Object-Preserving Siamese Network for Single-Object Tracking on Point Clouds
IEEE TRANSACTIONS ON MULTIMEDIA(2024)
摘要
Undoubtedly, the object is the primary factor in 3D single-object tracking (SOT) tasks. However, prior Siamese-based trackers overlook the adverse effects resulting from randomly dropped object points during backbone sampling, hindering the prediction of accurate bounding boxes (BBoxes). Therefore, developing an approach that maximizes the preservation of object points and their object-aware features is of the utmost significance. To address this, we propose an object-preserving Siamese network (OPSNet) that can effectively maintain object integrity and boost tracking performance. First, an object highlighting module amplifies the object-aware features and extracts discriminative features from the template and search area. Next, object-preserving sampling selects object candidates, obtains object-preserving search area seeds, and discards background points that have less impact on tracking. Finally, an object localization network accurately locates 3D BBoxes based on the object-preserving search area seeds. Extensive experiments demonstrate that the performance of OPSNet exceeds the state-of-the-art performance, achieving success gains of similar to 9.4% and similar to 2.5% on the KITTI and Waymo Open datasets, respectively.
更多查看译文
关键词
Three-dimensional displays,Search problems,Feature extraction,Point cloud compression,Location awareness,Task analysis,Deep learning,Object tracking,Siamese network,point clouds,autonomous driving,deep learning
AI 理解论文
溯源树
样例
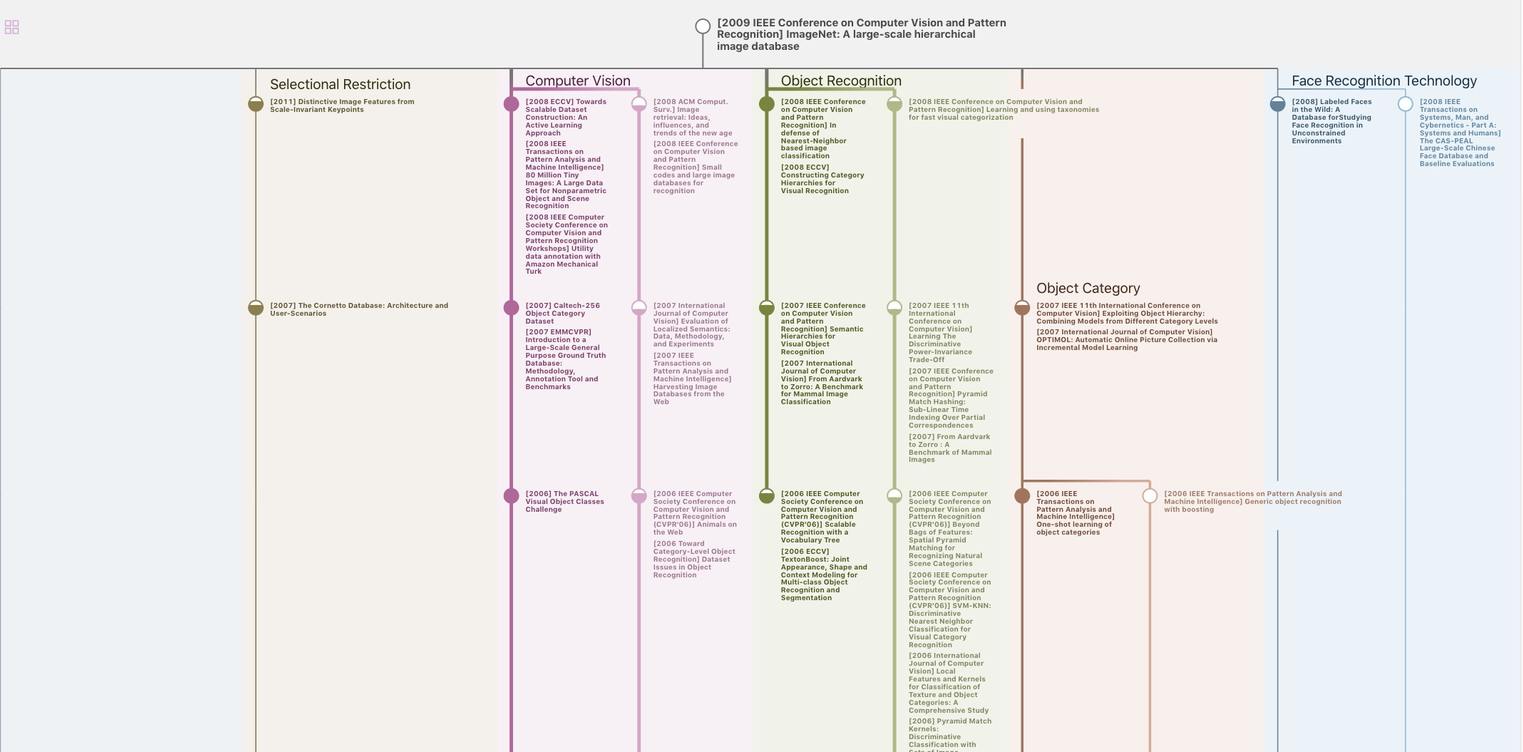
生成溯源树,研究论文发展脉络
Chat Paper
正在生成论文摘要