How Far Can It Go?: On Intrinsic Gender Bias Mitigation for Text Classification
arxiv(2023)
摘要
To mitigate gender bias in contextualized language models, different intrinsic mitigation strategies have been proposed, alongside many bias metrics. Considering that the end use of these language models is for downstream tasks like text classification, it is important to understand how these intrinsic bias mitigation strategies actually translate to fairness in downstream tasks and the extent of this. In this work, we design a probe to investigate the effects that some of the major intrinsic gender bias mitigation strategies have on downstream text classification tasks. We discover that instead of resolving gender bias, intrinsic mitigation techniques and metrics are able to hide it in such a way that significant gender information is retained in the embeddings. Furthermore, we show that each mitigation technique is able to hide the bias from some of the intrinsic bias measures but not all, and each intrinsic bias measure can be fooled by some mitigation techniques, but not all. We confirm experimentally, that none of the intrinsic mitigation techniques used without any other fairness intervention is able to consistently impact extrinsic bias. We recommend that intrinsic bias mitigation techniques should be combined with other fairness interventions for downstream tasks.
更多查看译文
关键词
intrinsic gender bias mitigation,classification
AI 理解论文
溯源树
样例
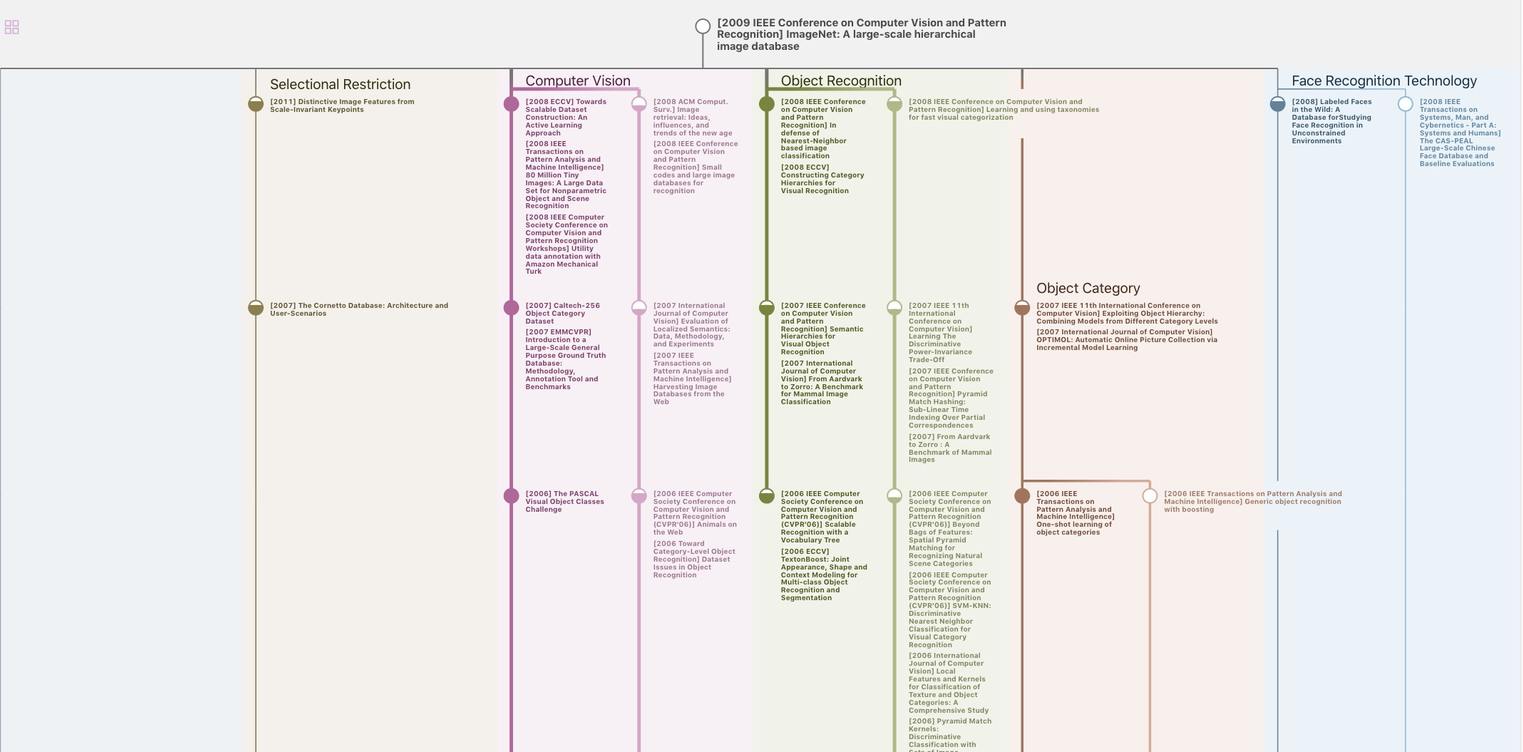
生成溯源树,研究论文发展脉络
Chat Paper
正在生成论文摘要