ABDPool: Attention-based Differentiable Pooling.
ICPR(2022)
摘要
Graph Neural Networks (GNNs) have achieved state-of-the-art performance on a wide range of graph-based tasks such as graph classification and node classification. This is because the unique structure of GNNs allows them to effectively learn embeddings for unstructured data. One important operation for graph classification tasks is downsampling or pooling, which obtains graph representations from node representations. However, most GNNs are associated with global pooling, that can not learn hierarchical graph representations. Meanwhile, current hierarchical pooling methods have the shortcomings of unclear node assignment and uniform aggregation. To overcome these drawbacks, we propose an attention-based differentiable pooling operation in this paper, which can learn a hard cluster assignment for nodes and aggregate nodes in each cluster differently by introducing an attention mechanism. Experiments on standard graph classification benchmarks show that our proposed approach performs better when compared with other competing methods.
更多查看译文
关键词
attention-based
AI 理解论文
溯源树
样例
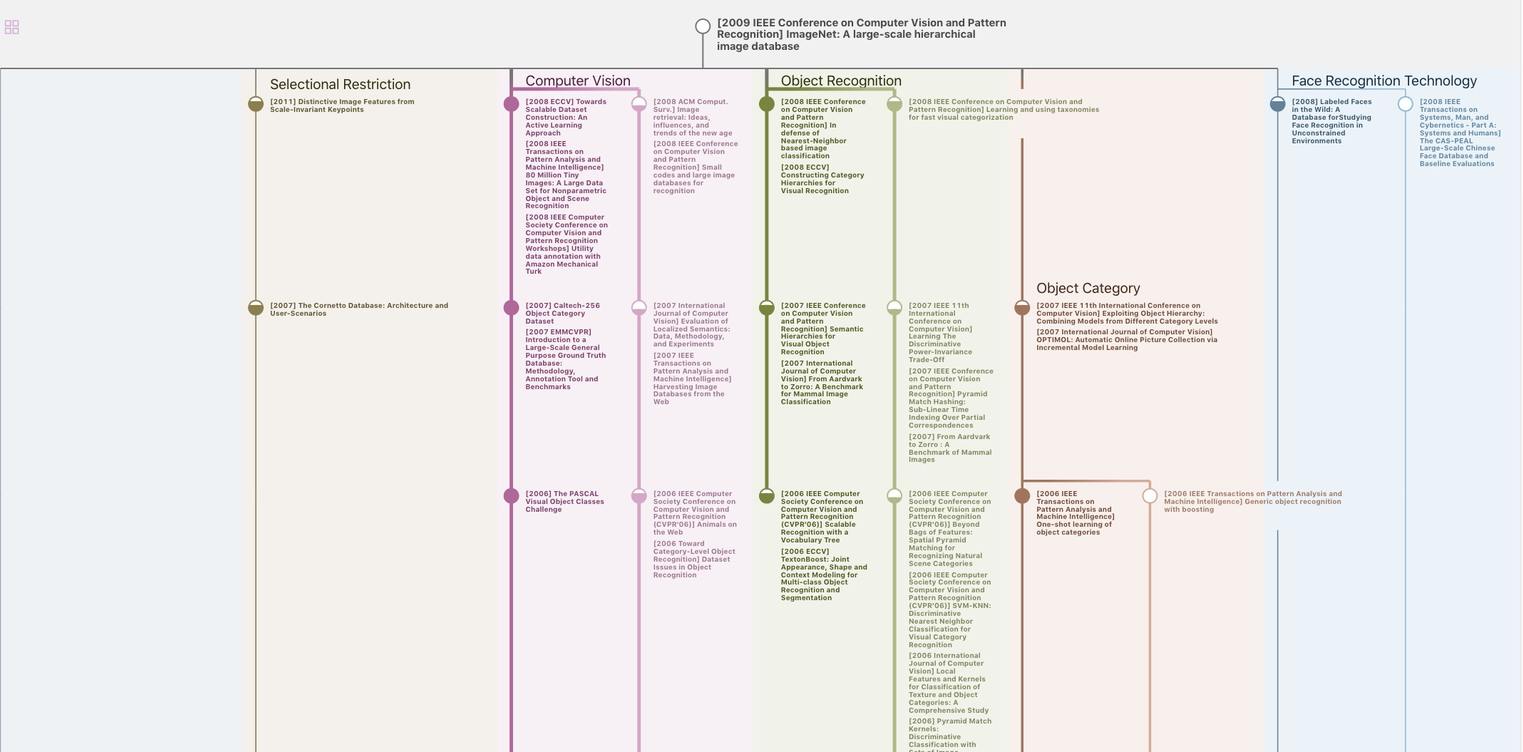
生成溯源树,研究论文发展脉络
Chat Paper
正在生成论文摘要