SVFLS: A Secure and Verifiable Federated Learning Training Scheme.
FCS(2022)
摘要
Federated learning has received extensive attention in recent years since the clients only need to share their local gradients with the servers without directly sharing their datasets to train the model. However, the existing research shows that the attackers can still reconstruct private information from shared gradients, resulting in privacy leakage. In addition, the aggregated results could be tampered with by servers or attackers. In this paper, we propose a secure and verifiable federated learning training scheme (SVFLS) to protect the privacy of data owners and verify aggregated results. Specifically, we employ threshold paillier encryption to protect the local gradients of data owners and use the bilinear aggregate signature to verify the correctness (or integrity) of aggregated results. Furthermore, our scheme can tolerate data owners dropping out during the training phase. We conduct extensive experiments on real datasets and demonstrate that our scheme is effective and practical.
更多查看译文
关键词
Homomorphic encryption,Bilinear aggregate signature,Privacy-preserving,Verifiable federated learning
AI 理解论文
溯源树
样例
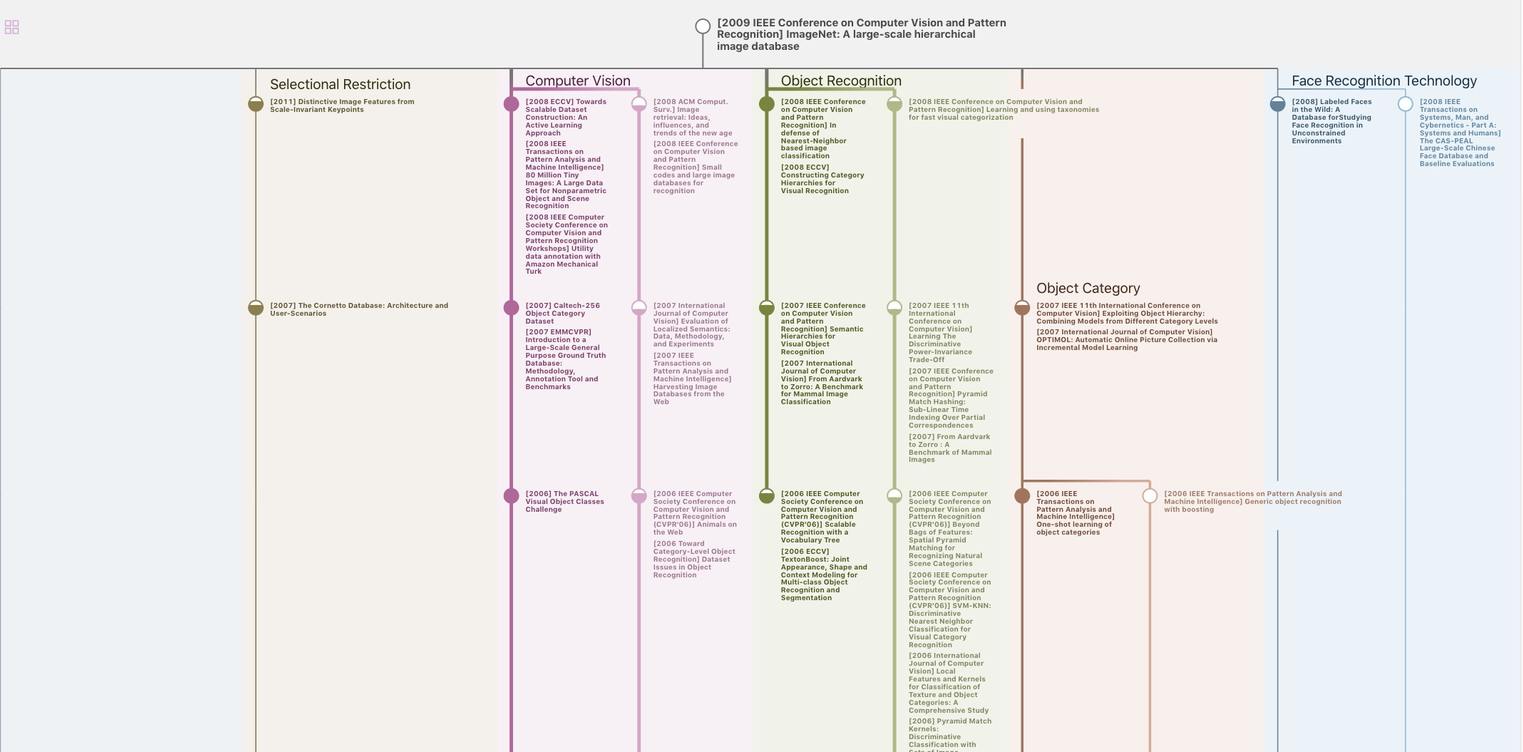
生成溯源树,研究论文发展脉络
Chat Paper
正在生成论文摘要