Generating Diverse Clustering Datasets with Targeted Characteristics.
BRACIS (1)(2022)
摘要
When evaluating clustering algorithms, it is important to assess their performance in retrieving clusters of datasets with known structures. Nonetheless, generating and choosing diverse datasets to compose such test benchmarks is non-trivial. The datasets must present a large variety of structures and characteristics so that the algorithms can be challenged and their strengths and weaknesses can be revealed. The use of generators currently available in the literature relies on trial and error procedures that can be quite costly and inaccurate. Taking advantage of an Instance Space Analysis of popular clustering benchmarks, where datasets are projected into a 2-D embedding with linear trends according to different characteristics, we use a genetic algorithm to produce new datasets at targeted locations in the instance space. This is a natural extension of the Instance Space Analysis framework, and as a result, we are able to produce diverse datasets for composing test benchmarks for clustering.
更多查看译文
关键词
diverse clustering datasets,targeted
AI 理解论文
溯源树
样例
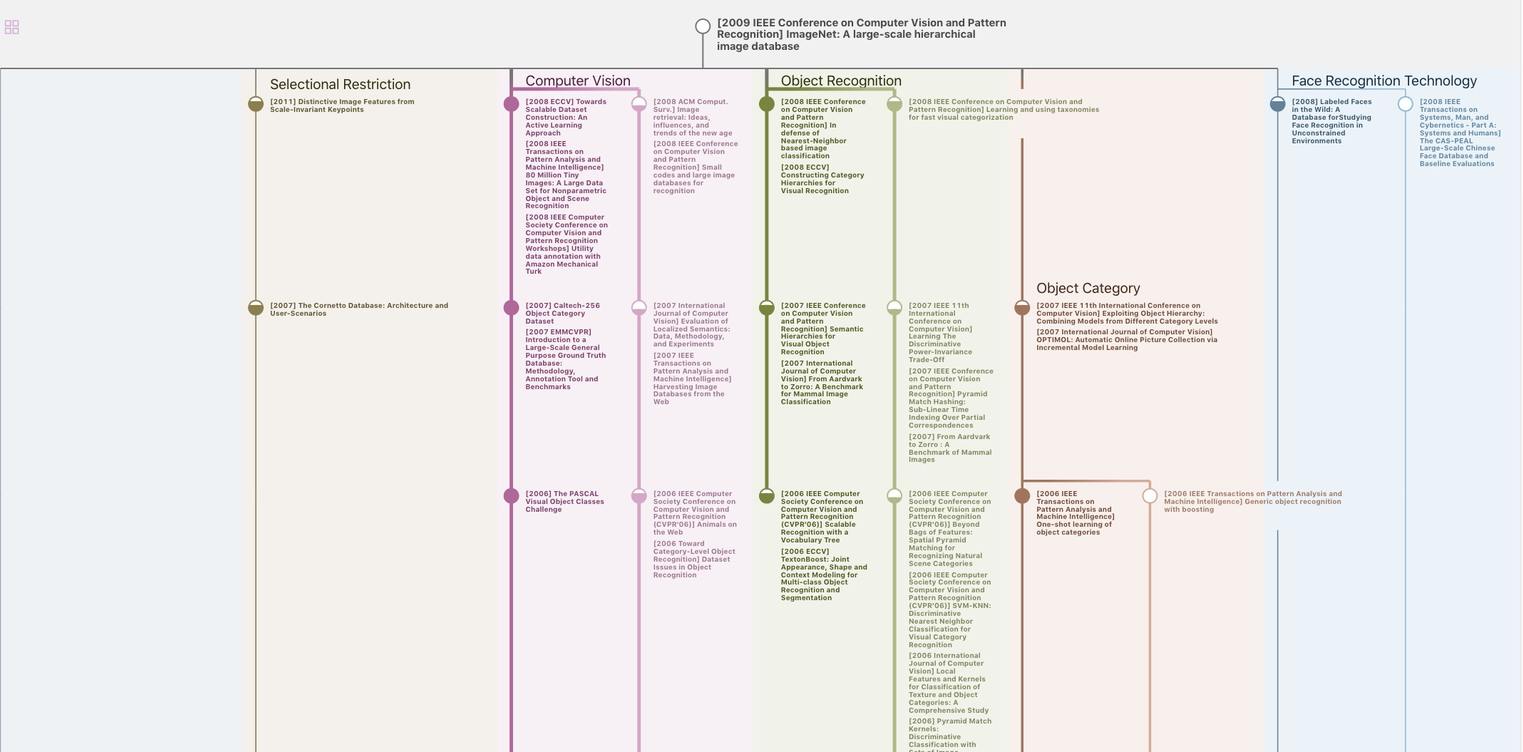
生成溯源树,研究论文发展脉络
Chat Paper
正在生成论文摘要