On-line fault diagnosis of rotating machinery based on deep residual network
2022 37th Youth Academic Annual Conference of Chinese Association of Automation (YAC)(2022)
摘要
Rotating machinery is an indispensable basic component in industrial applications. Because the working environment of rotating machinery often has severe conditions such as heavy loads, failures will inevitably occur. In the fault diagnosis of rotating machinery, the original time series signals often needs to be extracted by the time-frequency analysis method, which is very dependent on prior knowledge and manual experience. The deep neural network is an end-to-end fitting algorithm with self-learning capability, which can automatically find highdimensional features from the incoming signals or images, thereby avoiding the complex feature extraction process in traditional algorithms that requires a lot of experience. This research constructed a deep residual network model and proposed an online fault diagnosis method for rotating machinery. Using the data processing method of converting the multi-axis vibration time-series signal into a multi-channel sample matrix, 100% accuracy is obtained in the fault diagnosis tasks of the Case Western Reserve University bearing fault data and the pump fault data experimental platform. Compared with traditional CNN, the convergence speed and accuracy are improved. At the same time, compared with traditional fault diagnosis methods, this method reduces the feature extraction process that requires experience and has good generalization and applicability.
更多查看译文
关键词
Rotating machinery,Fault diagnosis,Deep learning,Deep residual network
AI 理解论文
溯源树
样例
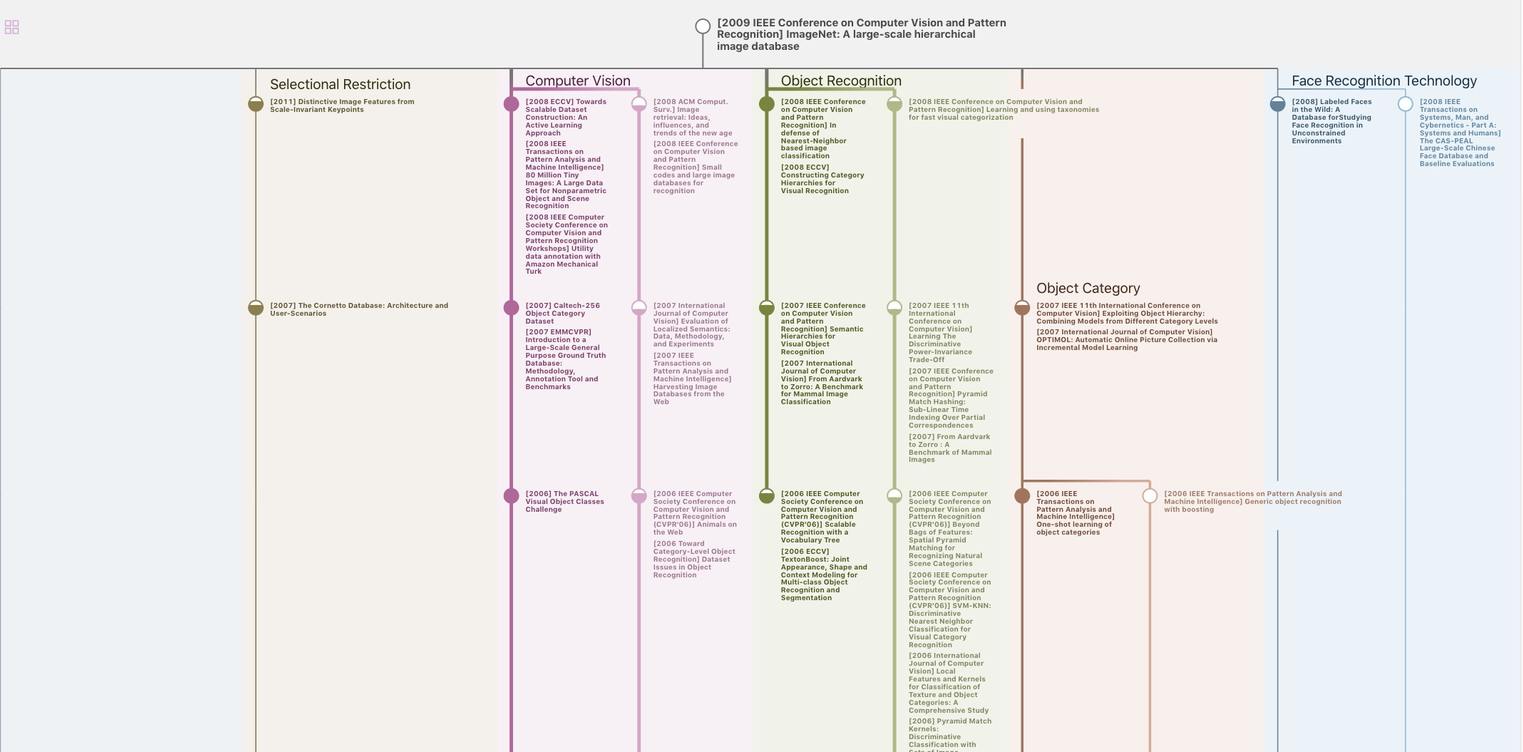
生成溯源树,研究论文发展脉络
Chat Paper
正在生成论文摘要