Drivers’ drowsiness detection based on an optimized random forest classification and single-channel electroencephalogram
International Journal of Electrical and Computer Engineering (IJECE)(2023)
The state of functioning (posture) of a driver at the wheel of a car involves a complex set of psychological, physiological, and physical parameters. This combination induces fatigue, which manifests itself in repeated yawning, stinging eyes, a frozen gaze, a stiff and painful neck, back pain, and other signs. The driver may fight fatigue for a few moments, but it inevitably leads to drowsiness, periods of micro-sleep, and then falling asleep. At the first signs of drowsiness, the risk of an accident becomes immense. In Morocco, drowsiness at the wheel is the cause of 1/3 of fatal accidents on the freeways. Thus, in this paper, a new hybrid data analysis and an efficient machine learning algorithm are designed to detect the drowsiness of drivers who spend most of their time behind the wheel over long distances (older than 35 years). This analysis is based on a single channel of electroencephalogram (EEG) recordings using time, frequency fast Fourier transform (FFT), and power spectral density (PSD) analysis. To distinguish between the two states of alertness and drowsiness, several features were extracted from each domain (time, FFT, and PSD), and subjected to different classifier architectures to conduct a general comparison and achieve the highest detection accuracy (98.5%) and best time consumption (13 milliseconds).
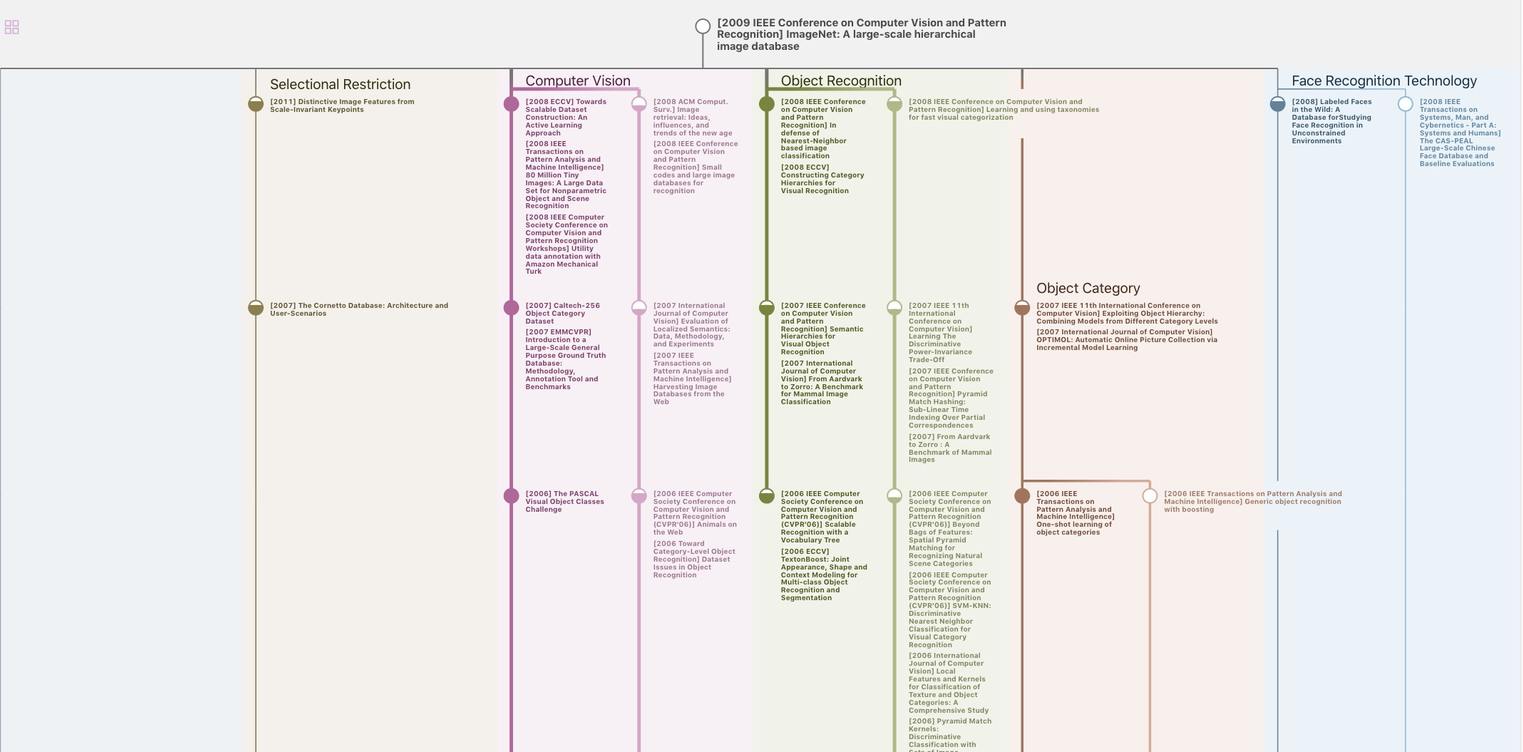