SNNIM: A 10T-SRAM based Spiking-Neural-Network-In-Memory architecture with capacitance computation.
ISCAS(2022)
摘要
Spiking-Neural-Networks (SNN) have natural advantages in high-speed signal processing and big data operation. However, due to the complex implementation of synaptic arrays, SNN based accelerators may face low area utilization and high energy consumption. Computing-InMemory (CIM) shows great potential in performing intensive and high energy efficient computations. In this work, we proposed a 10T-SRAM based Spiking-Neural-Network-InMemory architecture (SNNIM) with 28nm CMOS technology node. A compact 10T-SRAM bit-cell was developed to realize signed 5bit synapses arrays and configurable bias arrays (SYBIA). The soma array based standard 8T-SRAM (SMTA) stores the soma membrane voltage and the threshold value. A capacitance computation scheme (CCA) between them was proposed to support various SNN operations. The proposed SNNIM achieved energy efficiency of 25.18 TSyOPS/W. And the proposed SNNIM achieved 1.79+x better array efficiency compared with previous works.
更多查看译文
关键词
Spiking Neural Network,Computing In Memory (CIM),Neuromorphic hardware,Capacitance computation,JOT SRAM,Analog computation.
AI 理解论文
溯源树
样例
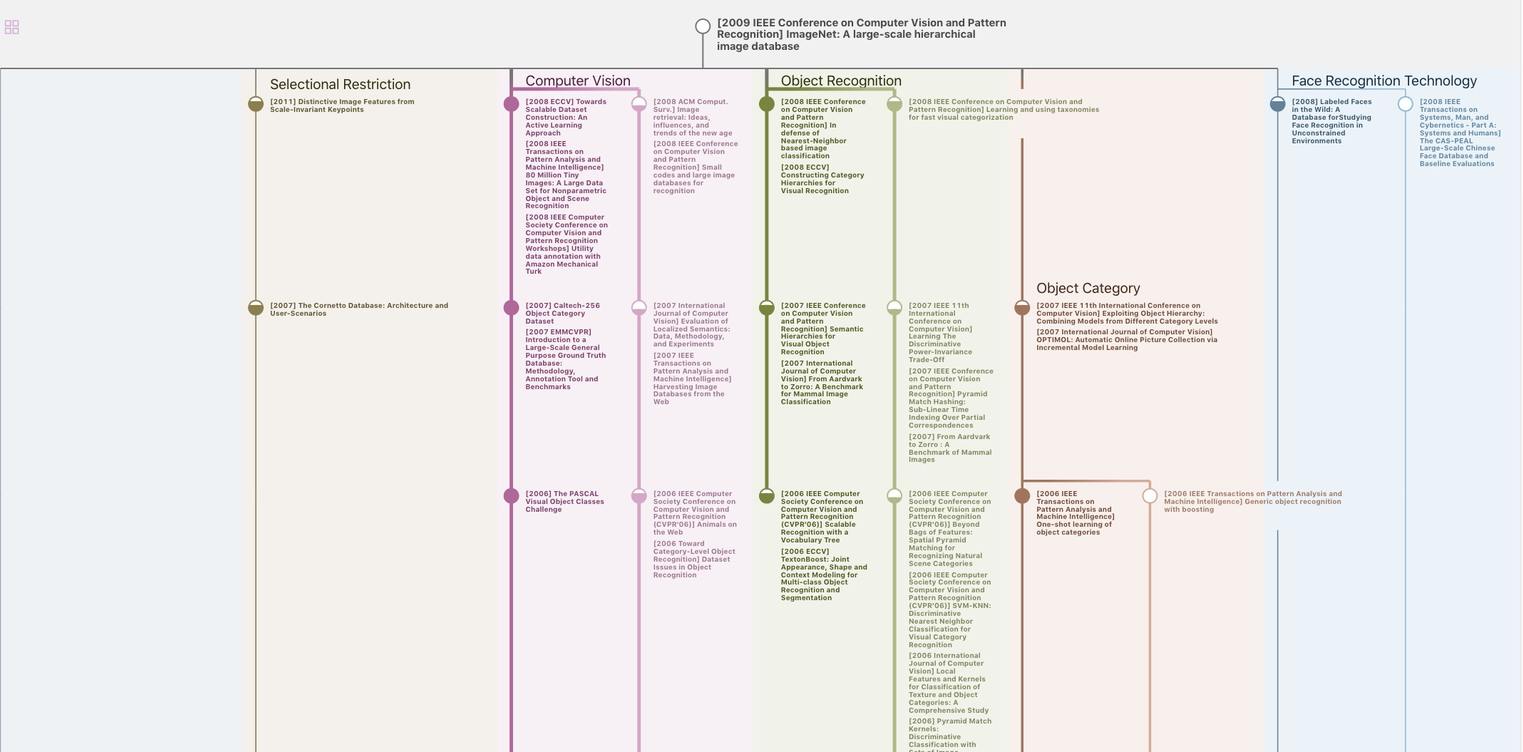
生成溯源树,研究论文发展脉络
Chat Paper
正在生成论文摘要