Self-supervised learning of spatiotemporal thermal signatures in additive manufacturing using reduced order physics models and transformers
COMPUTATIONAL MATERIALS SCIENCE(2024)
摘要
Microstructure control via additive manufacturing has enormous potential as manufacturers, materials scien-tists, and designers alike seek to exploit novel fabrication technologies to improve component performance. Recent works have demonstrated the feasibility of producing materials with controlled microstructures across various length scales. However, the experimental approach towards exploring the process-structure space can be laborious and costly. This is particularly true if also considering scan pattern optimization which is well suited for processes such as powder bed fusion electron beam melting. In this work we propose an approach for encoding additive manufacturing layer-wise thermal response signatures using self-supervised representation learning. Thermal simulations from a reduced order model are utilized to estimate the spatiotemporal response during printing. A machine learning framework, using video-transformers, is utilized to efficiently distill spatiotemporal patterns into a compact latent space representation. This latent state representation encodes the relevant physics which is then utilized to establish a data-driven process-structure model for an additively manufactured Ni-based superalloy. The proposed methodology could potentially be used towards in-situ process monitoring, scan pattern experimental design, and component qualification.
更多查看译文
关键词
Additive manufacturing,Microstructure control,Machine learning,Data-driven modeling
AI 理解论文
溯源树
样例
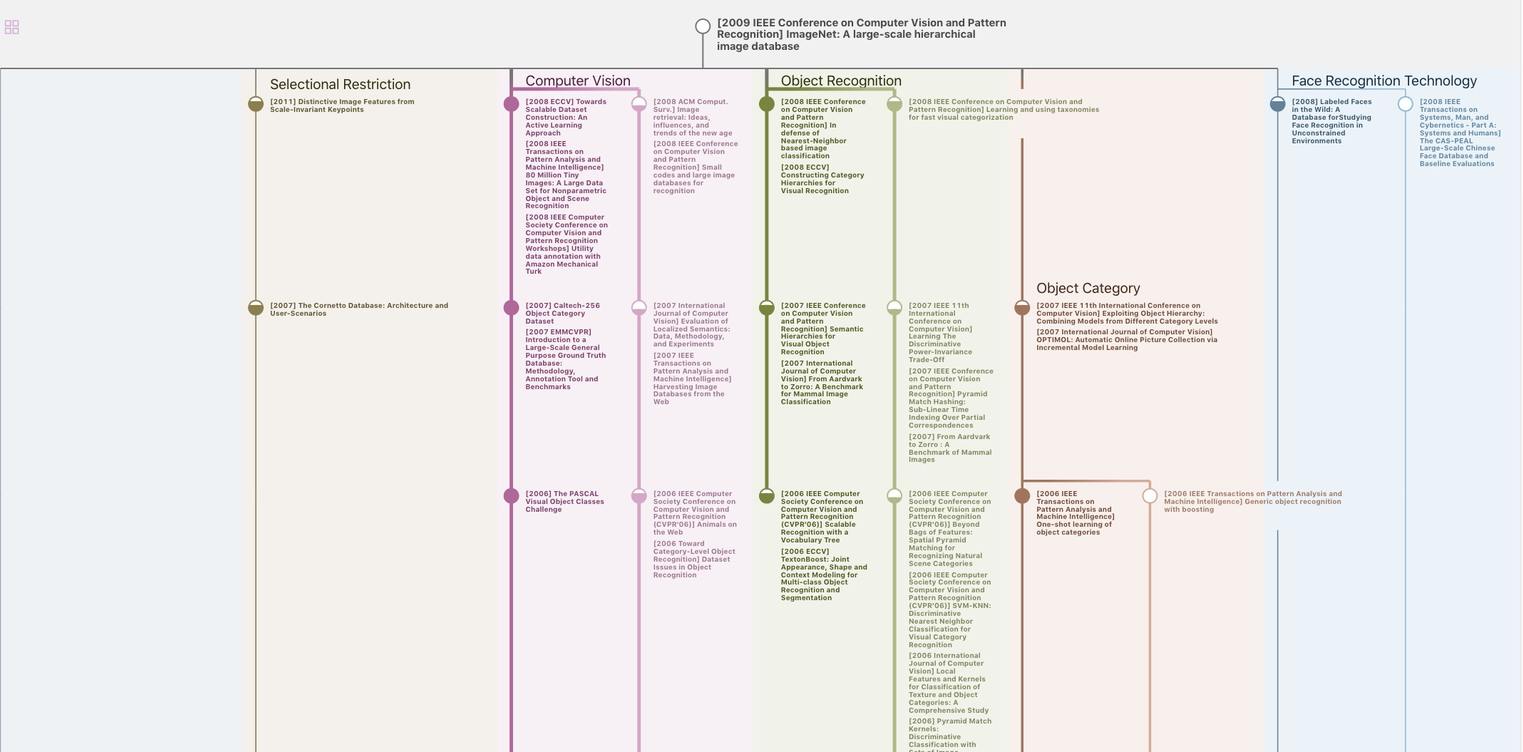
生成溯源树,研究论文发展脉络
Chat Paper
正在生成论文摘要