Federated Learning for Water Consumption Forecasting in Smart Cities
ICC 2023 - IEEE INTERNATIONAL CONFERENCE ON COMMUNICATIONS(2023)
摘要
Water consumption remains a major concern among the world's future challenges. For applications like load monitoring and demand response, deep learning models are trained using enormous volumes of consumption data in smart cities. On the one hand, the information used is private. For instance, the precise information gathered by a smart meter that is a part of the system's IoT architecture at a consumer's residence may give details about the appliances and, consequently, the consumer's behavior at home. On the other hand, enormous data volumes with sufficient variation are needed for the deep learning models to be trained properly. This paper introduces a novel model for water consumption prediction in smart cities while preserving privacy regarding monthly consumption. The proposed approach leverages federated learning (FL) as a machine learning paradigm designed to train a machine learning model in a distributed manner while avoiding sharing the users data with a central training facility. In addition, this approach is promising to reduce the overhead utilization through decreasing the frequency of data transmission between the users and the central entity. Extensive simulation illustrate that the proposed approach shows an enhancement in predicting water consumption for different households.
更多查看译文
关键词
Federated learning,edge learning,water consumption prediction
AI 理解论文
溯源树
样例
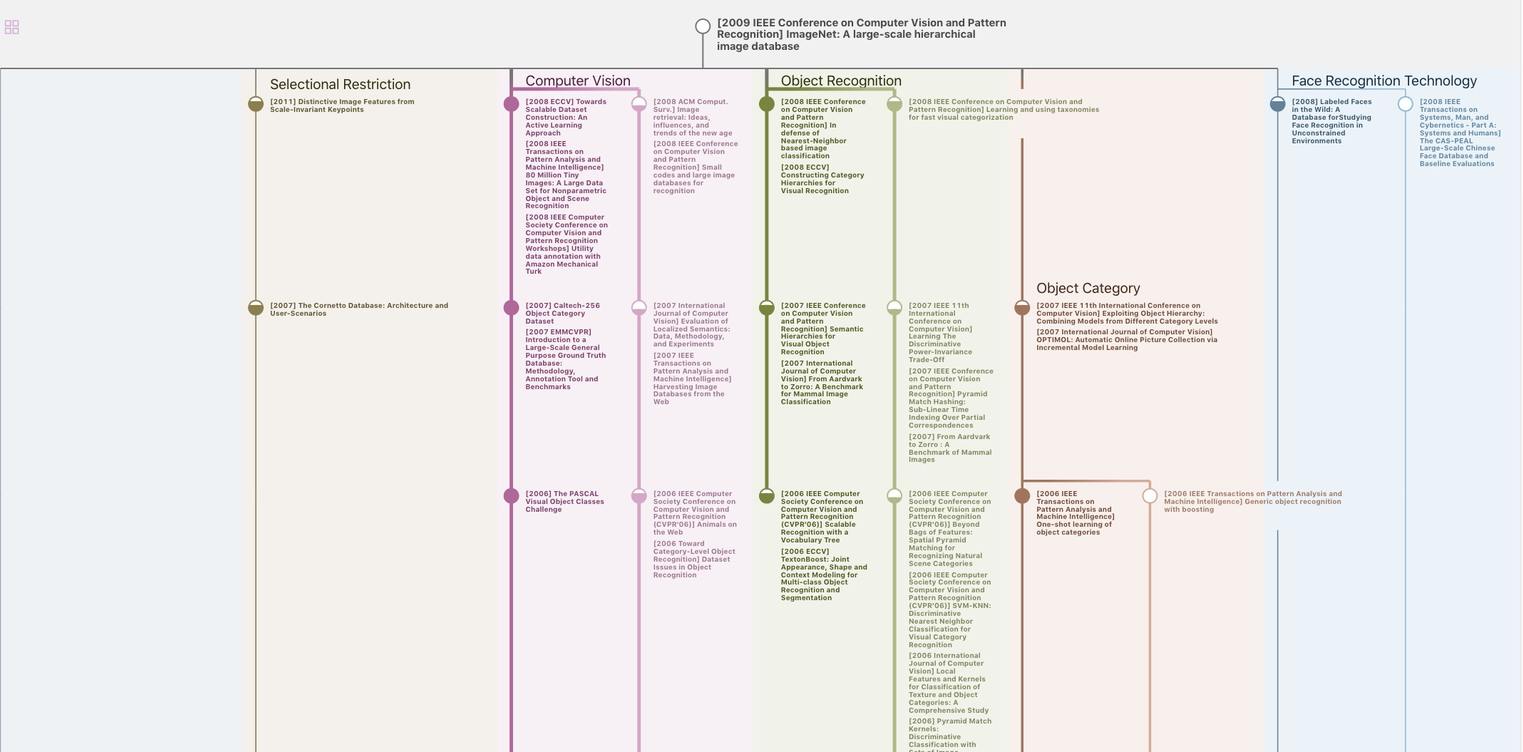
生成溯源树,研究论文发展脉络
Chat Paper
正在生成论文摘要