Hierarchical Imitation Learning with Vector Quantized Models
arxiv(2023)
摘要
The ability to plan actions on multiple levels of abstraction enables intelligent agents to solve complex tasks effectively. However, learning the models for both low and high-level planning from demonstrations has proven challenging, especially with higher-dimensional inputs. To address this issue, we propose to use reinforcement learning to identify subgoals in expert trajectories by associating the magnitude of the rewards with the predictability of low-level actions given the state and the chosen subgoal. We build a vector-quantized generative model for the identified subgoals to perform subgoal-level planning. In experiments, the algorithm excels at solving complex, long-horizon decision-making problems outperforming state-of-the-art. Because of its ability to plan, our algorithm can find better trajectories than the ones in the training set
更多查看译文
关键词
hierarchical imitation learning,models
AI 理解论文
溯源树
样例
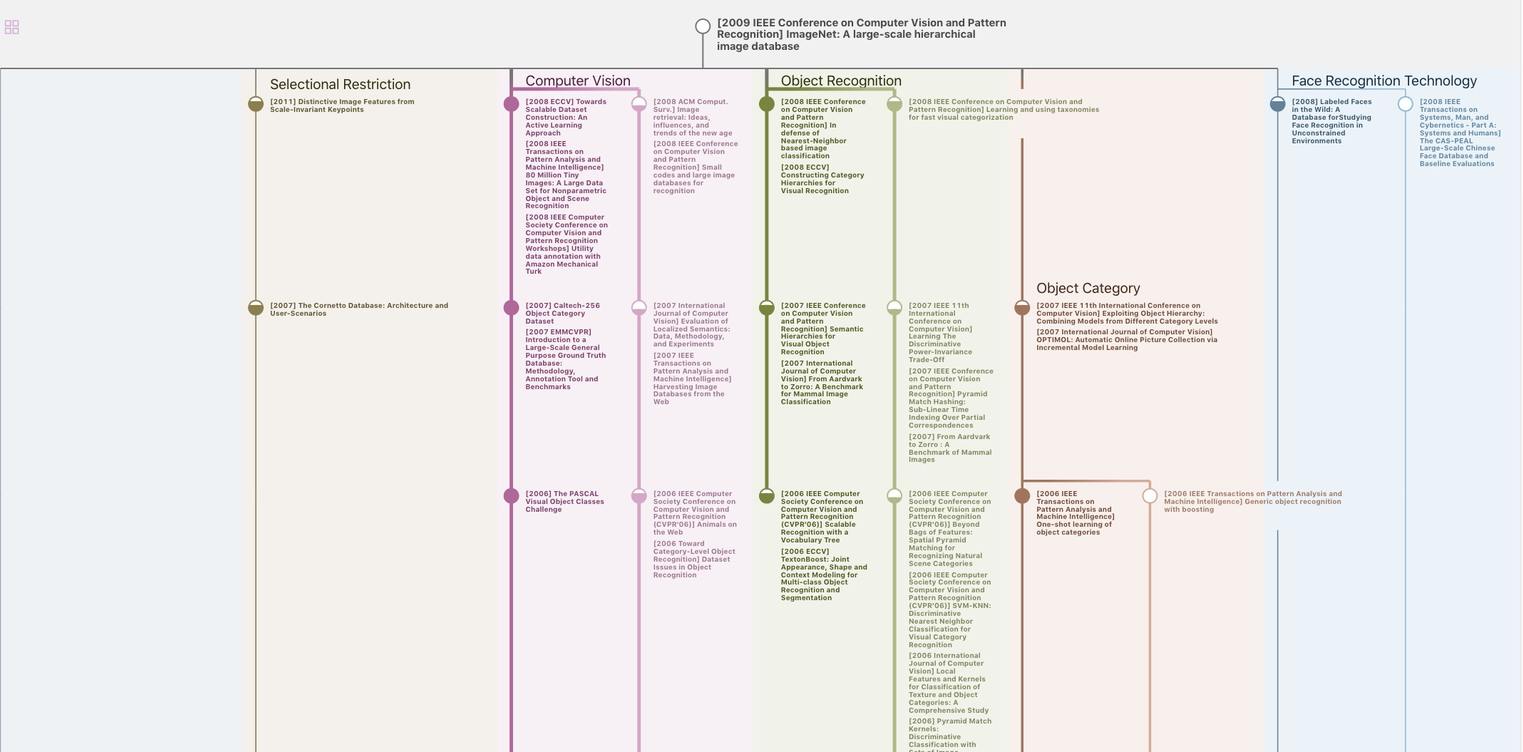
生成溯源树,研究论文发展脉络
Chat Paper
正在生成论文摘要