Poster Abstract: Privacy-Preserving Data Augmentation for Thermal Sensation Dataset Based on Variational Autoencoder
BuildSys@SenSys(2022)
摘要
Machine learning-based methods show high performance in estimating the thermal sensation of a person. These methods are based on a huge amount of personal data. The dataset used for the training of the estimator includes personal physiological data, which includes people's private information. Generative models have received significant attention to anonymize such data, including private information. In this paper, we propose privacy-preserving data augmentation for the thermal sensation dataset, including the subject's physiological data using Variational Autoencoder. The generative model trained with a thermal sensation dataset collected in the uncontrolled environment tends to be biased because subjects in the environment rarely report extreme thermal sensation labels. To tackle this problem, we introduce a weighted loss function for the generative model to mitigate the bias of the thermal sensation labels. The evaluation result shows that our method generates an anonymized dataset that works to train a thermal sensation estimator as well as the original dataset.
更多查看译文
关键词
Data Augmentation, Data Anonymization, Thermal Sensation
AI 理解论文
溯源树
样例
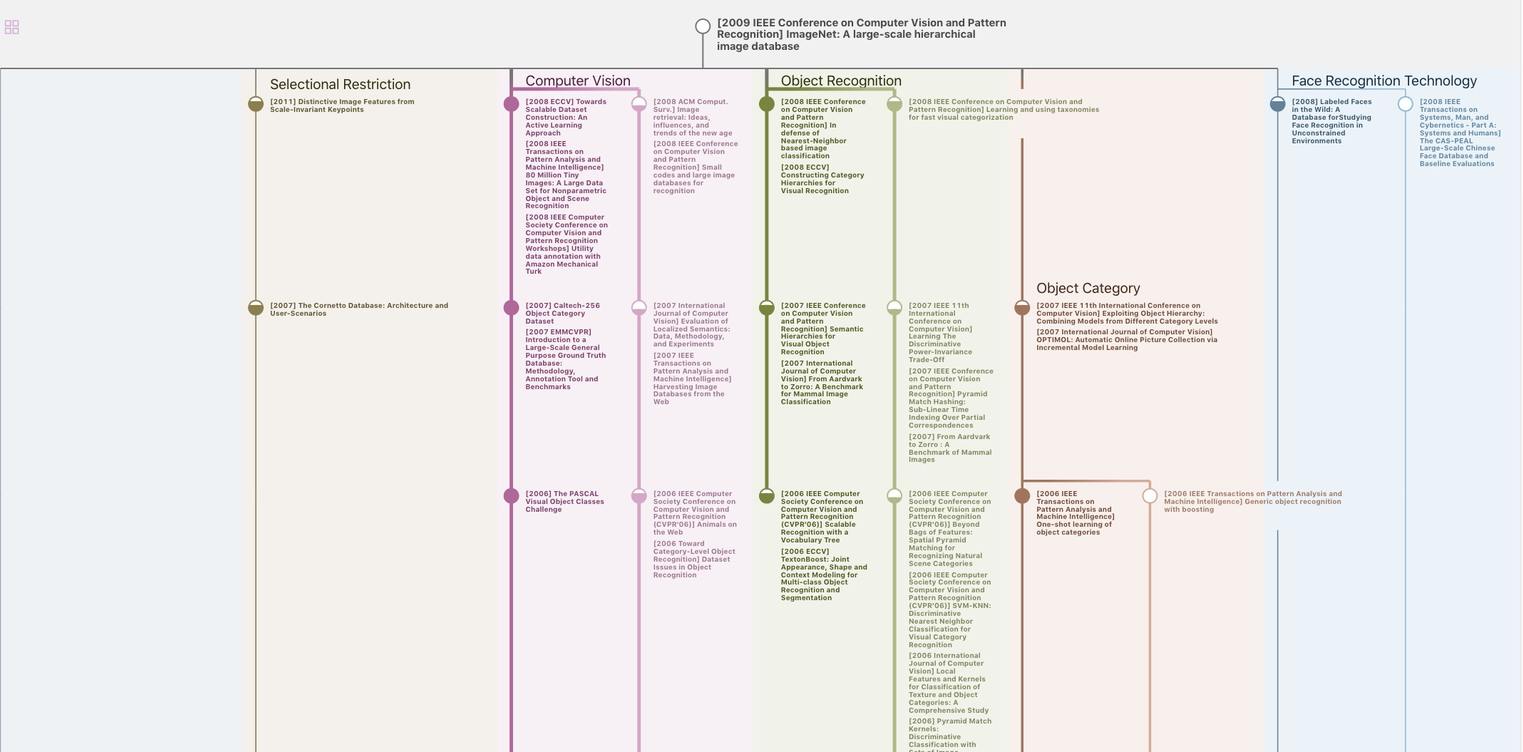
生成溯源树,研究论文发展脉络
Chat Paper
正在生成论文摘要