Benefits of Multiobjective Learning in Solar Energy Prediction
arxiv(2023)
摘要
While the space of renewable energy forecasting has received significant attention in the last decade, literature has primarily focused on machine learning models that train on only one objective at a time. A host of classification (and regression) tasks in energy markets lead to highly imbalanced training data. Say, to balance reserves, it is natural for market regulators to have a choice to be more/less averse to false negatives (can lead to poor operating efficiency and costs) than to false positives (can lead to market shortfall). Besides accuracy, other metrics like algorithmic bias, RMBE (in regression problems), inferencing time, and model sparsity are also very crucial. This paper is amongst the firsts in the field of renewable energy forecasting that attempts to present a Pareto frontier of solutions (tradeoffs), that answers the question on handling multiple objectives by means of using the XGBoost model (Gradient Boosted Trees). Our proposed algorithm relies on using a sequence of weighted (uniform meshes) single objective model training routines. Real world data examples from the Amherst (Massachusetts, United States) solar energy prediction panels with both triobjective (focus on accuracy) and biojective (focus on fairness/bias) classification instances are considered. Numerical experiments appear promising and clear advantages over single objective methods are seen by observing the spread and variety of solutions (model configurations).
更多查看译文
关键词
multiobjective learning,energy,prediction
AI 理解论文
溯源树
样例
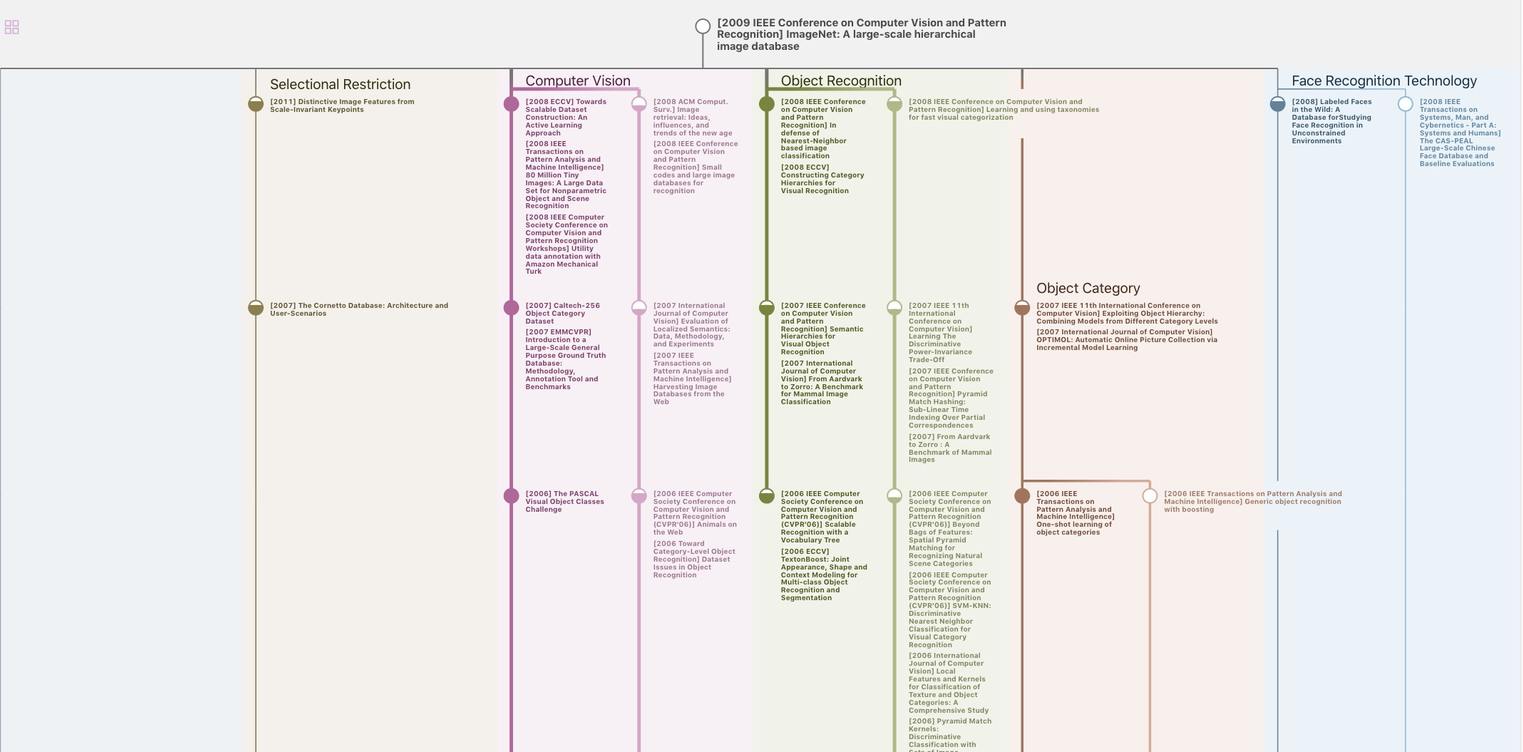
生成溯源树,研究论文发展脉络
Chat Paper
正在生成论文摘要