Joint Representation Learning for Anomaly Detection in Surveillance Videos.
ISCAS(2022)
摘要
Video anomaly detection in the unconstrained environment is challenging due to various background scenes, illuminations, and occlusions. Recent studies show that deep learning approaches can achieve remarkable performance on video anomaly detection. In this paper, we propose a joint representation learning structure for video anomaly detection. The proposed architecture extracts features from the object appearance and their associate motion features via different encoders based on ResNet network architecture. Our network architecture is designed to combine spatial and temporal features, which share the same decoder. Using a joint representation learning approach, the proposed architecture effectively learn both appearance and motion features to detect anomalies in various scene scenarios. The experiments on three benchmark datasets demonstrate the remarkable detection accuracy with respect to existing state-of-the-art methods, which achieve 96.5%, 86.9%, and 73.4% in UCSD Pedestrian, CHUK Avenue, and ShanghaiTech datasets, respectively.
更多查看译文
关键词
Terms Anomaly Detection, Generative Network, Video Anomaly, GAN, Autoencoder
AI 理解论文
溯源树
样例
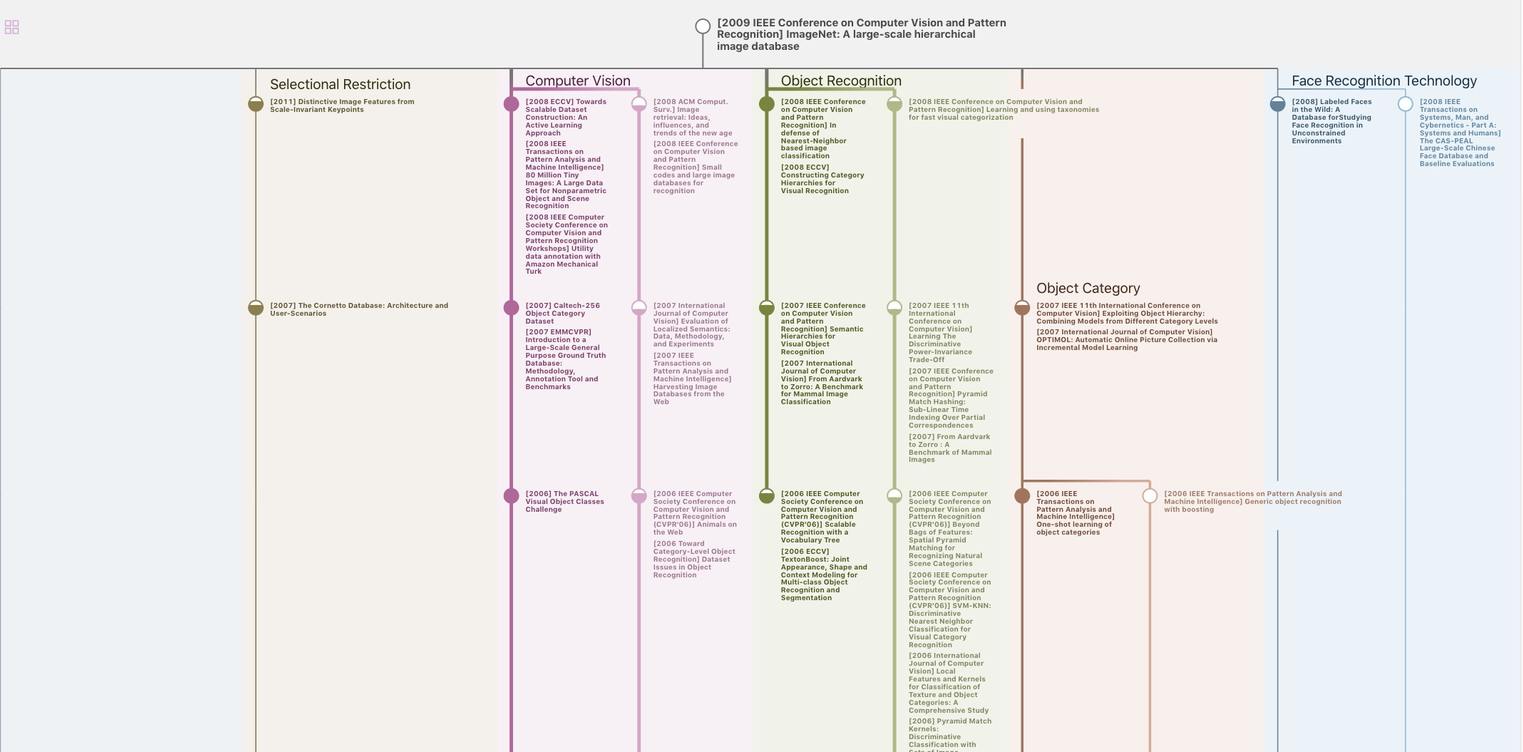
生成溯源树,研究论文发展脉络
Chat Paper
正在生成论文摘要