A New Approach to Visual Classification Using Concatenated Deep Learning for Multimode Fusion of EEG and Image Data.
ISVC (1)(2022)
摘要
In this work, we explore various approaches for automated visual classification of multimodal inputs such as EEG and Image data for the same item, focusing on finding an optimal solution. Our new technique examines the fusion of EEG and Image data using a concatenation of deep learning models for classification, where the EEG feature space is encoded with 8-bit-grayscale images. This concatenated-based model achieves a 95% accuracy for the 39 class EEG-ImageNet dataset, setting a new benchmark and surpassing all prior work. Furthermore, we show that it is computationally effective in multimodal classification when human subjects are presented with visual stimuli of objects in three-dimensional real-world space rather than images of the same. These findings will improve machine visual perception and bring it closer to human-learned vision.
更多查看译文
关键词
EEG, Images, Multimodal visual classification, Deep learning, Machine vision
AI 理解论文
溯源树
样例
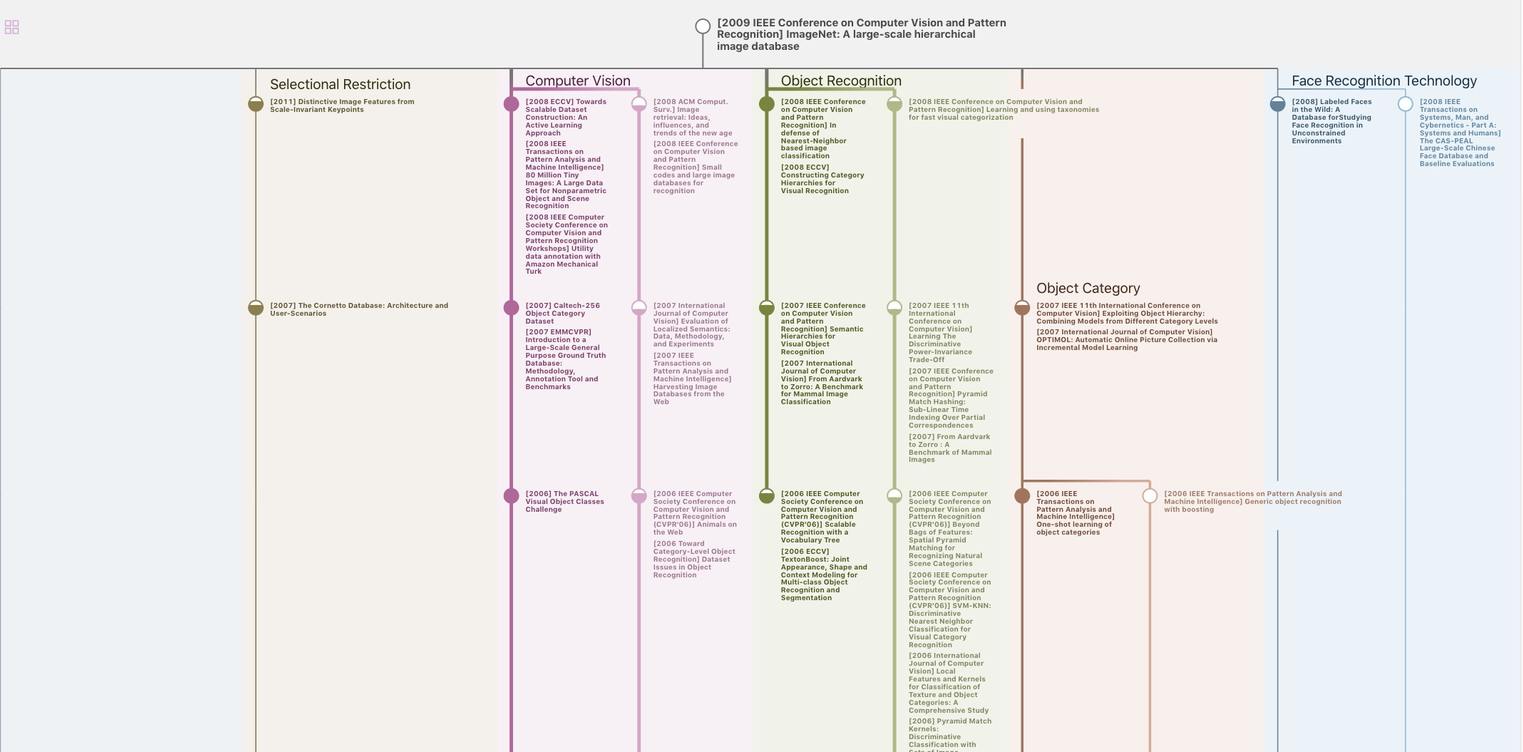
生成溯源树,研究论文发展脉络
Chat Paper
正在生成论文摘要