Defending Against Deepfakes with Ensemble Adversarial Perturbation.
ICPR(2022)
摘要
Maliciously manipulated images and videos, represented by prevalent deepfakes, can easily deceive human and mislead the public opinions. A great deal of effort was spent on detecting these fake images or videos. However, these detection methods always encounter various problems in practical applications. Do we have other ways to block the spread of fake image or videos? This motivates us to focus on an emerging interesting topic, disruption of deepfake generation. We propose the ensemble attacks of various types of deepfake models including facial attribute editing, face swapping and face reenactment models. With the help of hard model mining, we boost the attack success rate significantly comparing with the straightforward average ensemble. Extensive experiments demonstrate the proposed approach can successfully disrupt multiple deepfake models simultaneously under white-box or gray-box attack protocols.
更多查看译文
AI 理解论文
溯源树
样例
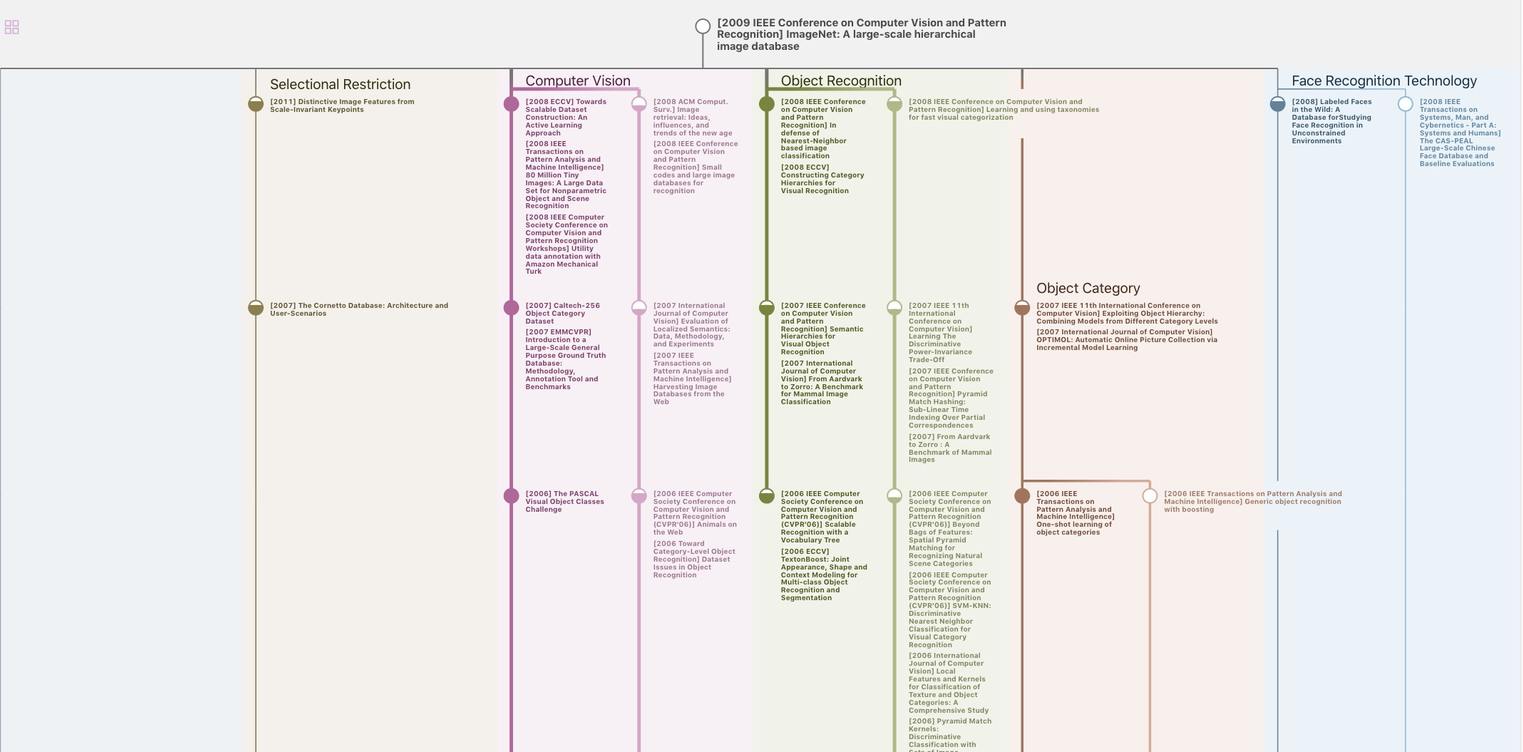
生成溯源树,研究论文发展脉络
Chat Paper
正在生成论文摘要