Connecting Quality Metrics to Deep Learning Accuracy for Image Fusion Methods
ICTC(2022)
摘要
A lot of human-generated data consist of images. While various tasks are becoming automated, reducing the complexity of image processing remains a challenge. At the same time, an image fusion algorithm can be applied before a convolutional neural network (CNN) as an image preprocessing method, where the image fusion combines incoming side-channel images into a single image. Thus, an image fusion can reduce the complexity of the conventional CNN task. However, traditional quality assessment functions (QAFs) for image fusion are a variety of calculation that does not provide a direct clue for the CNN accuracy of interest. In this study, we seek the correlation between QAFs and classification accuracy through CNN. The simulation result by training on differently color-fused CIFAR-10 datasets provides a possible standard to choose an image fusion method in the case of classifying fused images through a CNN. We expect the communication overhead to be decreased while using future image classification models in public.
更多查看译文
关键词
deep learning accuracy,quality metrics,deep learning
AI 理解论文
溯源树
样例
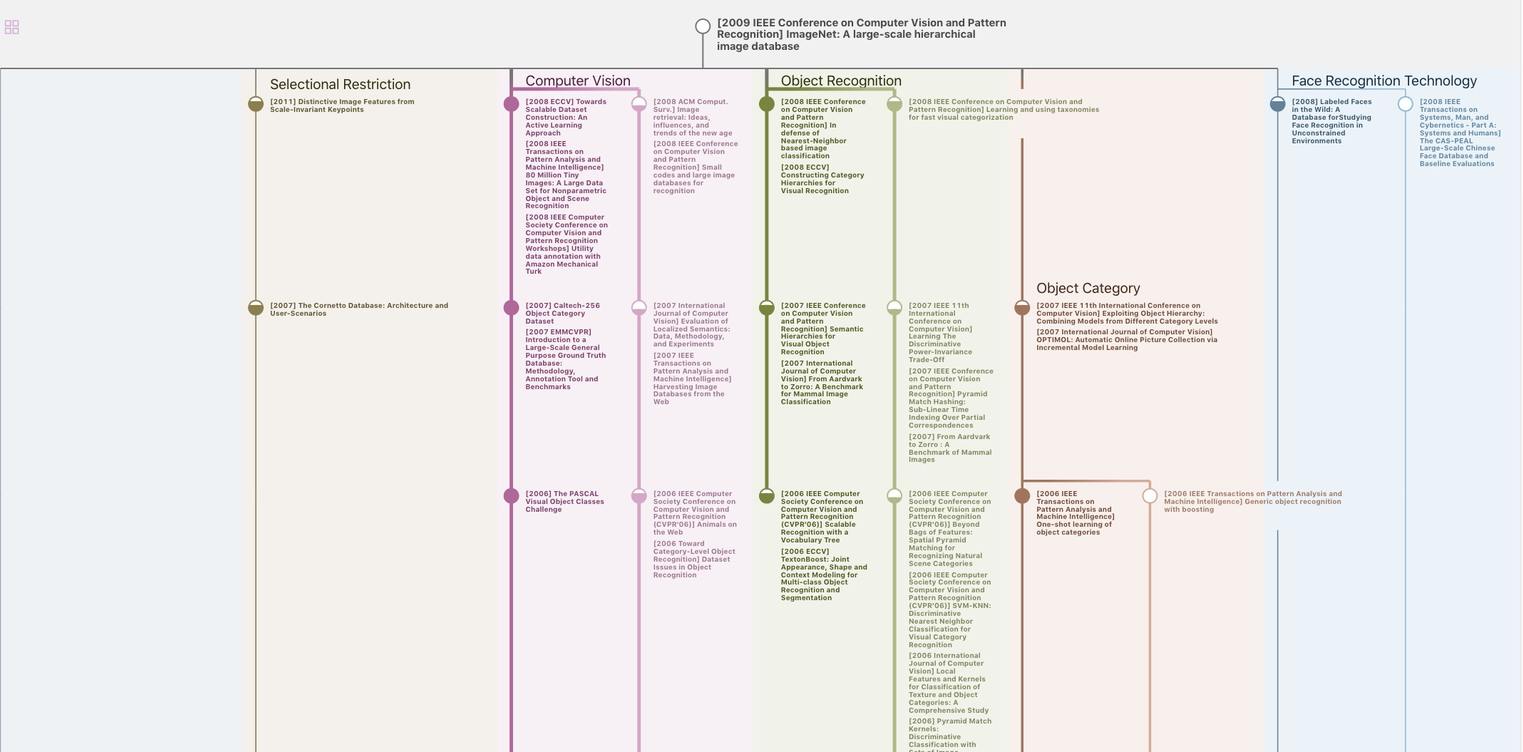
生成溯源树,研究论文发展脉络
Chat Paper
正在生成论文摘要