Towards Cross-Disaster Building Damage Assessment with Graph Convolutional Networks.
CoRR(2022)
摘要
In the aftermath of disasters, building damage maps are obtained using change detection to plan rescue operations. Current convolutional neural network approaches do not consider the similarities between neighboring buildings for predicting the damage. We present a novel graph-based building damage detection solution to capture these relationships. Our proposed model architecture learns from both local and neighborhood features to predict building damage. Specifically, we adopt the sample and aggregate graph convolution strategy to learn aggregation functions that generalize to unseen graphs which is essential for alleviating the time needed to obtain predictions for new disasters. Our experiments on the xBD dataset and comparisons with a classical convolutional neural network reveal that while our approach is handicapped by class imbalance, it presents a promising and distinct advantage when it comes to cross-disaster generalization.
更多查看译文
关键词
damage assessment,graph
AI 理解论文
溯源树
样例
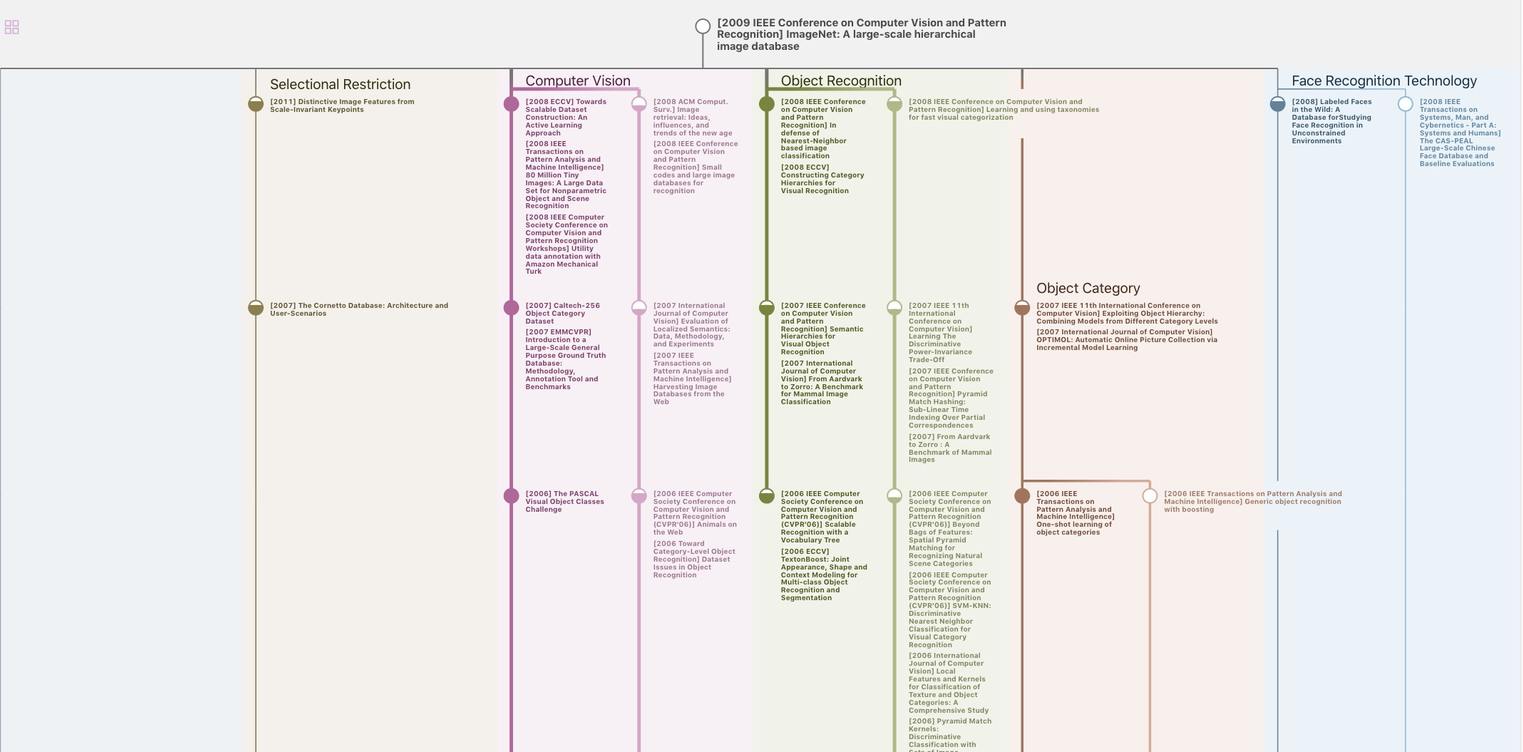
生成溯源树,研究论文发展脉络
Chat Paper
正在生成论文摘要