FP60 and FSNet: A Benchmark Dataset and a Family-Species Network for Forestry Pest Recognition.
ICPR(2022)
摘要
Forestry pest is like a non-smoke fire for causing a huge loss of forestry products; thus, timely detection and recognition for forestry pests can significantly reduce the loss. The performance of forestry pest recognition greatly depends on the quality of image dataset on which the state-of-the-art learning model can be trained. However, there is a lack of such a dataset that is specially collected for forestry pests. In this paper, we collect a forestry pest (FP60) dataset based on insect taxonomy, which contains 15 families with 7523 images that can be further divided into 60 species. This paper also designs a framework of family-species network (FSNet) for forestry pest recognition, which is a two-task model based on insect taxonomy. In FSNet, the first task is family classification that is to first detect forestry pests in a given image and then determine which families these pests belong to, and the second task is species classification that is to further identify the species of these pests based on their families. Extensive experimental results indicate that FSNet outperforms the state-of-the-art algorithms, especially in the case of increasing the number of pest categories. The FP60 dataset and algorithm codes used in this paper have been published on github.com/sk94747/FP60.
更多查看译文
关键词
benchmark dataset,family-species network,forestry pest dataset,forestry pest detection,forestry pest recognition,forestry products,FP60 dataset,FSNet dataset,image quality dataset,learning model,species classification,two-task model
AI 理解论文
溯源树
样例
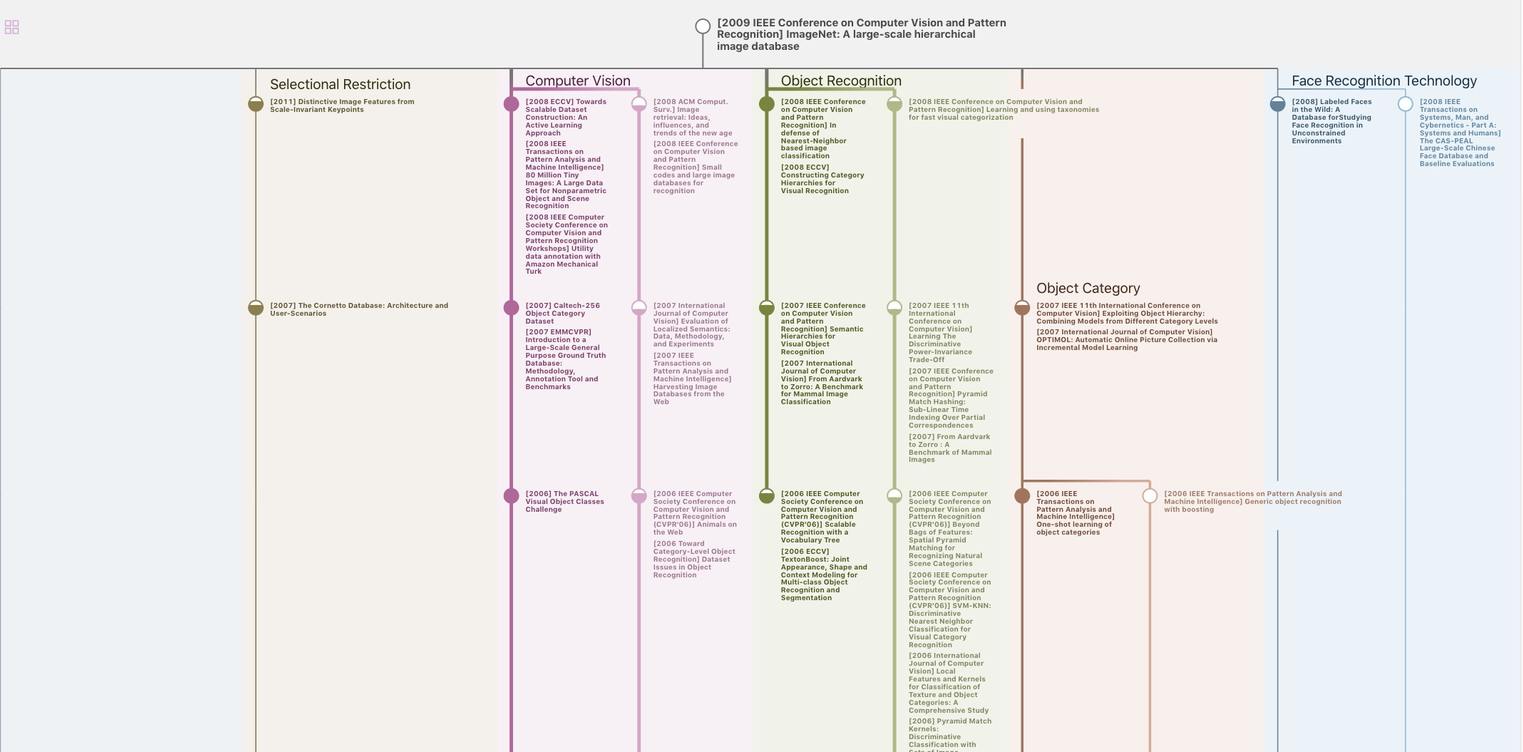
生成溯源树,研究论文发展脉络
Chat Paper
正在生成论文摘要