Informational Diversity and Affinity Bias in Team Growth Dynamics
PROCEEDINGS OF 2023 ACM CONFERENCE ON EQUITY AND ACCESS IN ALGORITHMS, MECHANISMS, AND OPTIMIZATION, EAAMO 2023(2023)
摘要
Prior work has provided strong evidence that, within organizational settings, teams that bring a diversity of information and perspectives to a task are more effective than teams that do not. If this form of informational diversity confers performance advantages, why do we often see largely homogeneous teams in practice? One canonical argument is that the benefits of informational diversity are in tension with affinity bias. To better understand the impact of this tension on the makeup of teams, we analyze a sequential model of team formation in which individuals care about their team's performance (captured in terms of accurately predicting some future outcome based on a set of features) but experience a cost as a result of interacting with teammates who use different approaches to the prediction task. Our analysis of this simple model reveals a set of subtle behaviors that team-growth dynamics can exhibit: (i) from certain initial team compositions, they can make progress toward better performance but then get stuck partway to optimally diverse teams; while (ii) from other initial compositions, they can also move away from this optimal balance as the majority group tries to crowd out the opinions of the minority. The initial composition of the team can determine whether the dynamics will move toward or away from performance optimality, painting a path-dependent picture of inefficiencies in team compositions. Our results formalize a fundamental limitation of utility-based motivations to drive informational diversity in organizations and hint at interventions that may improve informational diversity and performance simultaneously.
更多查看译文
AI 理解论文
溯源树
样例
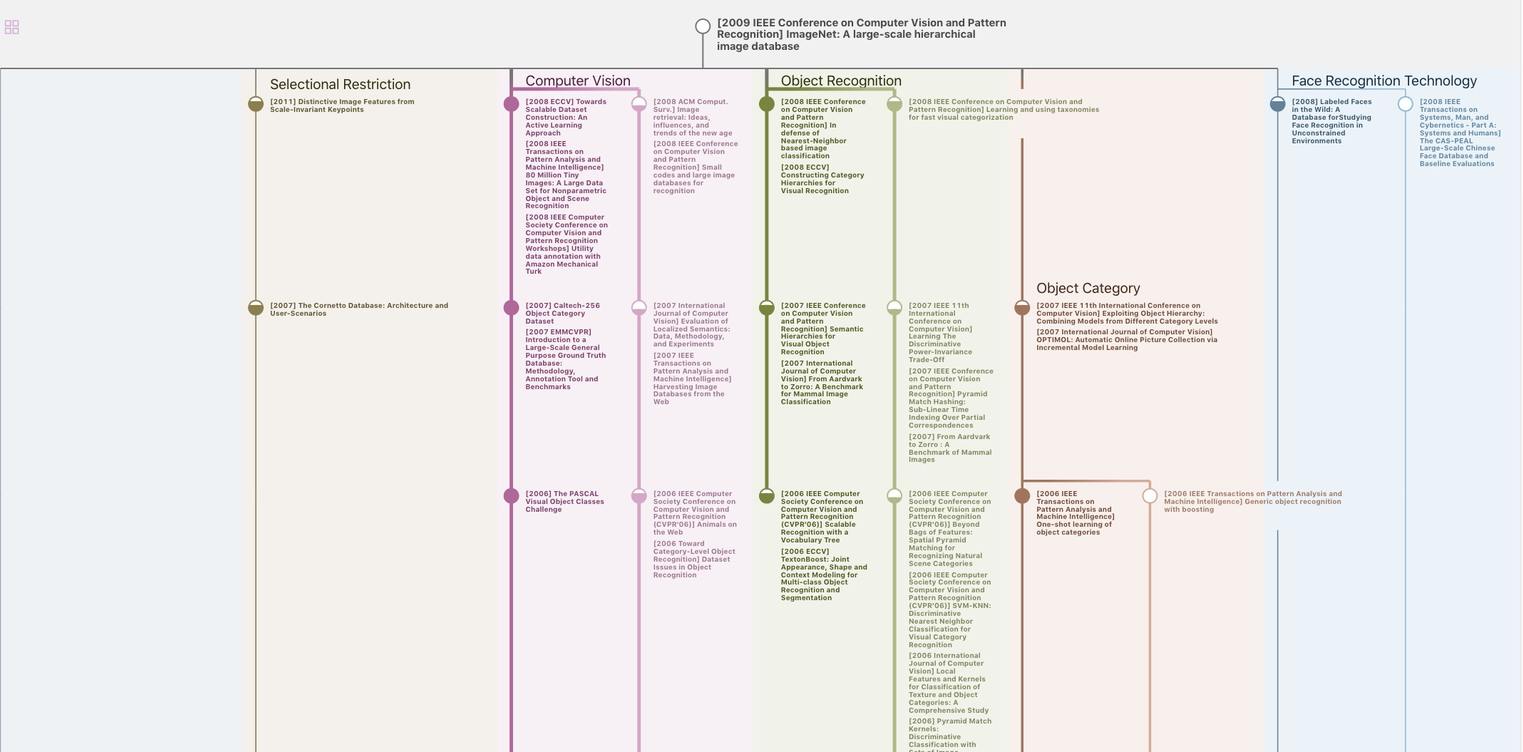
生成溯源树,研究论文发展脉络
Chat Paper
正在生成论文摘要