Weight-Specific-Decoder Attention Model to Solve Multiobjective Combinatorial Optimization Problems.
SMC(2022)
摘要
The multiobjective combinatorial optimization problems (MOCOPs) have a wide range of real-world applications. Designing an effective algorithm has an important and practical significance. Due to the huge search space and limited time, it is generally difficult to obtain the optimal solution of this kind of problem by traditional exact and heuristic algorithms. Recently, learning-based algorithms have achieved good results in solving MOCOPs, but the quality and diversity of found solutions can be further improved. In this paper, we propose a Weight-Specific-Decoder Attention Model (WSDAM) to better approximate the whole Pareto set. It embeds a weight-adaptive layer into the decoder to concentrate on the information of different weight vectors. During the model training, the weight vector is sampled from the Dirichlet distribution, which can further strengthen the learning of boundary solutions. We evaluate our method on two classic MOCOPs, i.e., the multiobjective traveling salesman problem (MOTSP) and multiobjective capacitated vehicle routing problem (MOCVRP). The experimental results show that our proposed method outperforms current state-of-the-art learning-based methods in both solution quality and generalization ability.
更多查看译文
关键词
multiobjective combinatorial optimization problems,attention,weight-specific-decoder
AI 理解论文
溯源树
样例
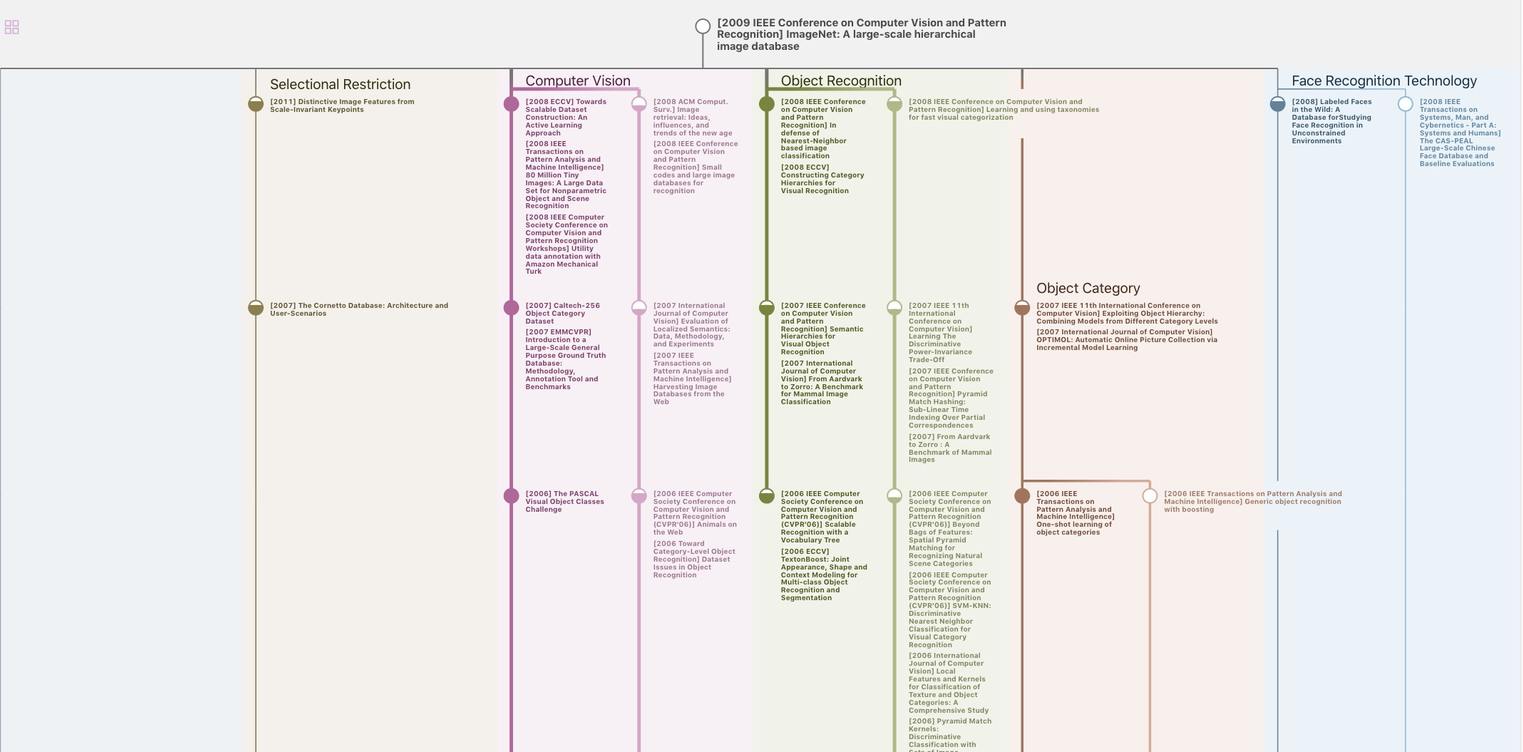
生成溯源树,研究论文发展脉络
Chat Paper
正在生成论文摘要