CarbonCast: multi-day forecasting of grid carbon intensity.
BuildSys@SenSys(2022)
摘要
The ever-increasing demand for energy is resulting in considerable carbon emissions from the electricity grid. In recent years, there has been growing attention on demand-side optimizations to reduce carbon emissions from electricity usage. A vital component of these optimizations is short-term forecasting of the carbon intensity of the grid-supplied electricity. Many recent forecasting techniques focus on day-ahead forecasts, but obtaining such forecasts for longer periods, such as multiple days, while useful, has not gotten much attention. In this paper, we present CarbonCast, a machine-learning-based hierarchical approach that provides multi-day forecasts of the grid's carbon intensity. CarbonCast uses neural networks to first generate production forecasts for all the electricity-generating sources. It then uses a hybrid CNN-LSTM approach to combine these first-tier forecasts with historical carbon intensity data and weather forecasts to generate a carbon intensity forecast for up to four days. Our results show that such a hierarchical design improves the robustness of the predictions against the uncertainty associated with a longer multi-day forecasting period. We also analyze which factors most influence the carbon intensity forecasts of any region with a specific mixture of electricity-generating sources. We show that CarbonCast's 4-day forecasts have a MAPE of 4.80--13.93% across six geographically distributed regions while outperforming state-of-the-art methods. Importantly, CarbonCast is the first open-sourced tool for multi-day carbon intensity forecasts where the code and data are freely available to the research community.
更多查看译文
关键词
grid carbon intensity, multi-day forecasting, hierarchical design, source production forecasts, machine learning
AI 理解论文
溯源树
样例
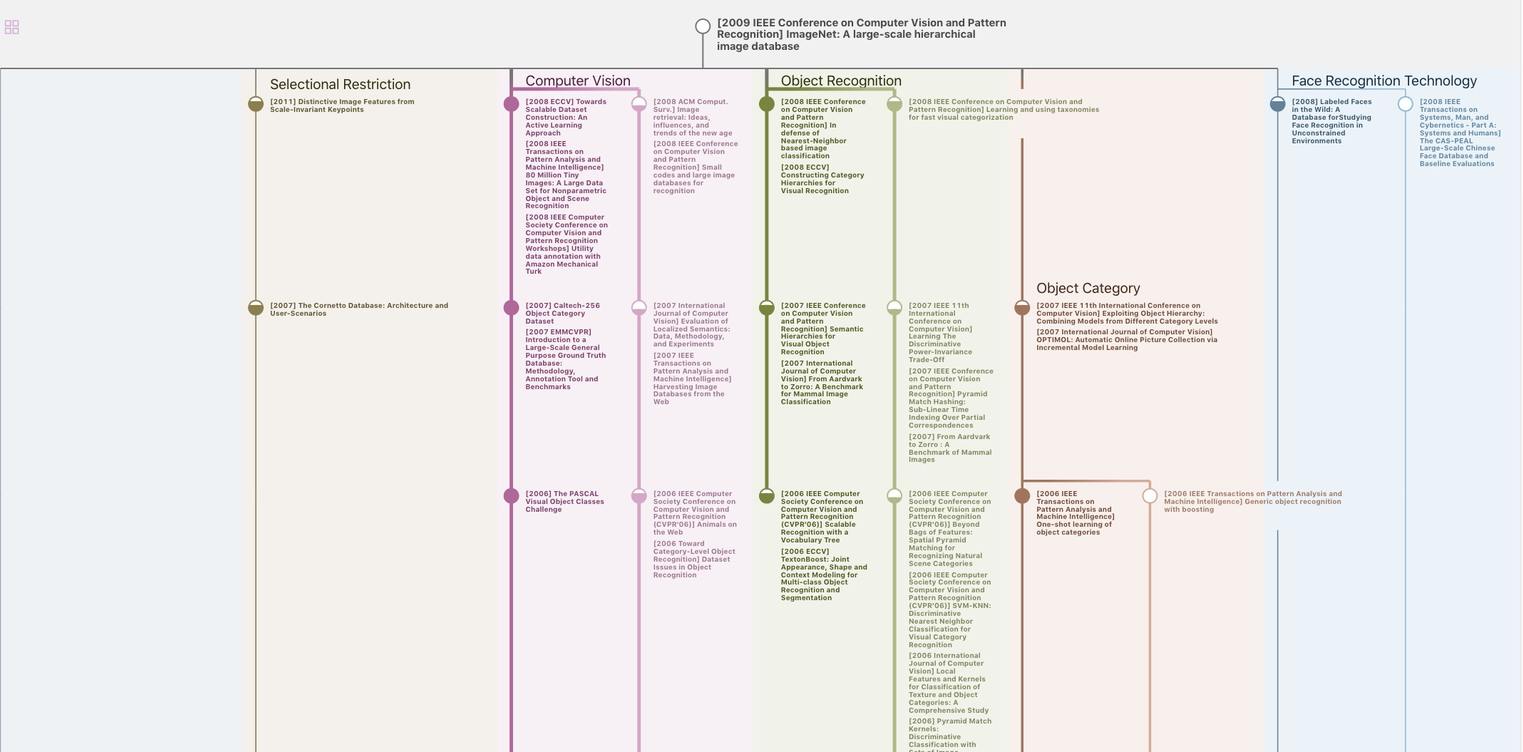
生成溯源树,研究论文发展脉络
Chat Paper
正在生成论文摘要