A Bi-directional Category-Aware Multi-task Learning Framework for Missing Check-in POI Identification.
ICSOC(2022)
摘要
The prevalence of Location-based Social Networks (LBSNs) services makes next personalized Point-of-Interest (POI) prediction become a research topic. However, due to device failure or intention camouflage, geolocation information missing prevents existing POI-oriented studies for advanced user preference analysis. Herein, we proposed a Bi-directional category-aware multi-task learning (Bi-CatMTL) framework, which fuses bi-direction spatiotemporal transition patterns and personalized dynamic preference to identify where the user has been at a past specific time, namely missing POI identification. Specifically, Bi-CatMTL introduces: (1) a two-channel encoder, i.e., spatial-aware POI encoder and temporal-aware category encoder, to capture user bidirectional dual-grained mobility transition patterns; (2) a task-oriented decoder to fuse learned transition patterns and personalized preference for multi-task prediction; (3) a POI2Cat matrix to make full use of both types of sequential dependencies. Extensive experiments demonstrate the superiority of our model, and it can also be adaptively extended to next POI prediction task with the convincing performance.
更多查看译文
关键词
Missing check-in POI identification,Spatial-aware POI encoder,Temporal-aware category encoder
AI 理解论文
溯源树
样例
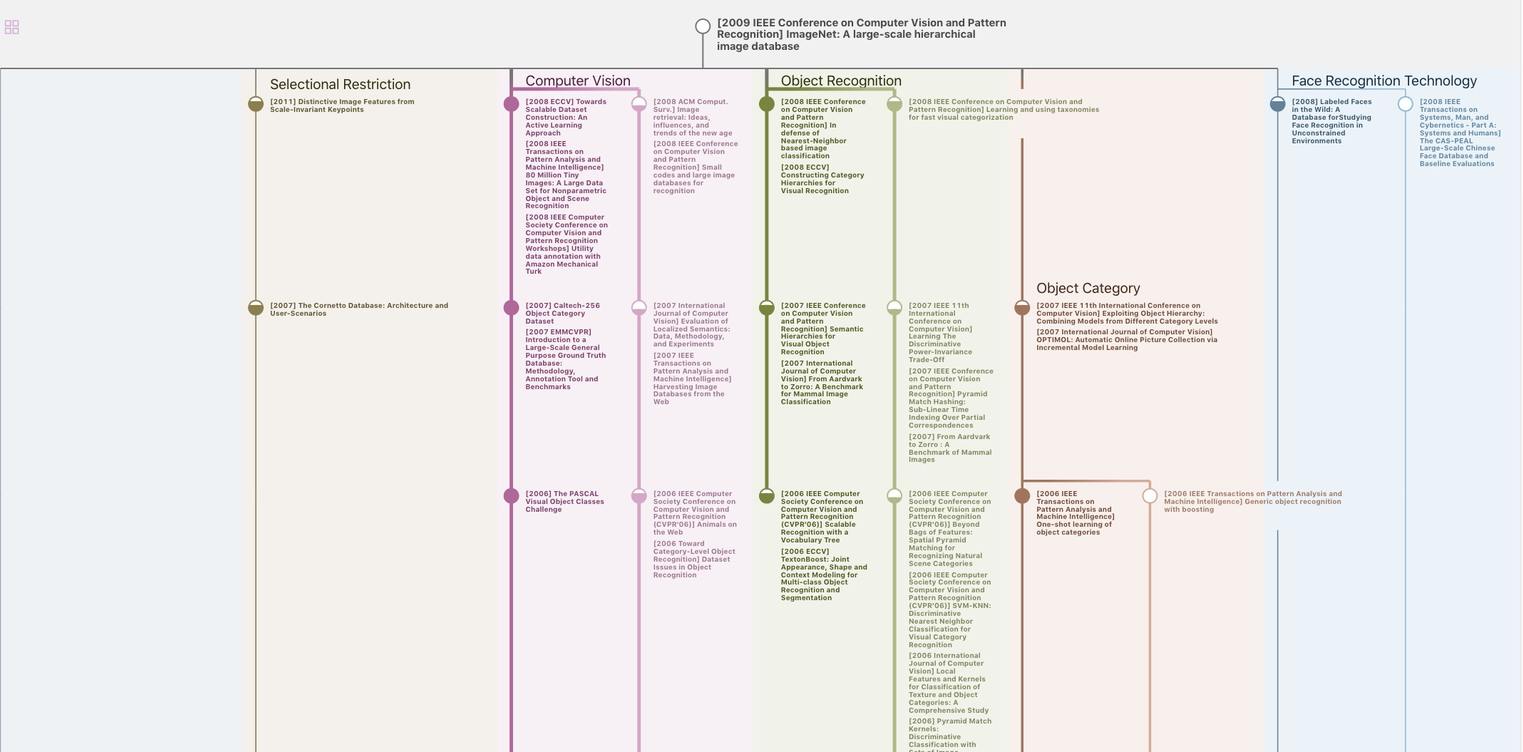
生成溯源树,研究论文发展脉络
Chat Paper
正在生成论文摘要