Continual Learning for Document Image Binarization.
ICPR(2022)
摘要
In the field of Document Image Analysis (DIA), it is common to find great heterogeneity in terms of the possible graphic domains. In this sense, it is interesting to build neural models that can be sequentially adapted to new domains without losing the knowledge from the domains already learned. This learning paradigm is known as Continual (or Lifelong) Learning (CL). Although the adaptation comes along with a training set of the new domain, neural networks suffer what is known as "catastrophic forgetting". Therefore, assuming the constraint of not keeping data from the domains already addressed, this paradigm represents a challenge yet to be solved. This work presents an approach for CL in document image binarization, one of the most considered tasks within the DIA field. Our results report that it is indeed feasible to address CL in this field, given that the approach is successfully implemented and outperforms the baseline by a wide margin in most of the analyzed scenarios.
更多查看译文
关键词
catastrophic forgetting,CL,Continual Learning,DIA field,Document Image Analysis,document image binarization,graphic domains,learning paradigm,lifelong learning,neural models,neural networks,training set
AI 理解论文
溯源树
样例
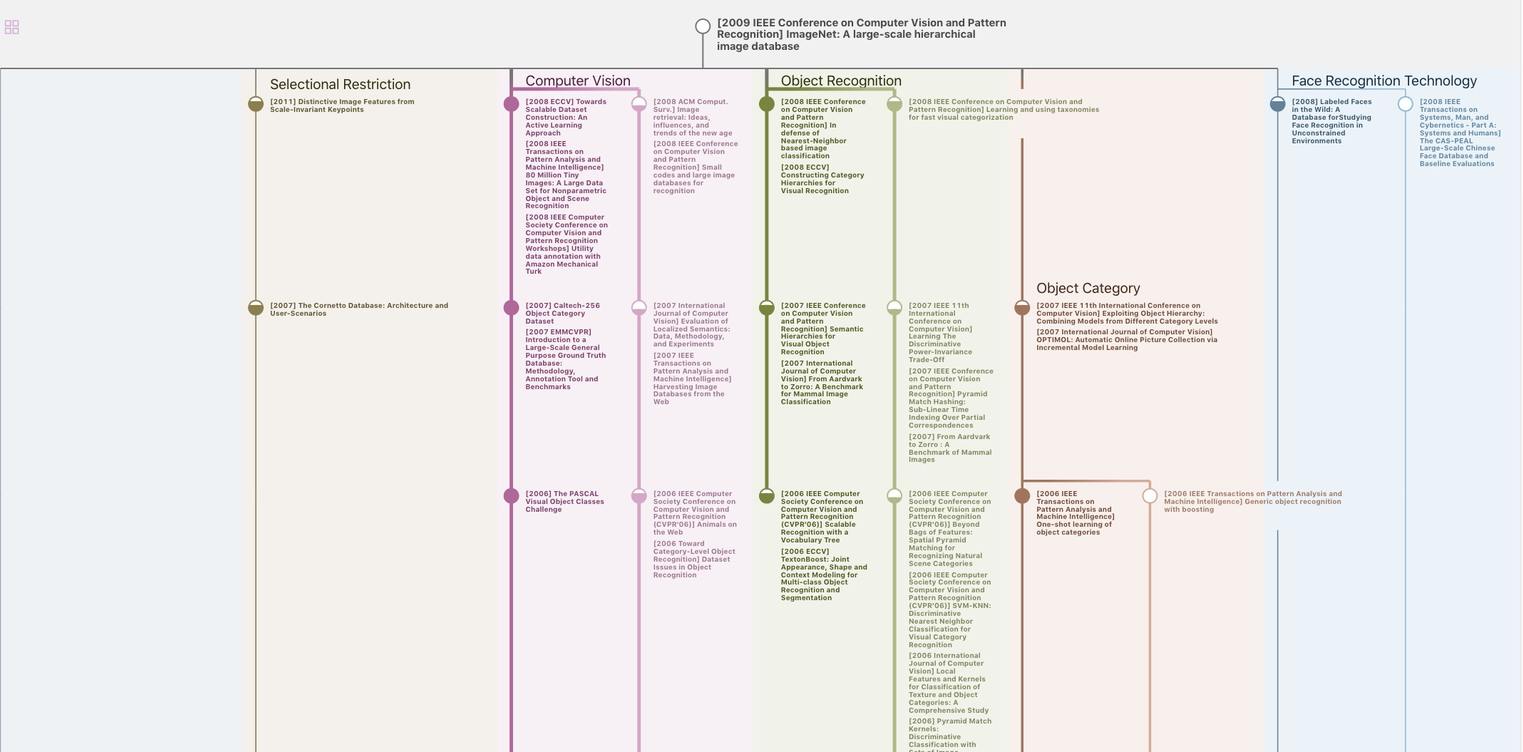
生成溯源树,研究论文发展脉络
Chat Paper
正在生成论文摘要