Convolutional Neural Network Approach for Multiple Sclerosis Lesion Segmentation.
IDEAL(2022)
摘要
Nowadays Deep Learning (DL) based automatic segmentation has outperformed traditionalmethods. In the present paper, we are interested in automatic MS lesion segmentation of 2D images based on DL techniques. The main challenge consists in proposing a new model that takes advantage of referenced CNN models: U-Net, ResNet, and DenseNet with a reduced number of parameters and a shorter execution time. To evaluate the proposed approach named "Concat-U-Net", we compared its performance to those of three implemented models, namely U-Net, U-ResNet, and Dense-U-Net. Furthermore, we employed just one modality (FLAIR) from the public ISBI dataset to segment MS lesions accurately. The best Dice value obtained was 0.73, which outperformed those reported in the literature. Our approach reduced the elapsed execution time from 48 s to 7 s. By reducing the number of parameters, an 85.42% time gain was achieved.
更多查看译文
关键词
Deep Learning, Multiple sclerosis segmentation, MRI, Data augmentation
AI 理解论文
溯源树
样例
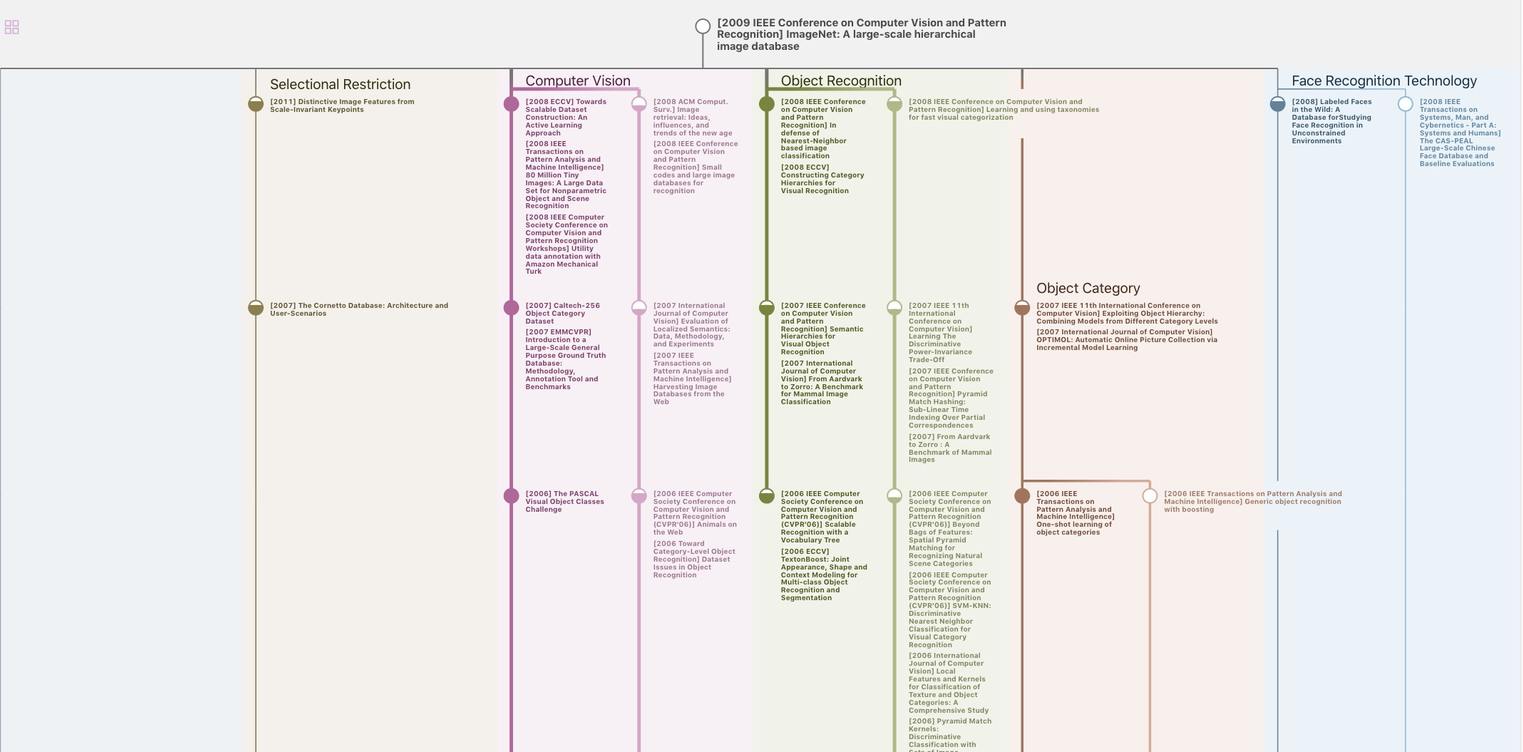
生成溯源树,研究论文发展脉络
Chat Paper
正在生成论文摘要