A Decomposable Causal View of Compositional Zero-Shot Learning
IEEE TRANSACTIONS ON MULTIMEDIA(2023)
摘要
Composing and recognizing novel concepts that are combinations of known concepts, i.e., compositional generalization, is one of the greatest power of human intelligence. With the development of artificial intelligence, it becomes increasingly appealing to build a vision system that can generalize to unknown compositions based on restricted known knowledge, which has so far remained a great challenge to our community. In fact, machines can be easily misled by superficial correlations in the data, disregarding the causal patterns that are crucial to generalization. In this paper, we rethink compositional generalization with a causal perspective, upon the context of Compositional Zero-Shot Learning (CZSL). We develop a simple yet strong approach based on our novel Decomposable Causal view (dubbed "DECA"), by approximating the causal effect with the combination of three easy-to-learn components. Our proposed DECA(1) is evaluated on two challenging CZSL benchmarks by recognizing unknown compositions of known concepts. Despite being simple in the design, our approach achieves consistent improvements over state-of-the-art baselines, demonstrating its superiority towards the goal of compositional generalization.
更多查看译文
关键词
Compositional zero-shot learning,vision and language,image recognition,causality
AI 理解论文
溯源树
样例
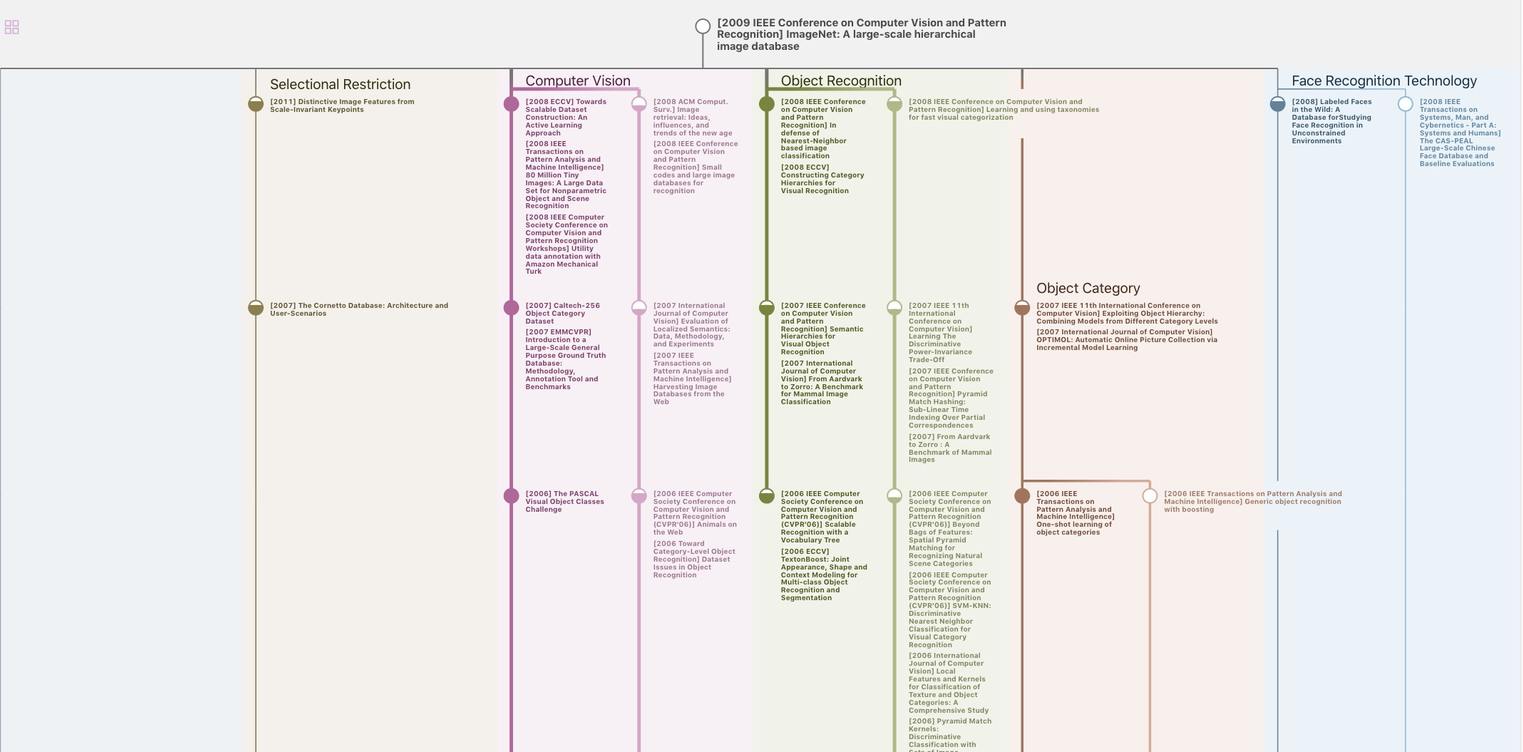
生成溯源树,研究论文发展脉络
Chat Paper
正在生成论文摘要