A data‐driven soft sensor based on weighted probabilistic slow feature analysis for nonlinear dynamic chemical processes
Journal of Chemometrics(2023)
摘要
Modeling high-dimensional dynamic processes is a challenging task. In this regard, probabilistic slow feature analysis (PSFA) is revealed to be advantageous for dynamic soft sensor modeling, which can extract slowly varying intrinsic features from high-dimensional data. However, nonlinearities prevalent in industrial processes are not considered, which could lead to unsatisfactory prediction performance. In this paper, a weighted PSFA (WPSFA)-based soft sensor model is proposed for nonlinear dynamic chemical process. In WPSFA, a weighted log-likelihood function of complete data is constructed to linearize the nonlinear state emission equation. Then, the expectation maximization algorithm is applied to estimate the model parameters and a locally weighted regression model is established for quality prediction. Finally, the feasibility and effectiveness of the proposed approach are well illustrated through a numerical example and a real industrial process.
更多查看译文
关键词
expectation maximization algorithm,nonlinear dynamic processes,soft sensor,weighted probabilistic slow feature analysis
AI 理解论文
溯源树
样例
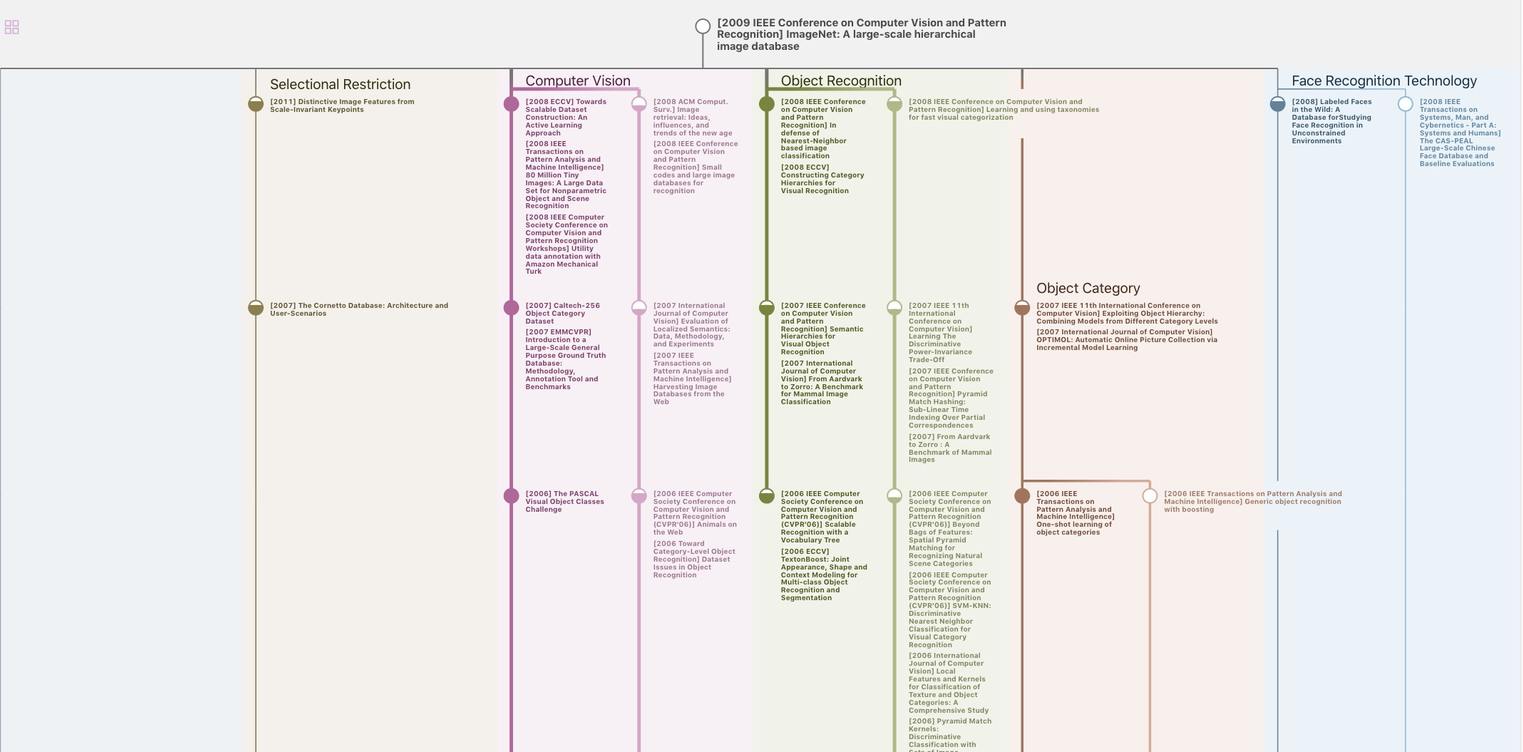
生成溯源树,研究论文发展脉络
Chat Paper
正在生成论文摘要