Quantitative Analysis of Object Detectors for Autonomous Driving and Autonomous Parking.
ICPR(2022)
摘要
State-of-the-art (SOTA) object detectors are generally evaluated on object detection challenge datasets. However, automotive domain-specific quantitative analysis of detectors is limited and often incomplete. Moreover, evaluation of detectors is mainly focused on average precision (AP) metric, ignoring AP's limitation such as insensitive to shape of the precision-recall curve. These issues are addressed in this paper by evaluating the most popular SOTA detectors on autonomous driving and autonomous parking datasets. AP weakness is addressed by evaluating detectors using the Localization-Recall-Precision (LRP) metric, which also provides a detailed understanding of a detector. Motivated from LRP, this paper also presents the optimal AP (oAP) metric for the fair comparison of detectors, which was ignored until now. The proposed oAP metric can be easily used with any object detector. In addition, a complete object detection pipeline starting from data collection to deployment on an embedded device is demonstrated in this work. Experimental results are presented graphically to analyze detector from a different perspective.
更多查看译文
关键词
AP limitation,AP weakness,autonomous driving,autonomous parking datasets,average precision metric,complete object detection pipeline,data collection,domain-specific quantitative analysis,localization-recall-precision metric,LRP metric,oAP metric,object detection challenge datasets,optimal AP metric,precision-recall curve,SOTA detectors,state-of-the-art object detectors
AI 理解论文
溯源树
样例
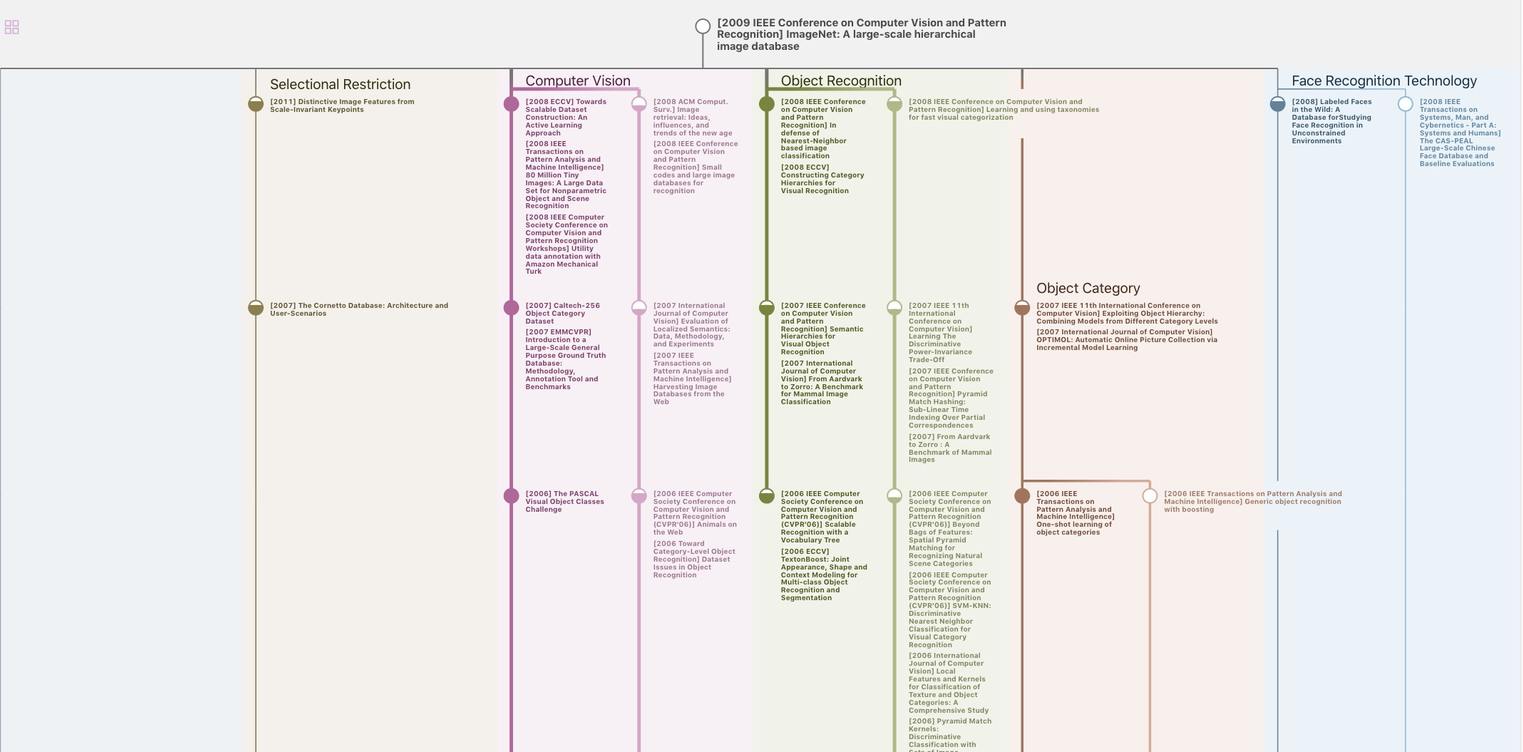
生成溯源树,研究论文发展脉络
Chat Paper
正在生成论文摘要