Robust empirical risk minimization via Newton’s method
arxiv(2023)
摘要
A new variant of Newton’s method for empirical risk minimization is studied, where at each iteration of the optimization algorithm, the gradient and Hessian of the objective function are replaced by robust estimators taken from existing literature on robust mean estimation for multivariate data. After proving a general theorem about the convergence of successive iterates to a small ball around the population-level minimizer, consequences of the theory in generalized linear models are studied when data are generated from Huber’s epsilon-contamination model and/or heavy-tailed distributions. An algorithm for obtaining robust Newton directions based on the conjugate gradient method is also proposed, which may be more appropriate for high-dimensional settings, and conjectures about the convergence of the resulting algorithm are offered. Compared to robust gradient descent, the proposed algorithm enjoys the faster rates of convergence for successive iterates often achieved by second-order algorithms for convex problems, i.e., quadratic convergence in a neighborhood of the optimum, with a stepsize that may be chosen adaptively via backtracking linesearch.
更多查看译文
关键词
robust empirical risk minimization,empirical risk minimization,newton
AI 理解论文
溯源树
样例
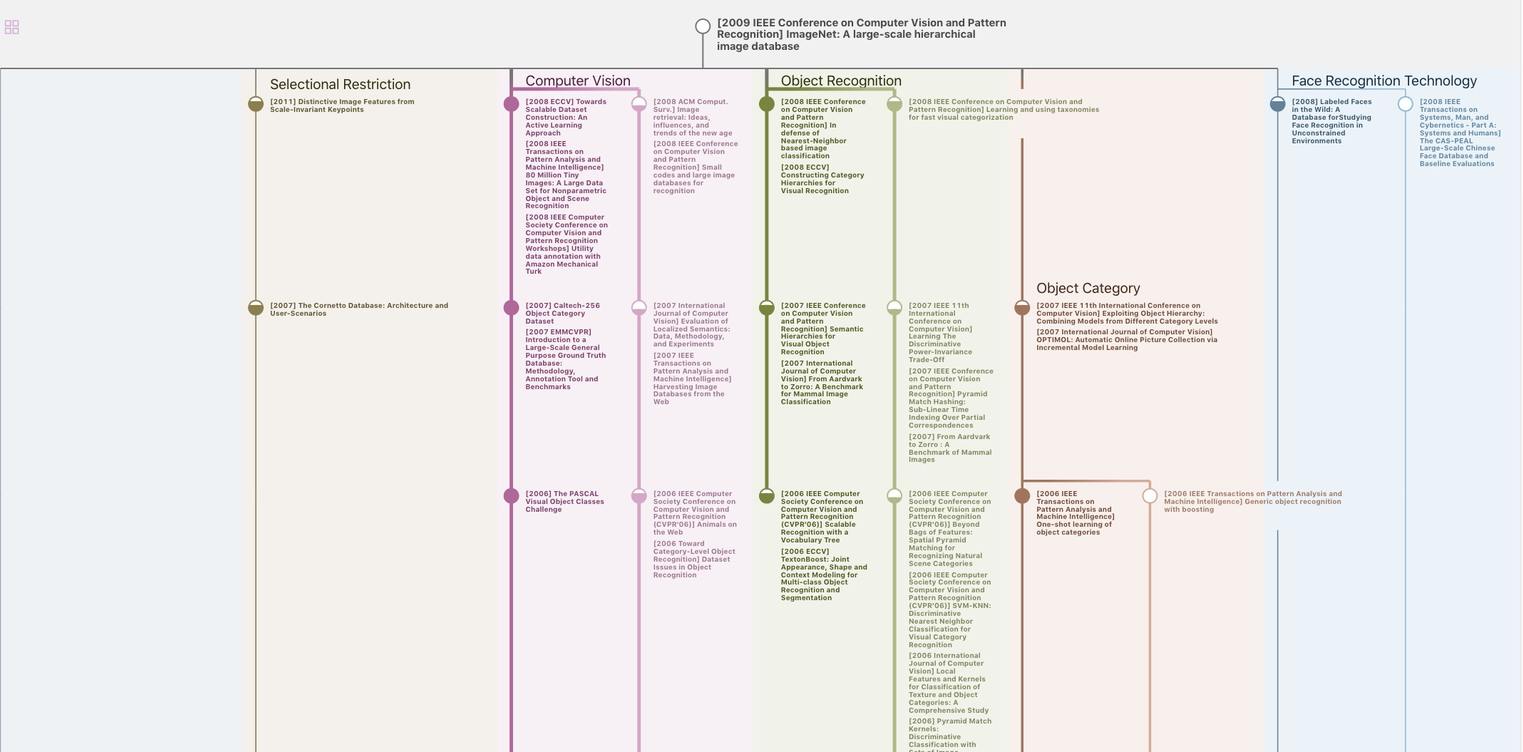
生成溯源树,研究论文发展脉络
Chat Paper
正在生成论文摘要