘要
In this paper, we study the identifiability and the estimation of the parameters of a copula-based multivariate model when the margins are unknown and are arbitrary, meaning that they can be continuous, discrete, or mixtures of continuous and discrete. When at least one margin is not continuous, the range of values determining the copula is not the entire unit square and this situation could lead to identifiability issues that are discussed here. Next, we propose estimation methods when the margins are unknown and arbitrary, using pseudo log-likelihood adapted to the case of discontinuities. In view of applications to large data sets, we also propose a pairwise composite pseudo log-likelihood. These methodologies can also be easily modified to cover the case of parametric margins. One of the main theoretical result is an extension to arbitrary distributions of known convergence results of rank-based statistics when the margins are continuous. As a by-product, under smoothness assumptions, we obtain that the asymptotic distribution of the estimation errors of our estimators are Gaussian. Finally, numerical experiments are presented to assess the finite sample performance of the estimators, and the usefulness of the proposed methodologies is illustrated with a copula-based regression model for hydrological data.
更多查看译文
关键词
semiparametric models,arbitrary marginal distributions,identifiability,inference,copula-based
AI 理解论文
溯源树
样例
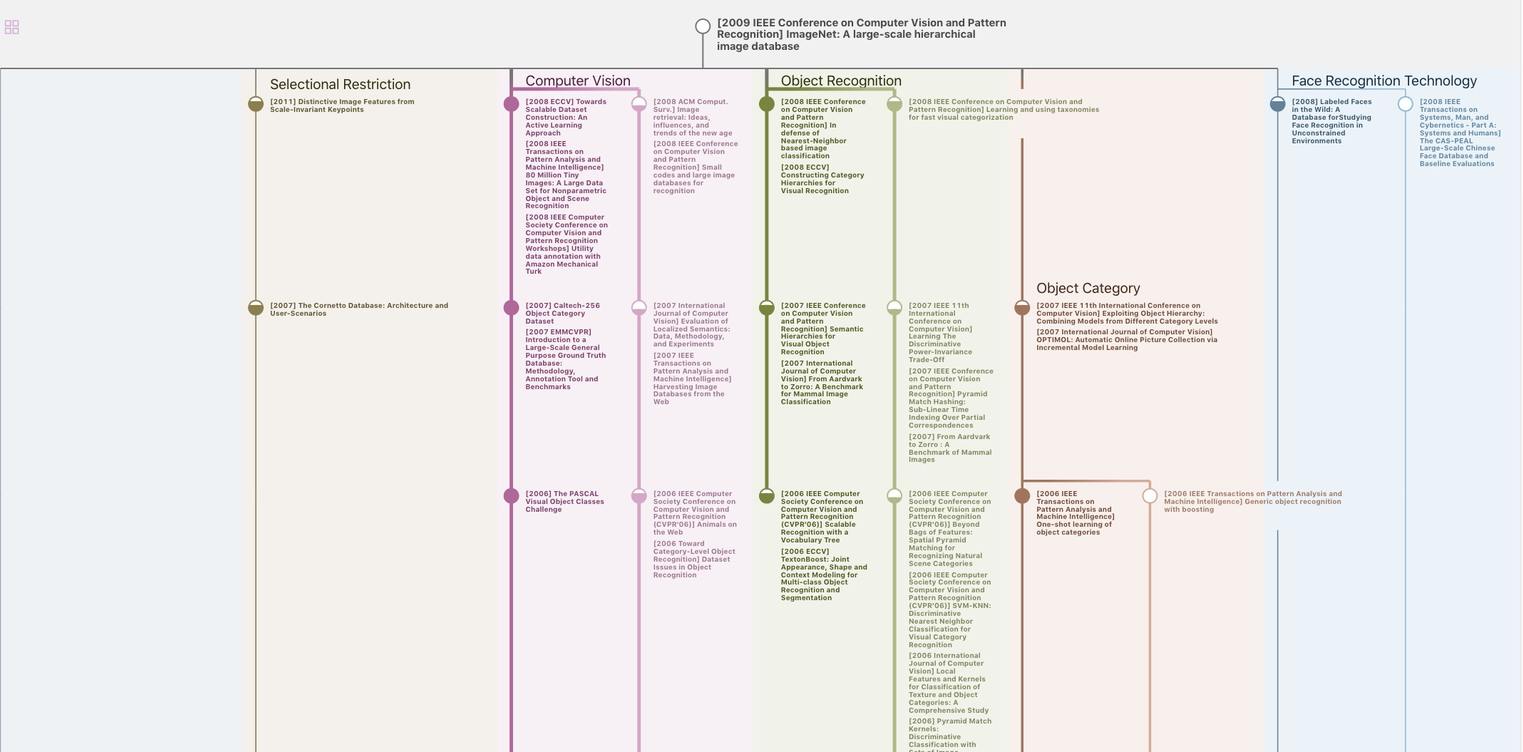
生成溯源树,研究论文发展脉络
Chat Paper
正在生成论文摘要