A Self-supervised Adversarial Learning Approach for Network Intrusion Detection System.
CNCERT(2022)
摘要
AbstractThe network intrusion detection system (NIDS) plays an essential role in network security. Although many data-driven approaches from the field of machine learning have been proposed to increase the efficacy of NIDSs, it still suffers from extreme data imbalance and the performance of existing algorithms depends highly on training datasets. To counterpart the class-imbalanced problem in network intrusion detection, it is necessary for models to capture more representative clues within same categories instead of learning from only classification loss. In this paper, we proposed a self-supervised adversarial learning approach for intrusion detection, which utilize instance-level discrimination for better representation learning and employs a adversarial perturbation styled data augmentation to improve the robustness of NIDS on rarely seen attacking types. State-of-the-art result was achieved on multiple frequently-used datasets and experiment conducted on cross-dataset setting demonstrated good generalization ability.
更多查看译文
关键词
network intrusion detection system,adversarial learning approach,self-supervised
AI 理解论文
溯源树
样例
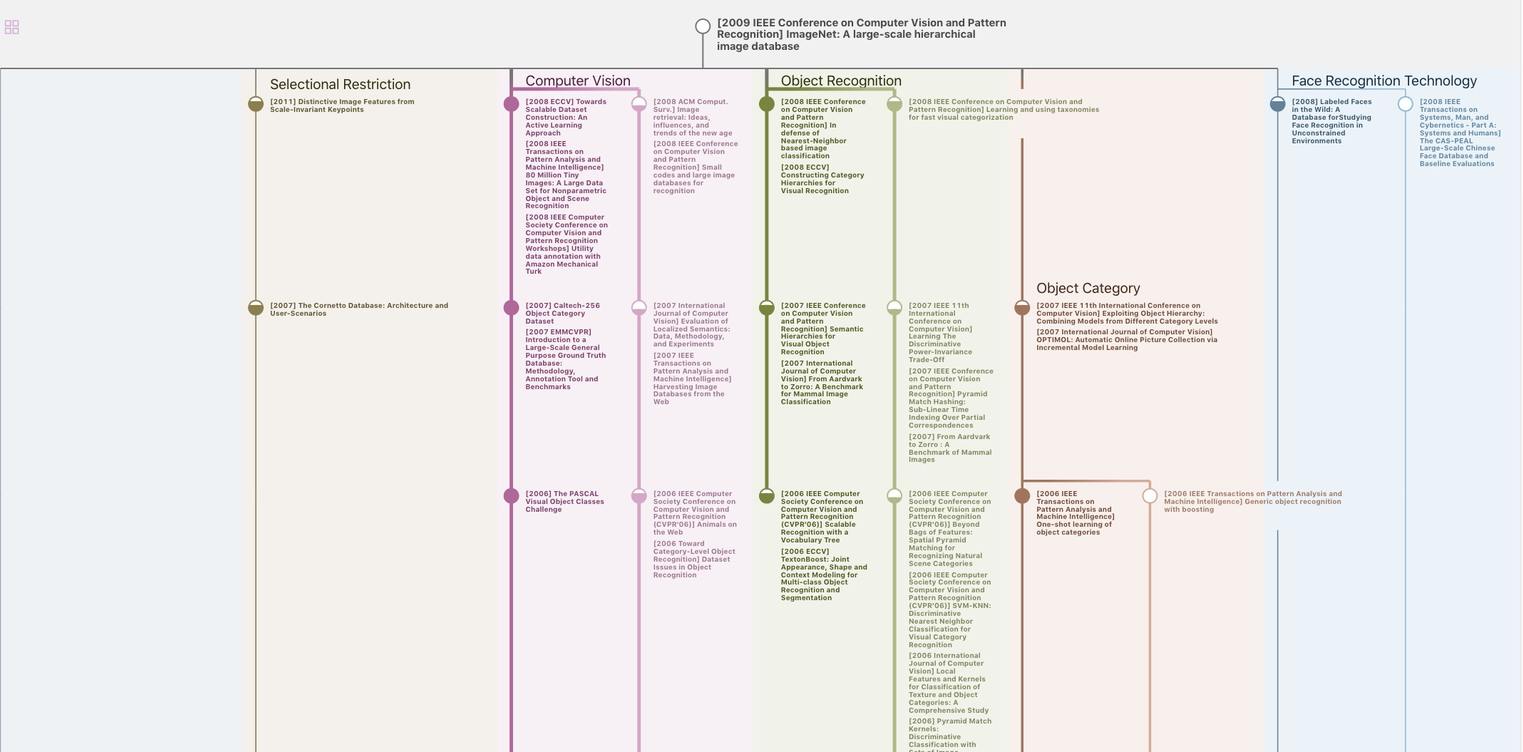
生成溯源树,研究论文发展脉络
Chat Paper
正在生成论文摘要