Deep Learning-Based Autonomous Cow Detection for Smart Livestock Farming.
GPC(2022)
摘要
Animal sourced protein is increasing rapidly due to the growing of population and incomes. The big data, robots and smart sensing technologies have brought the autonomous robotic system to the smart farming that enhance productivity and efficiency. Therefore, a YOLOv4-SAM was proposed to achieve high detection precision of cow body parts in long-term complex scenes. The proposed YOLOv4-SAM consists of two components: YOLOv4 is for multi-scale feature extraction, while the Spatial AttentionMechanisms (SAM) highlights the key cow biometric-related features. By doing this, visual biometric feature representation ability is enhanced for improving cowdetection performance. To verify the performance ofYOLOv4-SAM, a challenging dataset consisting of adult cows and calves with complex environments (e.g., day and night, occlusion, multiple target) was constructed for experimental testing. The precision, recall, mIoU and of the proposed YOLOv4-CBAMwere 92.29%, 96.51%, 77.22% and 93.13%, respectively. The data shows that its overall performance was better than that of the comparison algorithm (Faster R-CNN, RetinaNet and YOLOv4). In addition, object detection based on the YOLOv4-SAM model can capture the key biometric-related features for cow visual representation and improve the performance of cow detection. In addition, the detected height difference between head and leg proved the capability in automatic identification of lame cows. The proposed deep learning-based cow detection approach provides a basis for developing an automated system for animal monitoring and management on commercial dairy farms.
更多查看译文
关键词
autonomous cow detection,learning-based
AI 理解论文
溯源树
样例
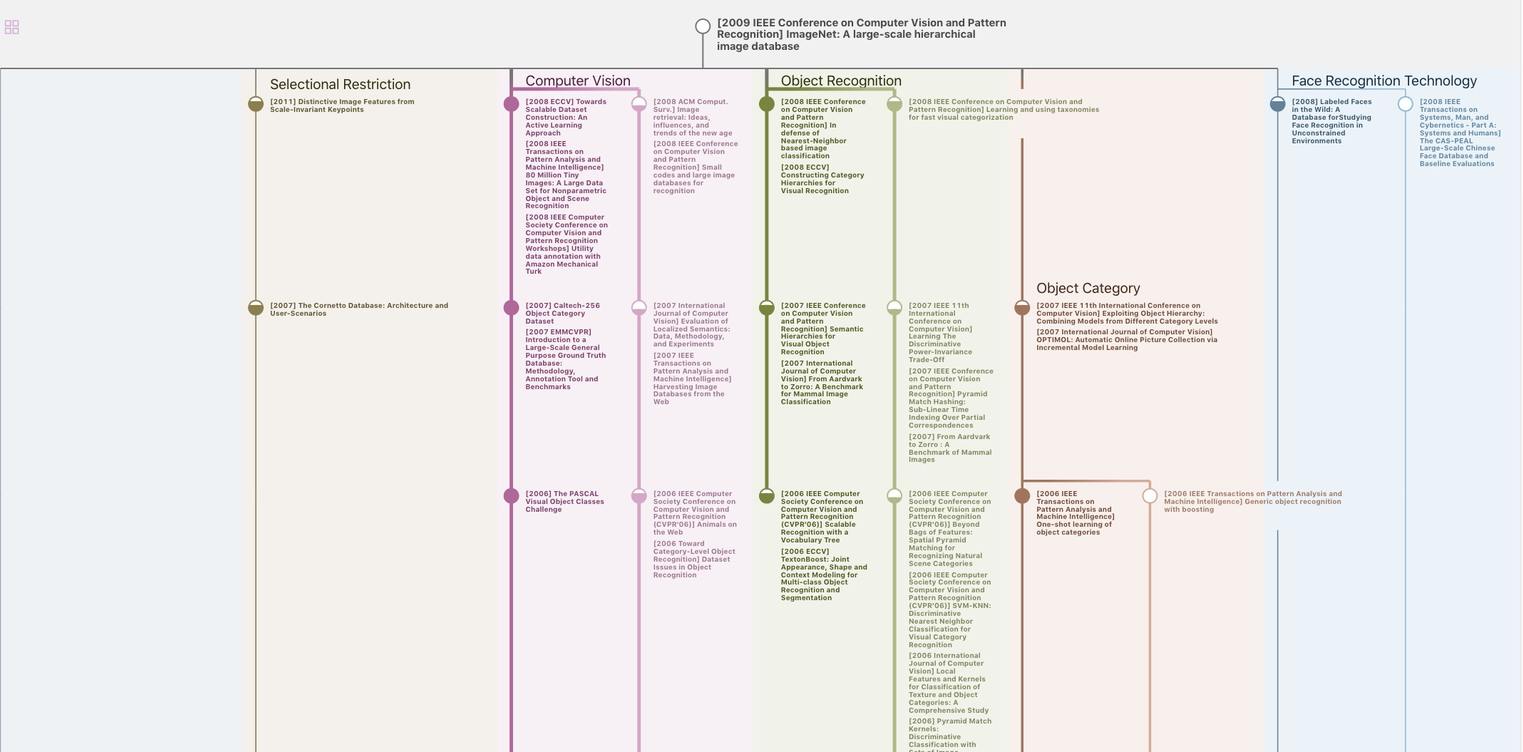
生成溯源树,研究论文发展脉络
Chat Paper
正在生成论文摘要