Interpretable Convolutional Learning Classifier System (C-LCS) for higher dimensional datasets.
SMC(2022)
摘要
The purpose of this paper is to devise an interpretable hybrid classification model for Convolutional Neural Networks (CNN) and a Learning Classifier System (LCS). The presented hybrid system integrates the fundamental attributes from both types of these classifiers. In the proposed hybrid model CNN works as an automatic feature extractor, and LCS works to provide interpretable rule-based classification results. Although LCS has limitations working on higher dimensional datasets, we resolve this limitation by using CNN as a feature extractor. The other concept of the non-interpretability of CNN is addressed by using the LCS rule. Furthermore, our experiment with higher dimensional datasets like CIFAR-10 and Fashion-MNIST shows that extended LCS provides comparable performance to the standard neural network model while also providing interpretable results. We named this extended LCS method Convolutional Learning Classifier Cystem (C-LCS).
更多查看译文
关键词
higher dimensional datasets,learning,c-lcs
AI 理解论文
溯源树
样例
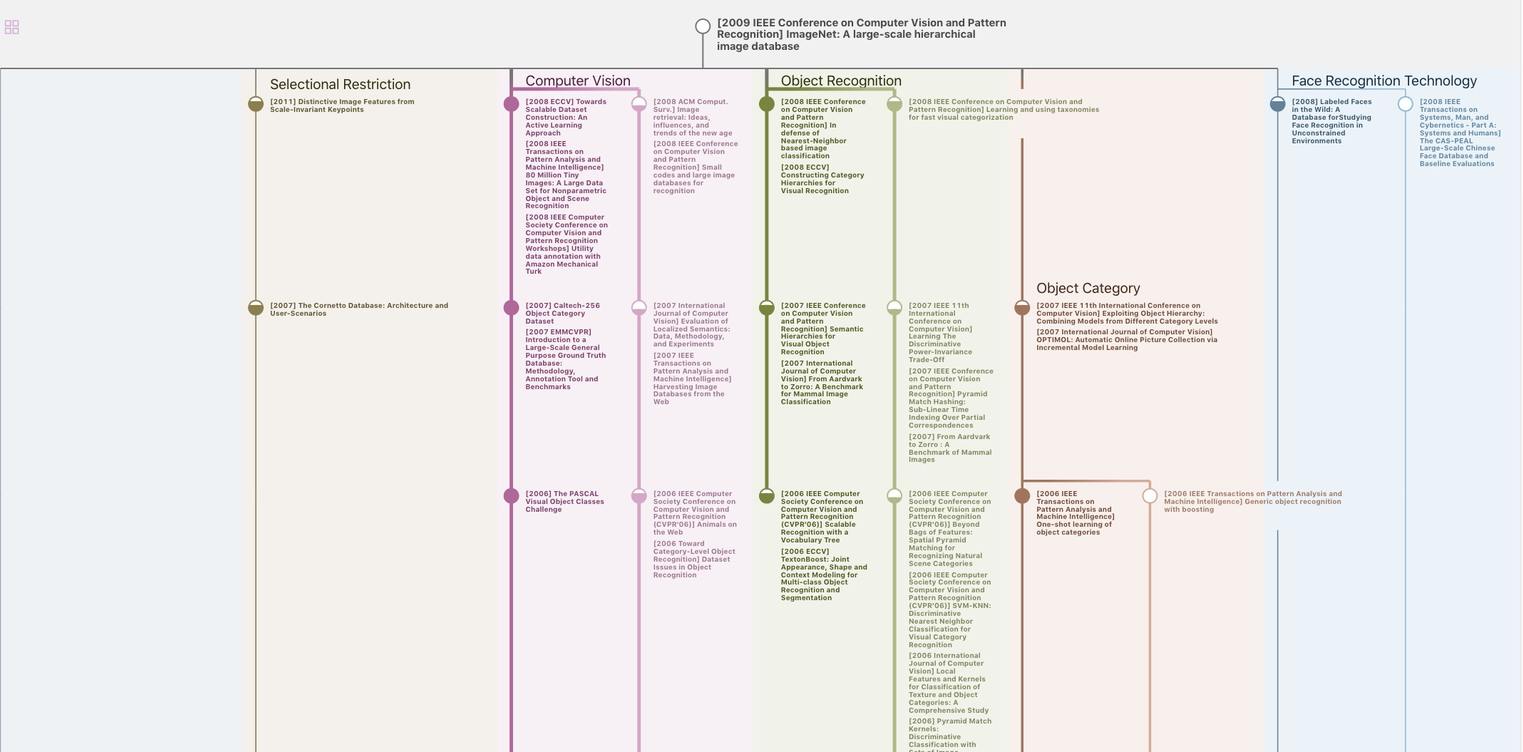
生成溯源树,研究论文发展脉络
Chat Paper
正在生成论文摘要