Structure-Aware Weakly Supervised Network for Building Extraction From Remote Sensing Images.
IEEE Trans. Geosci. Remote. Sens.(2022)
摘要
The use of fully supervised deep learning methods to extract buildings from remote sensing images (RSIs) has shown excellent performance, which requires large amounts of training data with laborious per-pixel labeling. Compared with pixelated intensive labeling, it is much easier to label data using scribbles, which only takes few seconds for one image. In this article, a one-stage structure-aware weakly supervised network (SAWSN) for building extraction is proposed, and it learns from easily accessible scribbles rather than from densely annotated ground truth. First, to solve the problem that direct training with scribble labels will lead to poor building structures, an auxiliary edge detection task is introduced to localize building edges explicitly. Second, a structure-aware scribble extension module (SASEM) is designed to recover building structures from scribbles through effective utilization of edge features. Finally, an edge-structure-aware loss is proposed to limit the scope of the restored structure. We perform extensive experiments on three newly labeled benchmark building extraction datasets (WHU, ISPRS Potsdam, and Vaihingen). Experimental results show that our method achieved 91.72%, 92.83%, and 92.22% of F1 using the ISPRS Vaihingen, Potsdam, and WHU datasets, respectively, and outperformed the state-of-the-art scribble-based weakly supervised (WS) methods by 3.27% of IoU.
更多查看译文
关键词
Buildings,Image edge detection,Feature extraction,Remote sensing,Data mining,Training,Deep learning,Building extraction,deep learning,remote sensing image (RSI),weakly supervised (WS) semantic segmentation
AI 理解论文
溯源树
样例
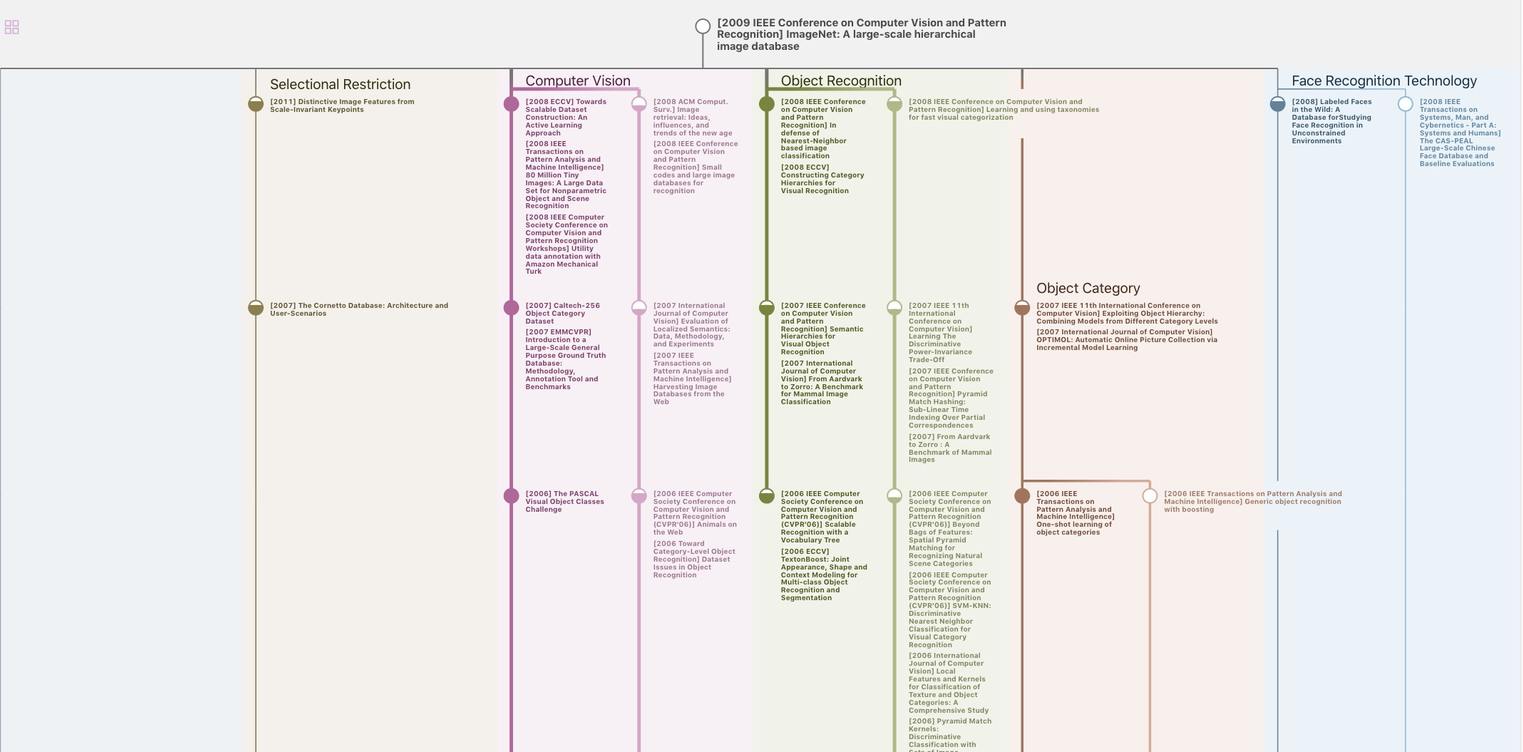
生成溯源树,研究论文发展脉络
Chat Paper
正在生成论文摘要