Metric Learning on Complex Projective Spaces.
ICSM(2022)
摘要
Shape analysis of landmarks is a fundamental problem in computer vision and multimedia. We propose a family of metrics called Fubini-Study distances defined in the complex projective space based on the seminal work of Kendall [ 11 ] for metric learning to measure the similarity between shape representations which are modeled directly by the equivalence classes of the 2D landmark configurations. Experiments conducted on the face landmarks for facial expression recognition demonstrate the competitiveness of the proposed method with respect to state-of-the-art approaches. A comparison with the metric defined in the Euclidean space has also been explored, proving that the Fubini-Study metric is more effective and discriminative than the Euclidean metric in identifying facial deformation.
更多查看译文
关键词
complex,learning
AI 理解论文
溯源树
样例
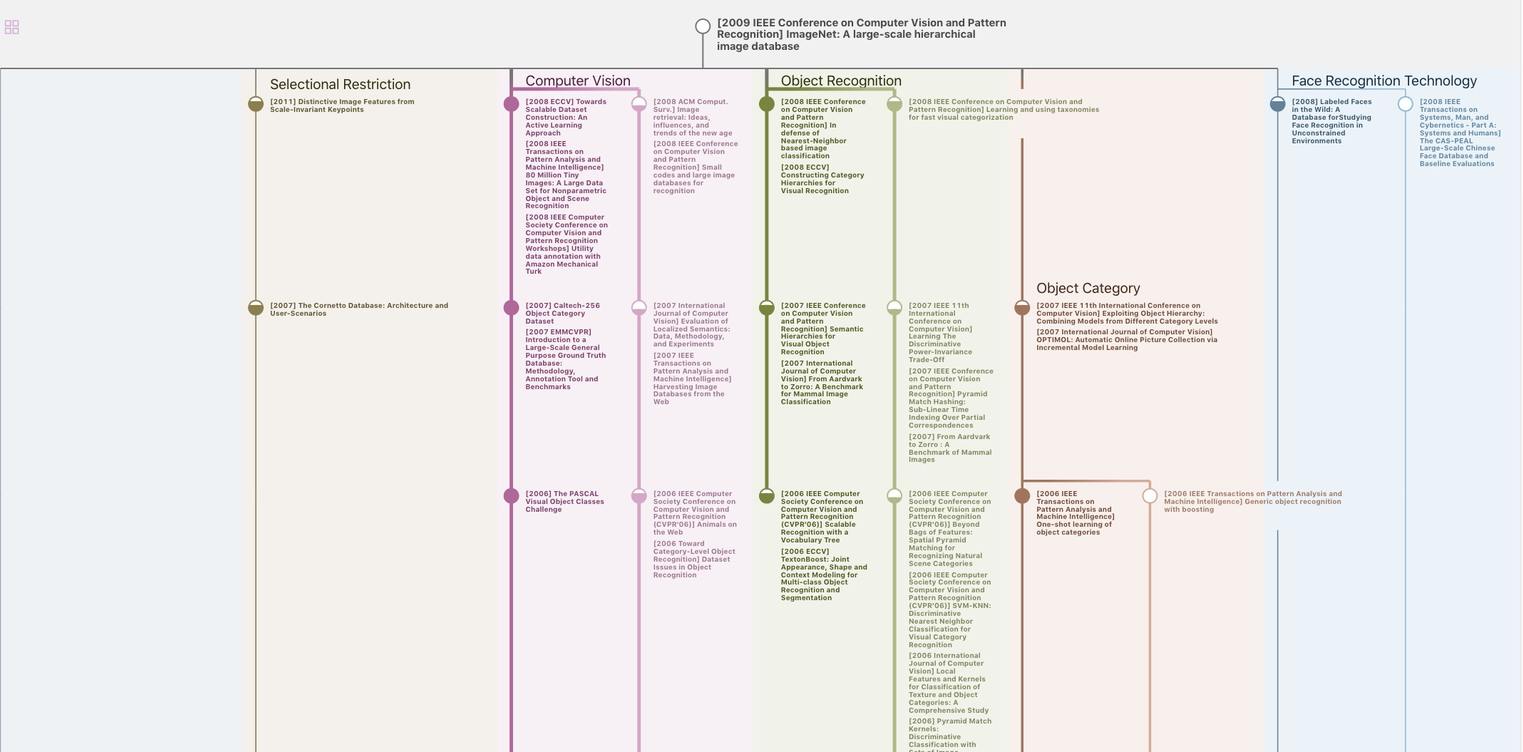
生成溯源树,研究论文发展脉络
Chat Paper
正在生成论文摘要