Maximum Information Coverage and Monitoring Path Planning with Unmanned Surface Vehicles Using Deep Reinforcement Learning.
OLA(2022)
摘要
Manual monitoring large water reservoirs is a complex and high-cost task that requires many human resources. By using Autonomous Surface Vehicles, informative missions for modeling and supervising can be performed efficiently. Given a model of the uncertainty of the measurements, the minimization of entropy is proven to be a suitable criterion to find a surrogate model of the contamination map, also with complete coverage pathplanning. This work uses Proximal Policy Optimization, a Deep Reinforcement Learning algorithm, to find a suitable policy that solves this maximum information coverage path planning, whereas the obstacles are avoided. The results show that the proposed framework outperforms other methods in the literature by 32% in entropy minimization and by 63% in model accuracy.
更多查看译文
关键词
Deep reinforcement learning, Informative path planning, Autonomous surface vehicles
AI 理解论文
溯源树
样例
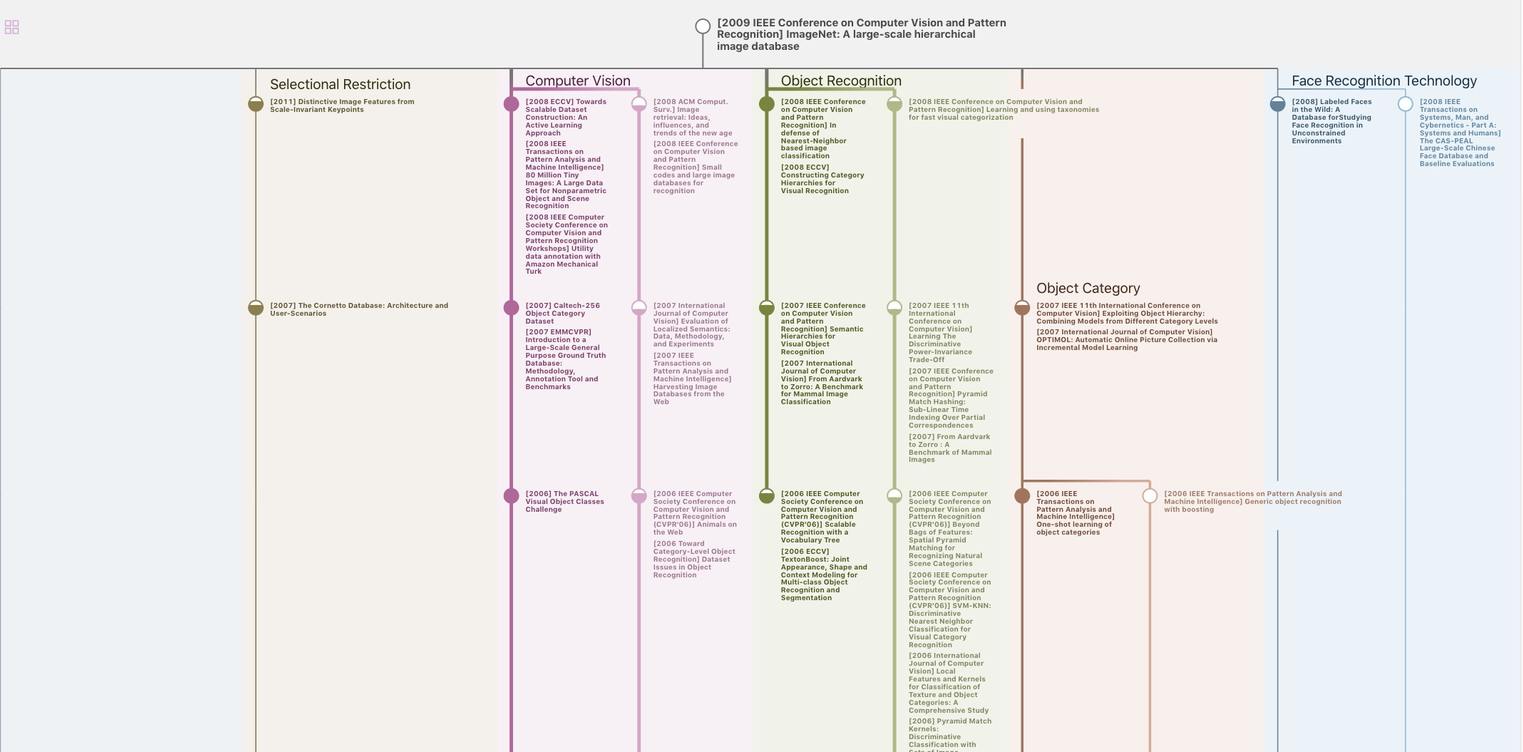
生成溯源树,研究论文发展脉络
Chat Paper
正在生成论文摘要