Asymptotic Inference for Multi-Stage Stationary Treatment Policy with High Dimensional Features
arxiv(2023)
摘要
Dynamic treatment rules or policies are a sequence of decision functions over multiple stages that are tailored to individual features. One important class of treatment policies for practice, namely multi-stage stationary treatment policies, prescribe treatment assignment probabilities using the same decision function over stages, where the decision is based on the same set of features consisting of both baseline variables (e.g., demographics) and time-evolving variables (e.g., routinely collected disease biomarkers). Although there has been extensive literature to construct valid inference for the value function associated with the dynamic treatment policies, little work has been done for the policies themselves, especially in the presence of high dimensional feature variables. We aim to fill in the gap in this work. Specifically, we first estimate the multistage stationary treatment policy based on an augmented inverse probability weighted estimator for the value function to increase the asymptotic efficiency, and further apply a penalty to select important feature variables. We then construct one-step improvement of the policy parameter estimators. Theoretically, we show that the improved estimators are asymptotically normal, even if nuisance parameters are estimated at a slow convergence rate and the dimension of the feature variables increases exponentially with the sample size. Our numerical studies demonstrate that the proposed method has satisfactory performance in small samples, and that the performance can be improved with a choice of the augmentation term that approximates the rewards or minimizes the variance of the value function.
更多查看译文
关键词
inference,multi-stage
AI 理解论文
溯源树
样例
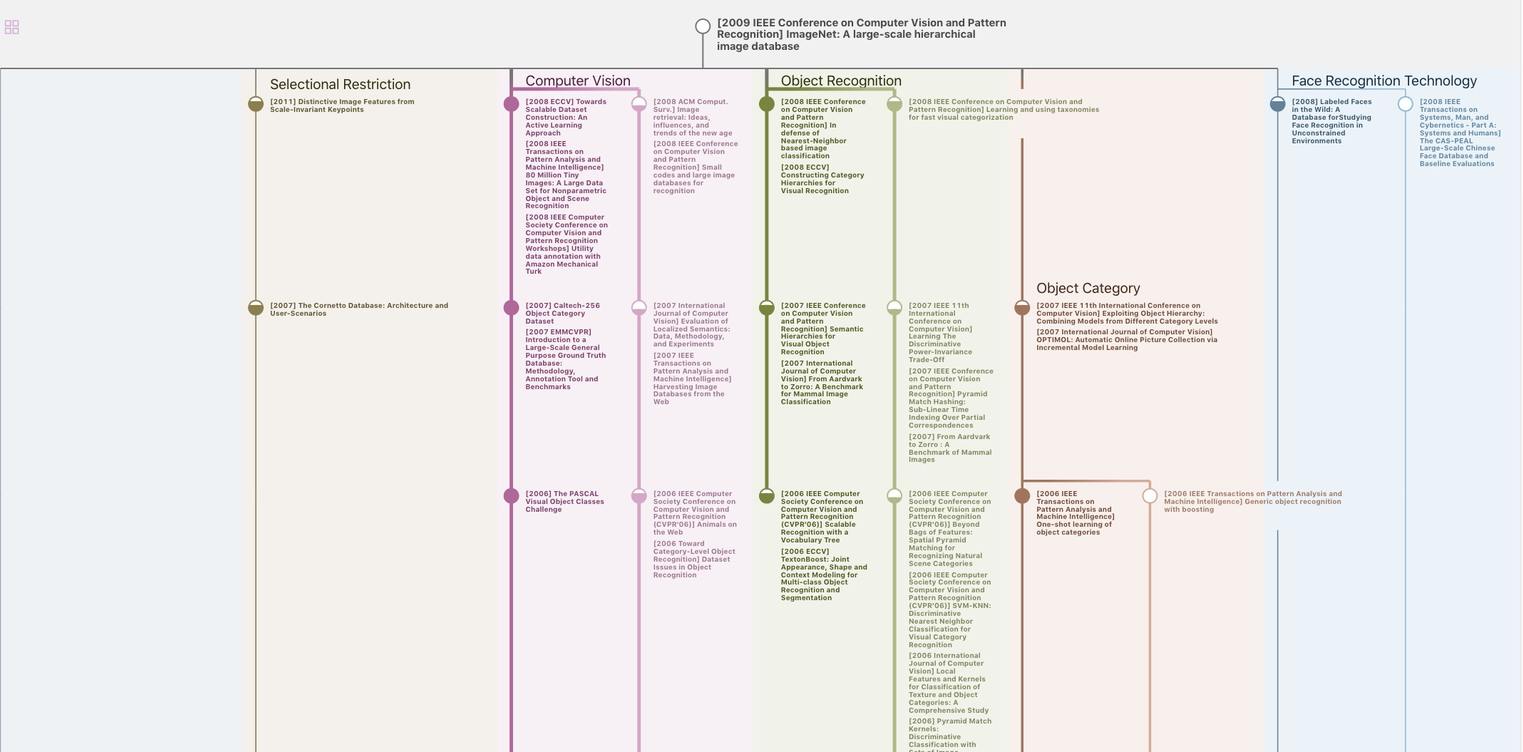
生成溯源树,研究论文发展脉络
Chat Paper
正在生成论文摘要