Role of Strong Localized vs. Weak Distributed Interactions in Disordered Protein Phase Separation
The Journal of Physical Chemistry B(2023)
摘要
Interaction strength and localization are critical parameters controlling the single-chain and condensed-state properties of intrinsically disordered proteins (IDPs). Here, we decipher these relationships using coarse-grained heteropolymers comprised of hydrophobic (H) and polar (P) monomers as model IDPs. We systematically vary the fraction of P monomers X P and employ two distinct particle-based models that include either strong localized attractions between only H–H pairs (HP model) or weak distributed attractions between both H–H and H–P pairs (HP+ model). To compare different sequences and models, we first carefully tune the attraction strength for all sequences to match the single-chain radius of gyration. Interestingly, we find that this procedure produces similar conformational ensembles, nonbonded potential energies, and chain-level dynamics for single chains of almost all sequences in both models, with some deviations for the HP model at large X P. However, we observe a surprisingly rich phase behavior for the sequences in both models that deviates from the expectation that similarity at the single-chain level will translate to similar phase-separation propensity. Coexistence between dilute and dense phases is only observed up to a model-dependent X P despite the presence of favorable interchain interactions, which we quantify using the second virial coefficient. Instead, the limited number of attractive sites (H monomers) leads to the self-assembly of finite-sized clusters of different sizes depending on X P. Our findings strongly suggest that models with distributed interactions favor the formation of liquid-like condensates over a much larger range of sequence compositions compared to models with localized interactions.
### Competing Interest Statement
The authors have declared no competing interest.
更多查看译文
关键词
disordered protein phase separation,strong localized
AI 理解论文
溯源树
样例
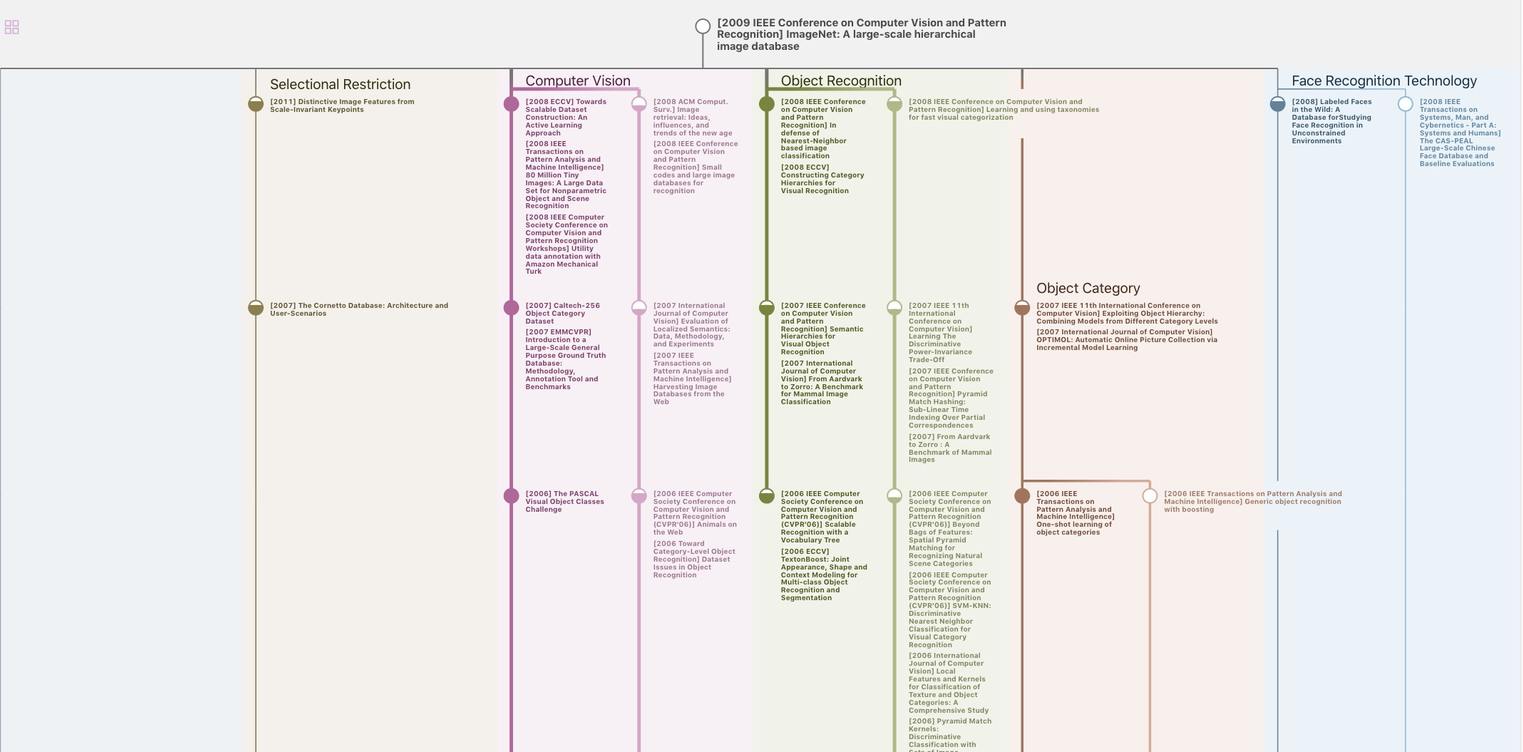
生成溯源树,研究论文发展脉络
Chat Paper
正在生成论文摘要