Semantic-Aware Feature Aggregation for Few-Shot Image Classification
Neural Processing Letters(2023)
摘要
Generating features from the most relevant image regions has shown great potential in solving the challenging few-shot image classification problem. Most of existing methods aggregate image regions weighted with attention maps to obtain category-specific features. Instead of using attention maps to indicate the relevance of image regions, we directly model the interdependencies between prototype features and image regions, resulting in a novel Semantic-Aware Feature Aggregation (SAFA) framework that can place more weights on category-relevant image regions. Specifically, we first design a “reduce and expand” block to extract category-relevant prototype features for each image. Then, we introduce an additive attention mechanism to highlight category-relevant image regions while suppressing the others. Finally, the weighted image regions are aggregated and used for classification. Extensive experiments show that our SAFA places more weights on category-relevant image regions and achieves state-of-the-art performance.
更多查看译文
关键词
Few-shot image classification,Metric learning,Semantic-aware feature aggregation,Neural network
AI 理解论文
溯源树
样例
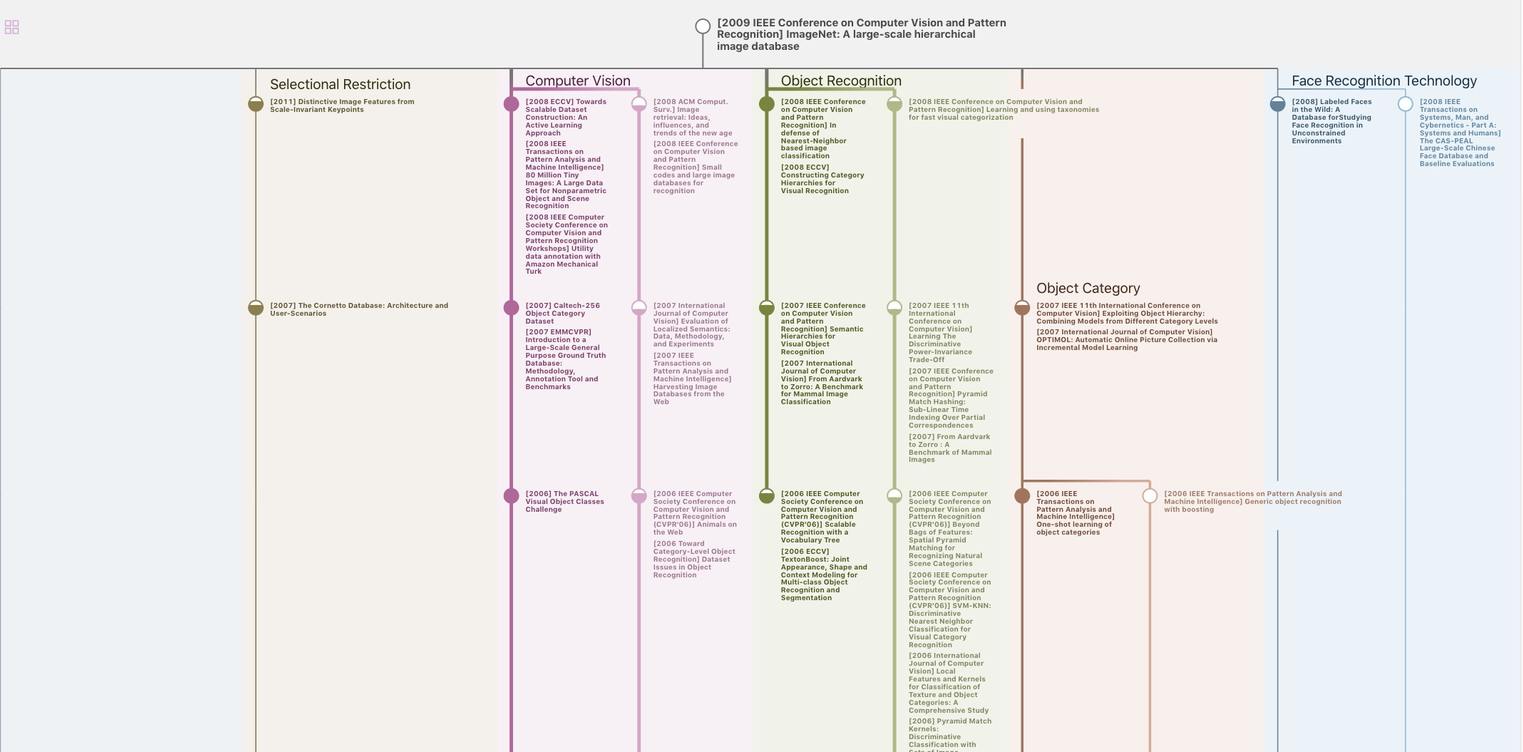
生成溯源树,研究论文发展脉络
Chat Paper
正在生成论文摘要