Learning Multi-Context Dynamic Listwise Relation for Generalizable Person Re-Identification.
ICPR(2022)
摘要
Although Person re-identification (Re-ID) has made rapid development in supervised learning and domain adaptation, it is more desirable to learn a generalizable model that can be directly applied to unseen scenes without updating. Generalizable Re-ID is challenging due to uncertain cross-camera variations in unseen target domain, such as illumination and viewpoint change, which result in visual ambiguities. Existing generalizable ReID methods focus on learning more generalizable features for individual instances. They ignore the context in the ranking list of the target domain. When human encounters visual ambiguities when matching pedestrians in unfamiliar scenes, comparing similar instances in the ranking list and comparing environments in different cameras can help remove ambiguities and refine matching results. This is actually exploiting contextual information in target domain, which is ignored by existing generalizable Re-ID methods. For learning contextual information to refine matching in unseen target domain, we propose a Multi-Context Dynamic Listwise Relation Network (MDLRN) to extract and aggregate instance-level and camera-level contextual features of a list of images, which can dynamically adapt the metric to unseen cross-domain scene variations. We further propose camera-specific feature perturbation (CFP) to simulate cross-camera variations in unseen target domain to improve generalization. Extensive experiments showed the superiority of our method in domain generalization.
更多查看译文
关键词
person re-identification,domain generalization,relation feature,context
AI 理解论文
溯源树
样例
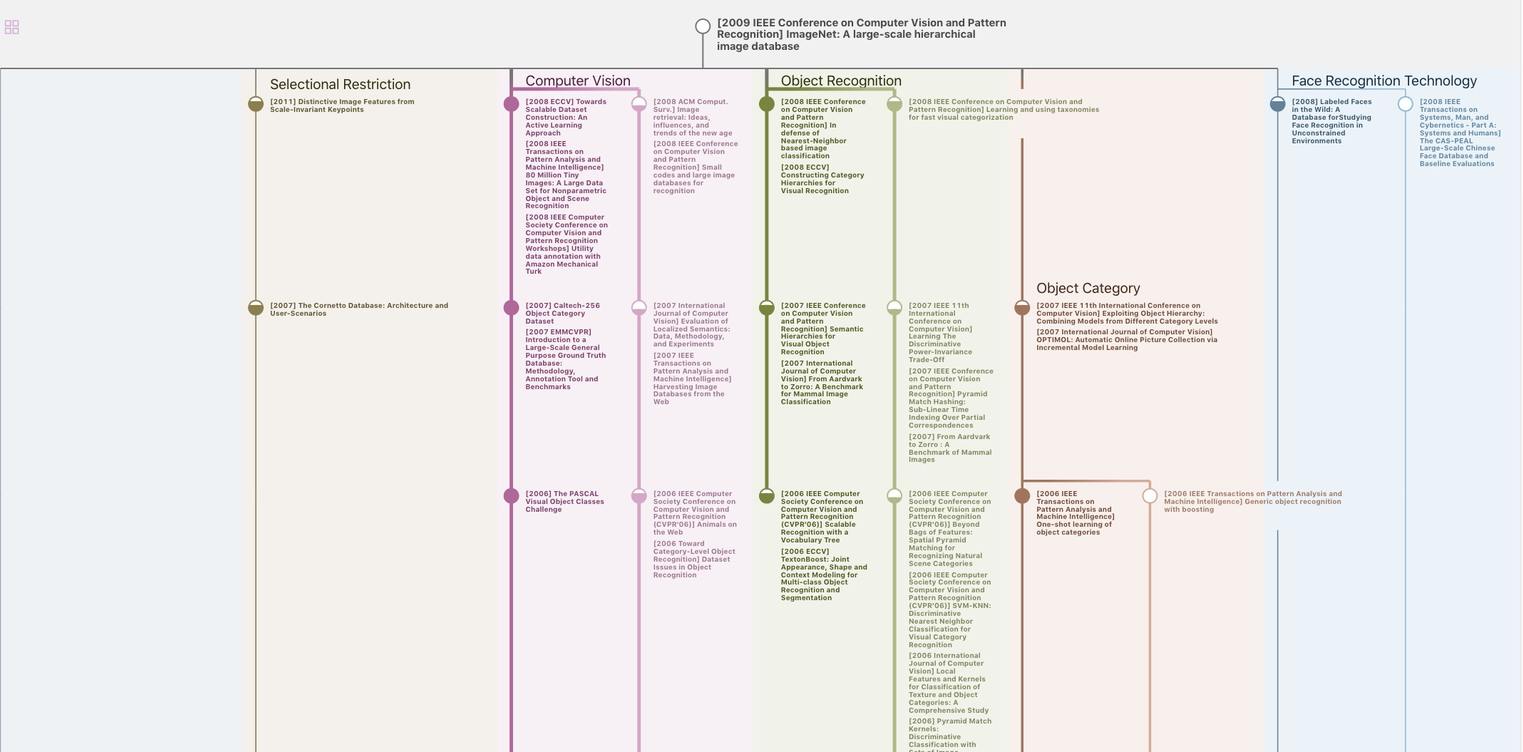
生成溯源树,研究论文发展脉络
Chat Paper
正在生成论文摘要