A Channel-Spatial Hybrid Attention Mechanism using Channel Weight Transfer Strategy.
ICPR(2022)
摘要
Attention is one of the most valuable breakthroughs in the deep learning community, and how to effectively utilize the attention information of channel and spatial is still one of the hot research topics. In this work, we integrate the advantages of channel and spatial mechanism to propose a Channel-Spatial hybrid Attention Module (CSHAM). Specifically, max-average fusion Channel Attention Module and Spatial Attention Neighbor Enhancement Module are firstly proposed, respectively. Then the connection between the two modules is analyzed and designed, and an alternate connection strategy with the transformation of channel weights is proposed. The key idea is to repeatedly use the channel weight information generated by the channel attention module, and to reduce the negative impact of the network complexity caused by the addition of the attention mechanism. Finally, a series of comparison experiments are conducted on CIFAR100 and Caltech-101 based on various backbone models. The results show that the proposed methods can obtain the best Top-1 performance among the existing popular methods, and can rise by nearly 1% in accuracy while basically maintaining the parameters and FLOPs. The code is publicly available at https://github.com/HuHaigen/A-Channel-Spatial-Hybrid-Attention-Mechanism-using-Channel-Weight-Transfer-Strategy. The package includes the proposed CSHAM for reproducibility purposes.
更多查看译文
关键词
channel-spatial weight transfer strategy,attention
AI 理解论文
溯源树
样例
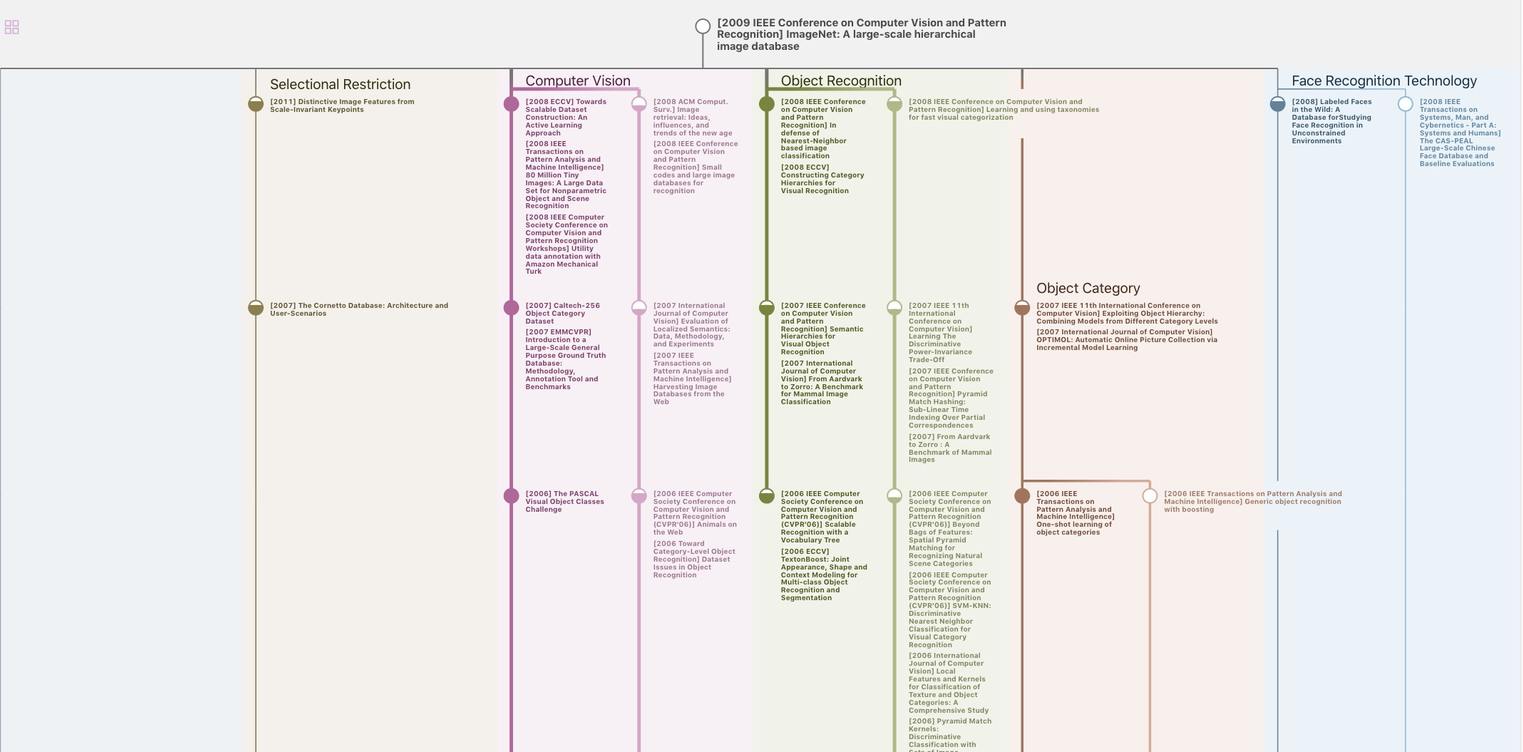
生成溯源树,研究论文发展脉络
Chat Paper
正在生成论文摘要