Combining ICESat-2 photons and Google Earth Satellite images for building height extraction
International Journal of Applied Earth Observations and Geoinformation(2023)
摘要
Building heights are one of the crucial data for comprehending the functions of urban systems. Employing optical remote sensing imagery, the shadow-based method is one of the most promising methods which have been proposed for estimating building height. However, the existing shadow-based studies for building height estimation are restricted to a small area due to the lack of building height annotations and ignorance of building azimuth variations. The Ice, Cloud, and Land Elevation Satellite-2 (ICESat-2) allows large-scale building height retrieval in the along-track direction and thus can be taken as ground truth building height annotations to support the shadow-based algorithms for large-scale building height extraction. Here, we proposed an approach for extracting building height by combining Google Earth Satellite (GES) images and ICESat-2 photons. Building and shadow instances were first extracted using a U-Net deep learning framework. Based on the building height annotations retrieved from ICESat-2 photons, an improved shadow-based building height estimation model by minimizing the global error across all sample buildings was developed. A typical urban area located in the city center of Shanghai, China with an area of around 90 km2 was selected to validate the proposed method. In total 15,966 buildings were successfully extracted and the results indicated that the estimated building heights have high accuracy with an absolute mean error of 4.08 m. Moreover, the proposed method shows a better performance compared to the existing shadow-based method and existing building height datasets. The method holds great potential for large-scale building-level height retrieval which contributes to further studies of urban morphologies.
更多查看译文
关键词
Building height,ICESat-2,Google Earth images,Building shadow,U-Net
AI 理解论文
溯源树
样例
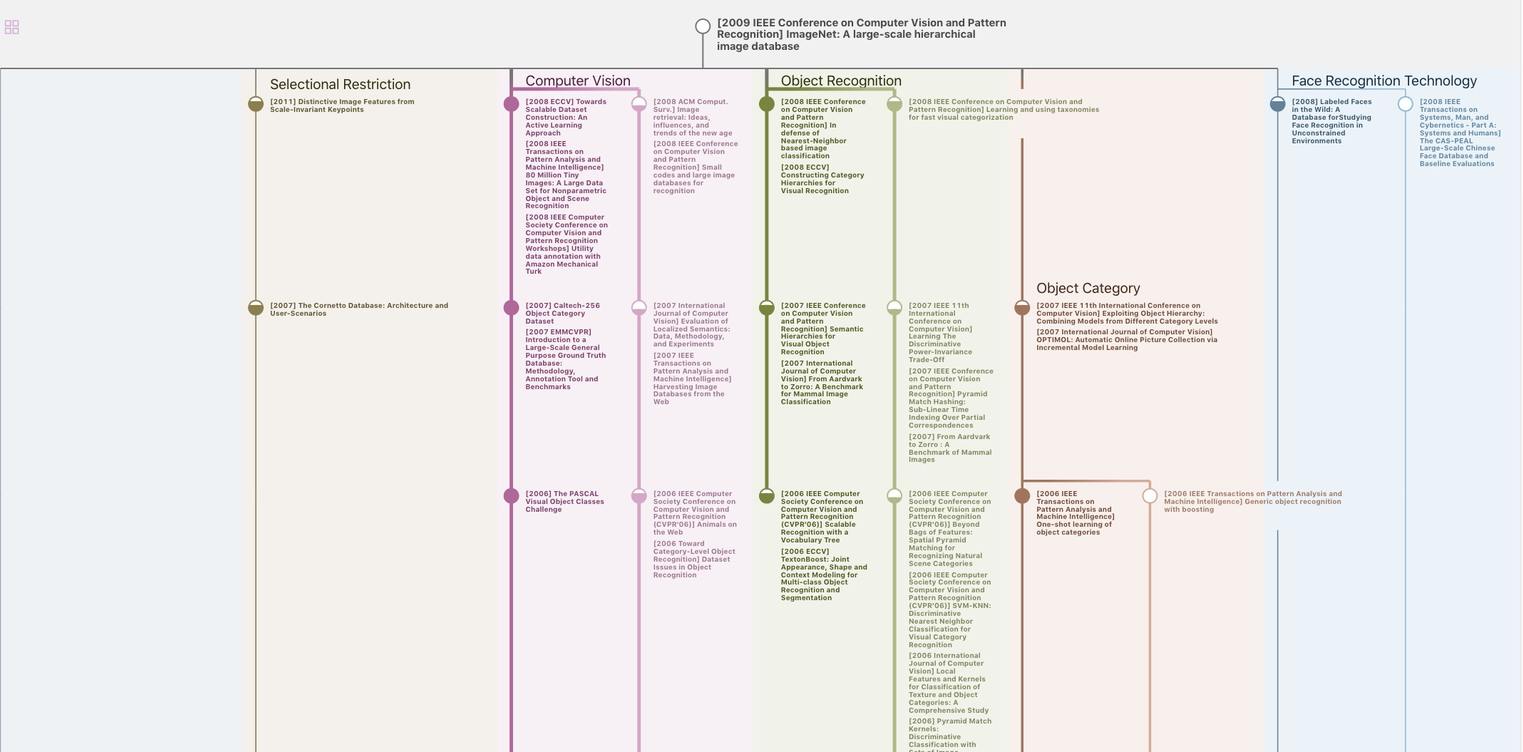
生成溯源树,研究论文发展脉络
Chat Paper
正在生成论文摘要