Focus on what matters: improved feature selection techniques for personal thermal comfort modelling.
BuildSys@SenSys(2022)
摘要
Occupants' personal thermal comfort (PTC) is indispensable for their well-being, physical and mental health, and work efficiency. Predicting PTC preferences in a smart home can be a prerequisite to adjusting the indoor temperature for providing a comfortable environment. In this research, we focus on identifying relevant features for predicting PTC preferences. We propose a machine learning-based predictive framework by employing supervised feature selection techniques. We apply two feature selection techniques to select the optimal sets of features to improve the thermal preference prediction performance. The experimental results on a public PTC dataset demonstrated the efficiency of the feature selection techniques that we have applied. In turn, our PTC prediction framework with feature selection techniques achieved state-of-the-art performance in terms of accuracy, Cohen's kappa, and area under the curve (AUC), outperforming conventional methods.
更多查看译文
关键词
Machine Learning, Feature Selection, Thermal Comfort Modelling
AI 理解论文
溯源树
样例
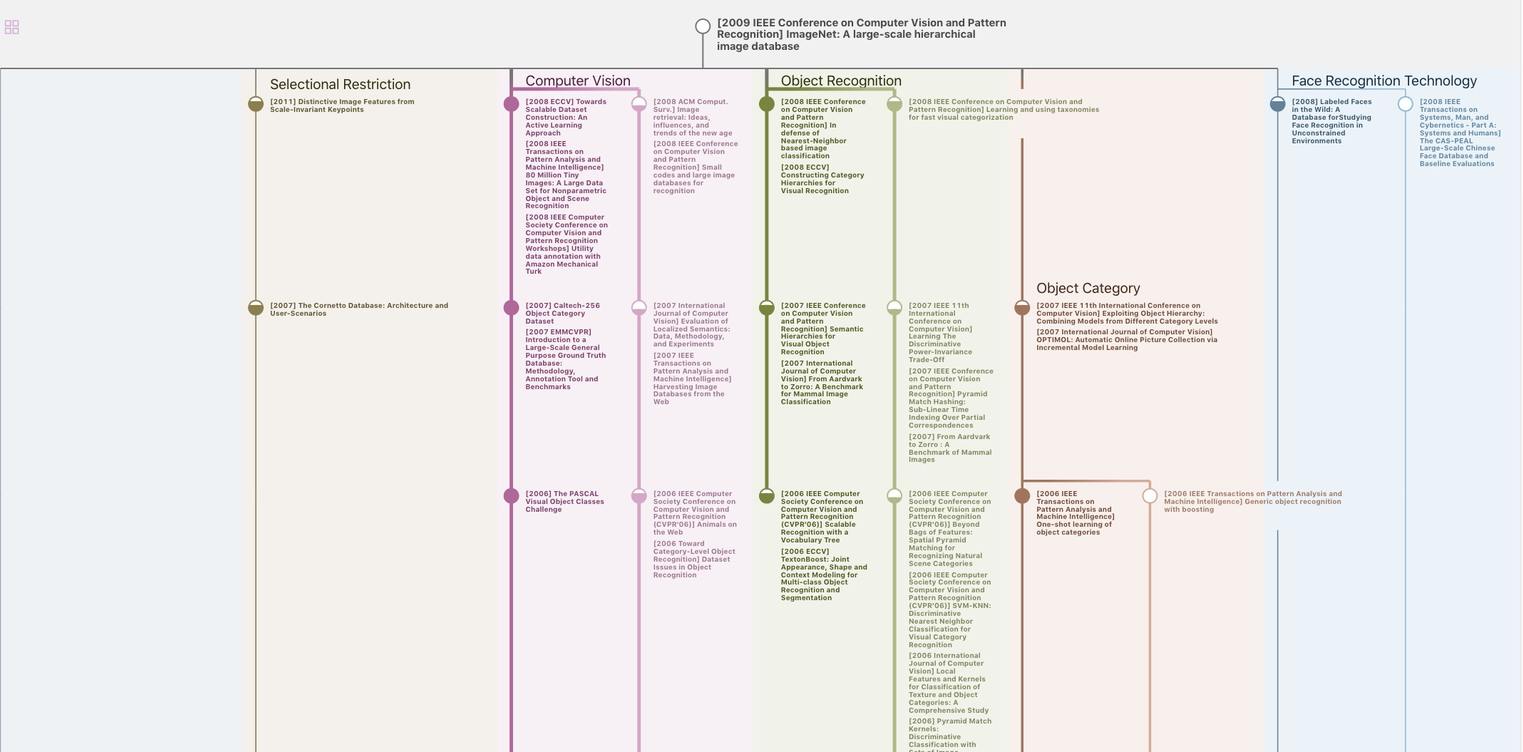
生成溯源树,研究论文发展脉络
Chat Paper
正在生成论文摘要