Diffusion-Based Approach to Style Modeling in Expressive TTS.
BRACIS (1)(2022)
摘要
In this article, we propose an aggregation of denoising diffusion probabilistic models (DDPMs) onto an end-to-end text-to-speech system to learn a distribution of reference speaking styles in an unsupervised manner. By applying a few steps of a forward noising process to an embedding extracted from a reference mel spectrogram, we make profit of its information to reduce the diffusion chain and reconstruct an improved style embedding with only a few reverse steps, performing style transfer. Additionally, a proposed combination of spectrogram reconstruction and denoising losses allows for conditioning of the acoustic model on the synthesized style embeddings. A subjective perceptual evaluation is conducted to evaluate naturalness and style transfer capability of the proposed approach. The results show a 5-point increment on the mean of naturalness ratings and a preference of the raters (43%) of our proposed approach over state-of-the-art models (29%) in the style transfer scenario.
更多查看译文
关键词
style modeling,diffusion-based
AI 理解论文
溯源树
样例
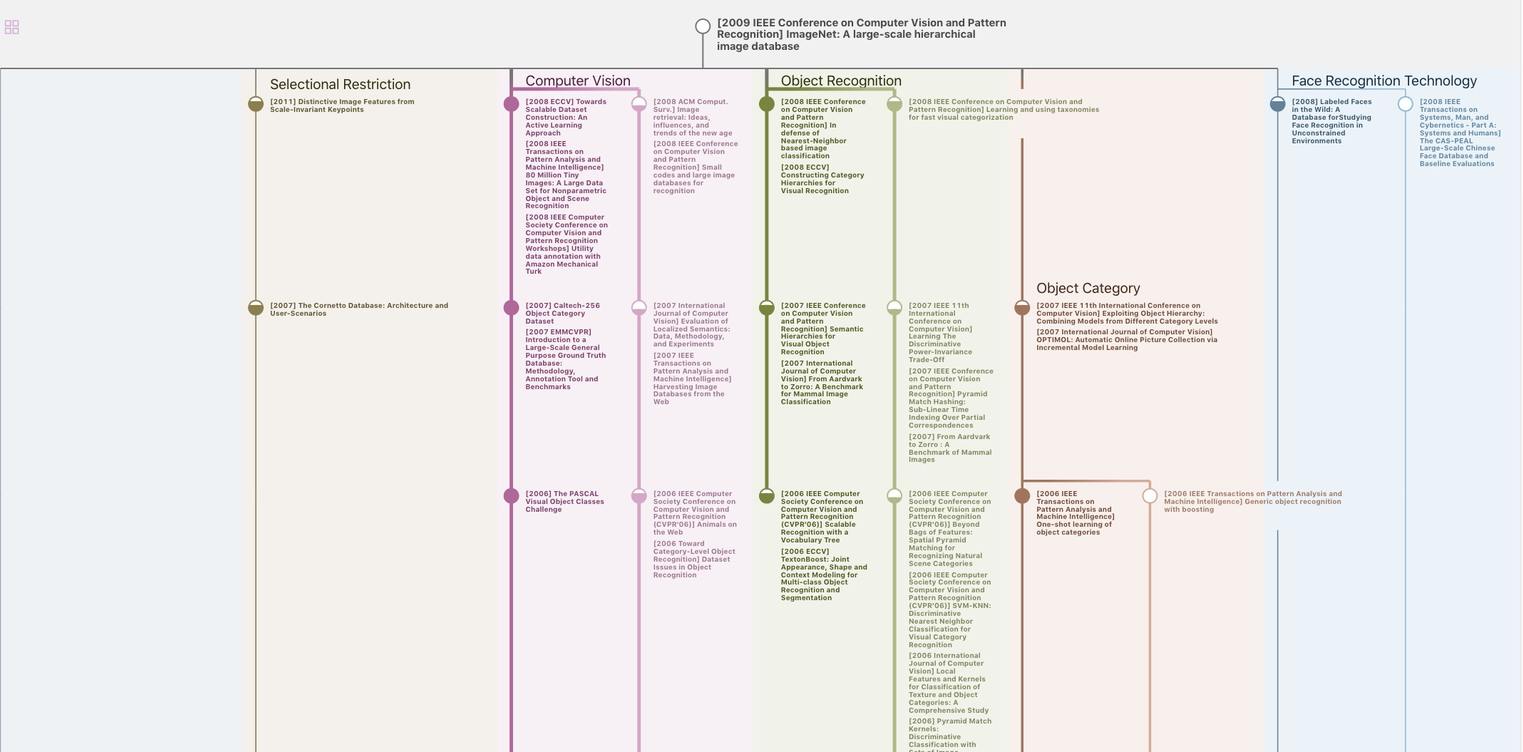
生成溯源树,研究论文发展脉络
Chat Paper
正在生成论文摘要