CoMix: Collaborative Filtering with Mixup for Implicit Datasets
Information Sciences(2023)
摘要
Collaborative filtering (CF) is the prevalent solution to mitigate massive information overload in modern recommender systems. However, it usually suffers from data sparsity and popularity bias problems. Existing studies exploit auxiliary information or data augmentation, requiring additional data collection or expensive computational overheads. Inspired by Mixup used in the classification problem, we propose a simple-yet-effective data augmentation method for CF, namely Collaborative Mixup (CoMix), for implicit feedback datasets. The underlying idea of CoMix is to generate virtual users/items by logically combining random users/items. Unlike the original Mixup, we synthesize virtual users/items to complement weak collaborative signals by distinguishing the intersection and non-overlapping parts between two users/items. Despite its simplicity, extensive experimental results show that various CF models equipped with CoMix consistently improve the base models on four benchmark datasets.
更多查看译文
关键词
collaborative filtering,implicit,mixup
AI 理解论文
溯源树
样例
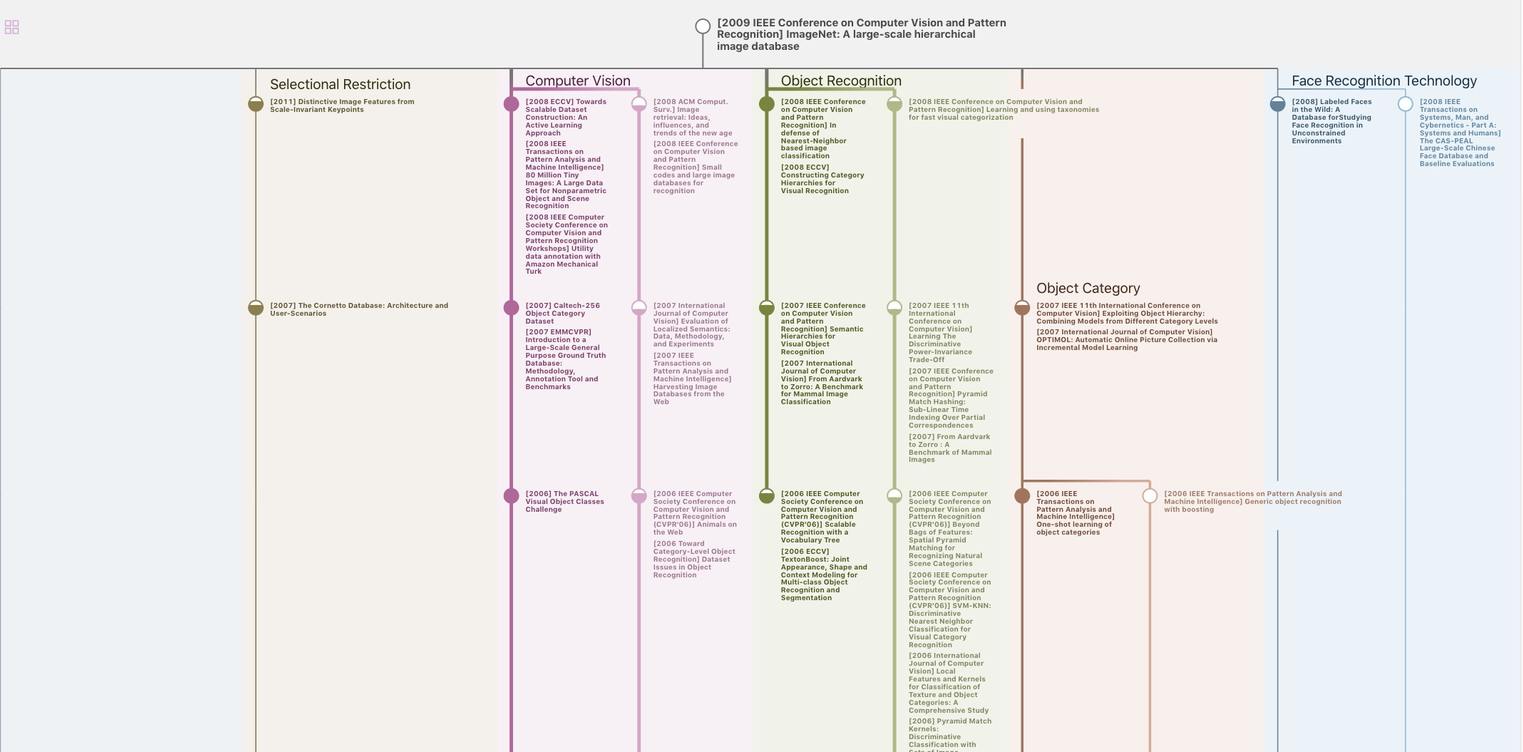
生成溯源树,研究论文发展脉络
Chat Paper
正在生成论文摘要