HWGN$$^{2}$$: Side-Channel Protected NNs Through Secure and Private Function Evaluation
SPACE(2022)
摘要
Recent work has highlighted the risks of intellectual property (IP) piracy of deep learning (DL) models from the side-channel leakage of DL hardware accelerators. In response, fundamental cryptographic approaches, specifically built upon the notion of secure and private function evaluation, could potentially improve the robustness against side-channel leakage. To examine this and weigh the costs and benefits, we introduce hardware garbled NN (HWGN 2), a DL hardware accelerator implemented on FPGA. HWGN2 also provides NN designers with the flexibility to protect their IP in real-time applications, where hardware resources are heavily constrained, through a hardware-communication cost trade-off. Concretely, we apply garbled circuits, implemented using a MIPS architecture that achieves up to 62.5x fewer logical and 66x less memory utilization than the state-of-the-art approaches at the price of communication overhead. Further, the side-channel resiliency of HWGN2 is demonstrated by employing the test vector leakage assessment (TVLA) test against both power and electromagnetic side-channels.
更多查看译文
关键词
private function evaluation,secure,side-channel
AI 理解论文
溯源树
样例
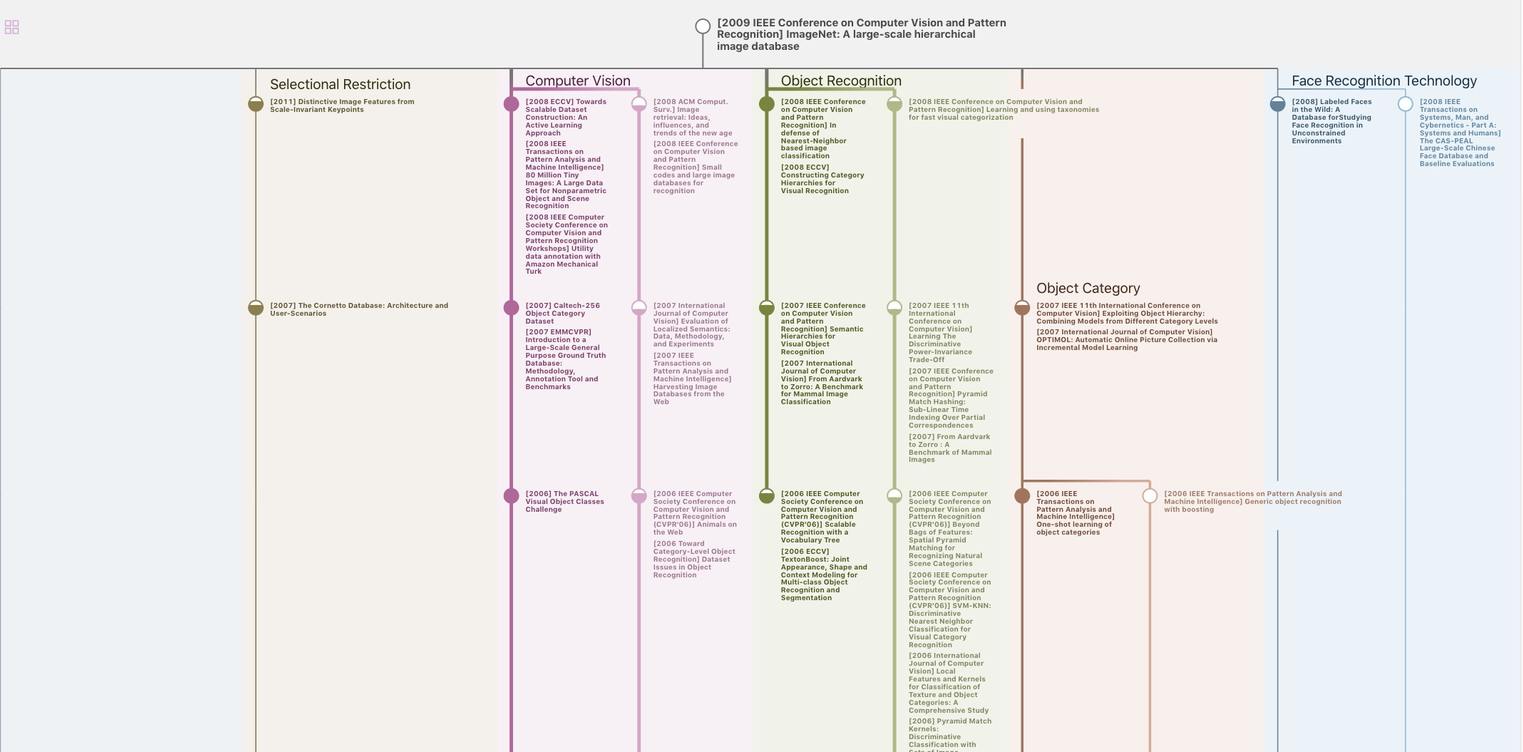
生成溯源树,研究论文发展脉络
Chat Paper
正在生成论文摘要