Composing Task Knowledge with Modular Successor Feature Approximators
ICLR 2023(2023)
摘要
Recently, the Successor Features and Generalized Policy Improvement (SF&GPI) framework has been proposed as a method for learning, composing, and transferring predictive knowledge and behavior. SF&GPI works by having an agent learn predictive representations (SFs) that can be combined for transfer to new tasks with GPI. However, to be effective this approach requires state features that are useful to predict, and these state-features are typically hand-designed. In this work, we present a novel neural network architecture, "Modular Successor Feature Approximators" (MSFA), where modules both discover what is useful to predict, and learn their own predictive representations. We show that MSFA is able to better generalize compared to baseline architectures for learning SFs and modular architectures
更多查看译文
关键词
deep reinforcement learning,successor features,generalization,compositional generalization
AI 理解论文
溯源树
样例
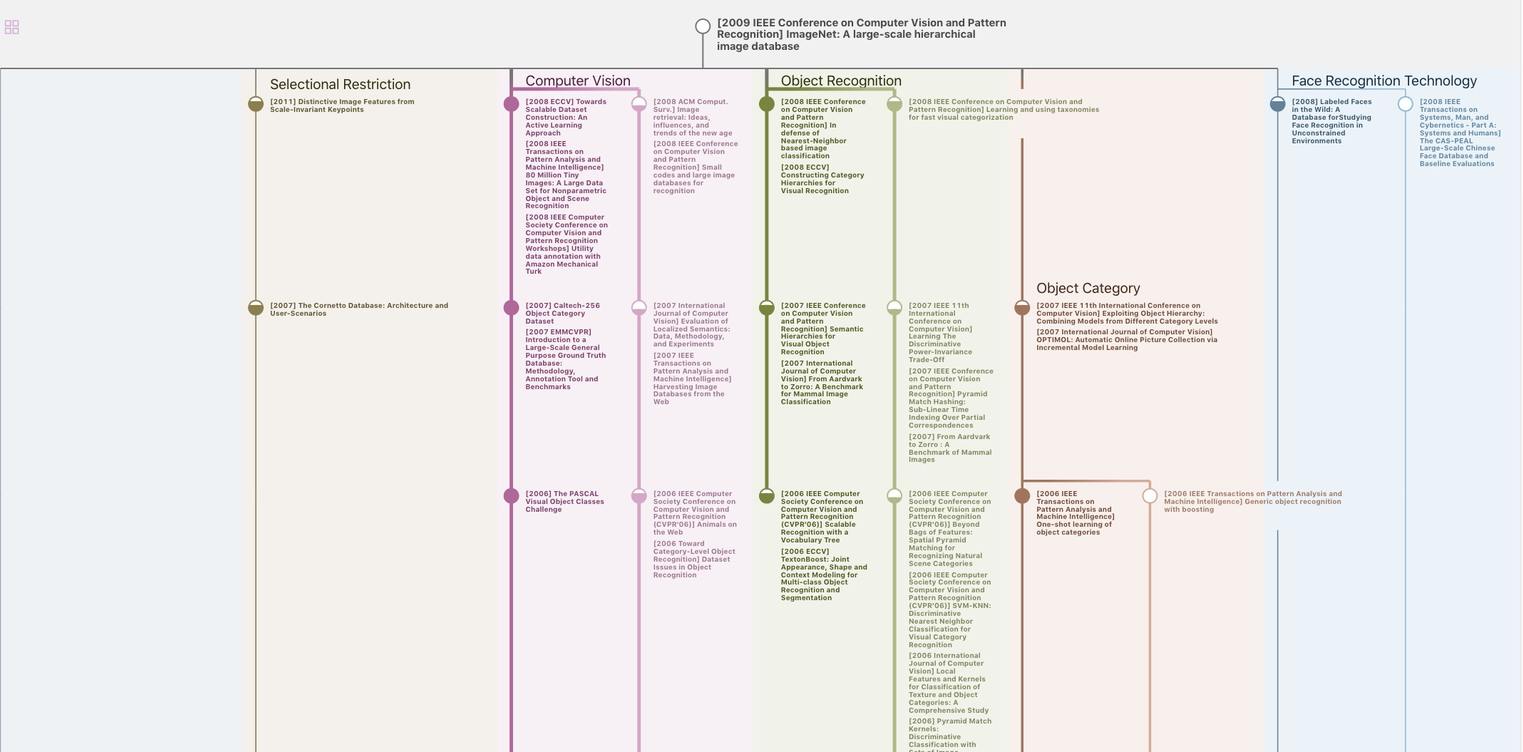
生成溯源树,研究论文发展脉络
Chat Paper
正在生成论文摘要