Learning Semantic Segmentation on Unlabeled Real-World Indoor Point Clouds via Synthetic Data.
ICPR(2022)
摘要
The data-hungry nature of deep learning and the high cost of annotating point-level labels for point clouds make it difficult to apply semantic segmentation methods to unlabeled real-world indoor scenes. Therefore, label-efficient point cloud segmentation has become a promising research topic. We noticed that the online housing design platforms can provide a large number of synthetic indoor 3D scenes, which are created with semantic labels. In this paper, we propose to learn semantic segmentation on synthetic point clouds and adapt the model for unlabeled real-world data. The main challenge is that directly using models trained on synthetic data for real-world data produces poor results due to the large domain gap between synthetic and real-world data. We design a point cloud style transfer network and a feature discrimination network to reduce the domain gap in both the input space and the feature space. Experiments show that our approach significantly improves the performance on real-world data for models learned from synthetic data.
更多查看译文
关键词
semantic segmentation,real-world
AI 理解论文
溯源树
样例
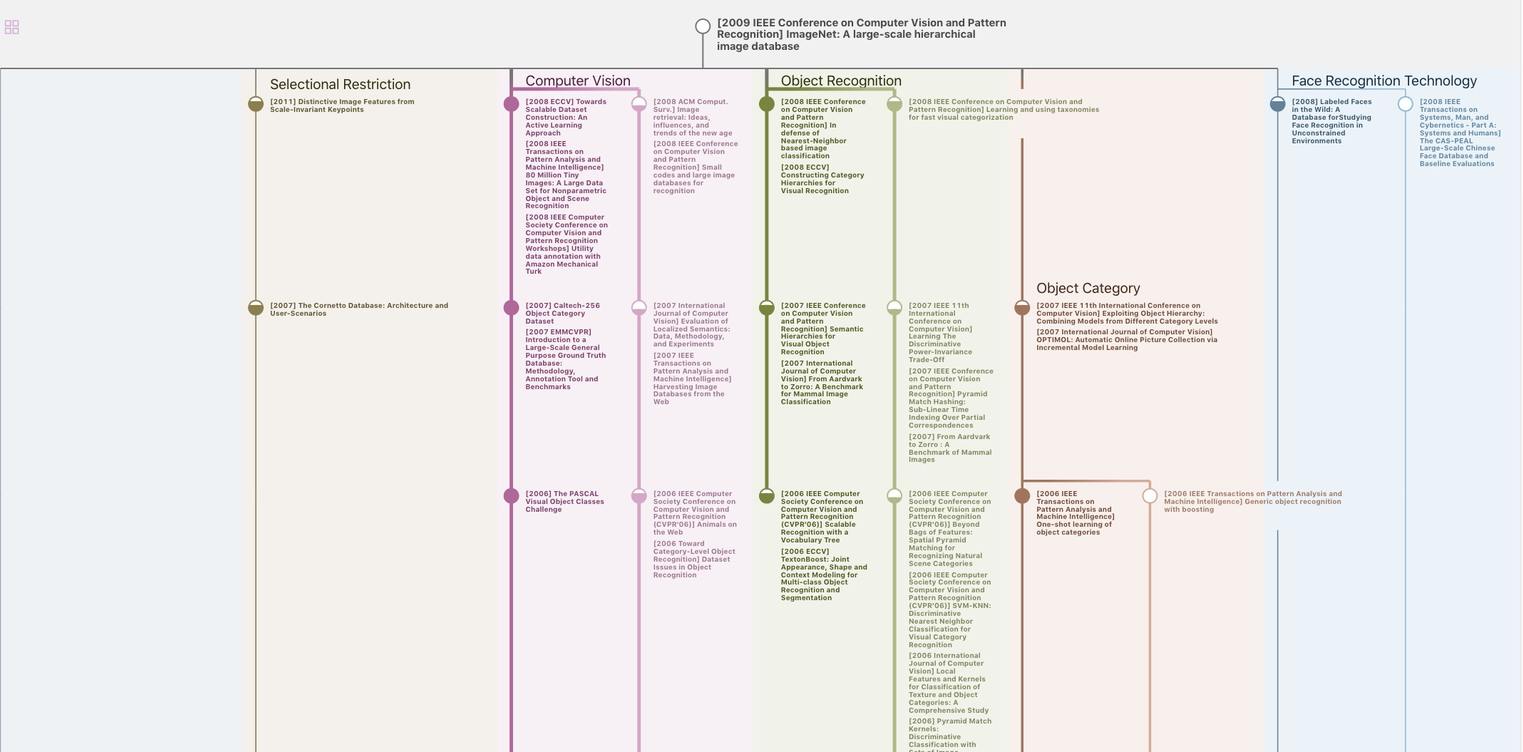
生成溯源树,研究论文发展脉络
Chat Paper
正在生成论文摘要