A Point Matching Strategy of 3D Loss Function for Single RGB Images Deep Mesh Reconstruction.
ISCAS(2022)
摘要
Recent the-state-of-the-art image-based three-dimensional (3D) reconstruction methods that represent 3D shapes mainly using triangular mesh because of its memory efficiency and ability to present surface detail of objects compared to voxel and point cloud. Previous works usually follow an encoding and decoding pattern. A deep neural network to extract the features from the picture and reconstruct the 3D structure. It is a typical supervised learning process, requiring loss function to supervise the training. No existing works directly calculate the loss between the reconstruction mesh and ground truth mesh. Instead, they indirectly used the Chamfer Distance (CD) between point clouds as the loss. Most of the previous works focus on the encoding and decoding parts instead of the loss and CD is used for all works. However, when CD is applied to two point clouds with the same number of points, some points can match any number of points in another point cloud, so some points will be less involved in calculating the loss function, which will reduce the utilization of information. Therefore, We propose a new point matching strategy to calculate the loss. The point matching strategy we proposed limits the maximum number of matches for each point, allowing more points to be more involved in the loss calculation, thereby improving the information utilization rate. Experiments on single view reconstruction (SVR) and auto-encoding methods show that this new loss method can replace CD in this type of works and has better training results and 3D reconstruction quality.
更多查看译文
关键词
3D reconstruction, image process, 3D loss function, triangular mesh
AI 理解论文
溯源树
样例
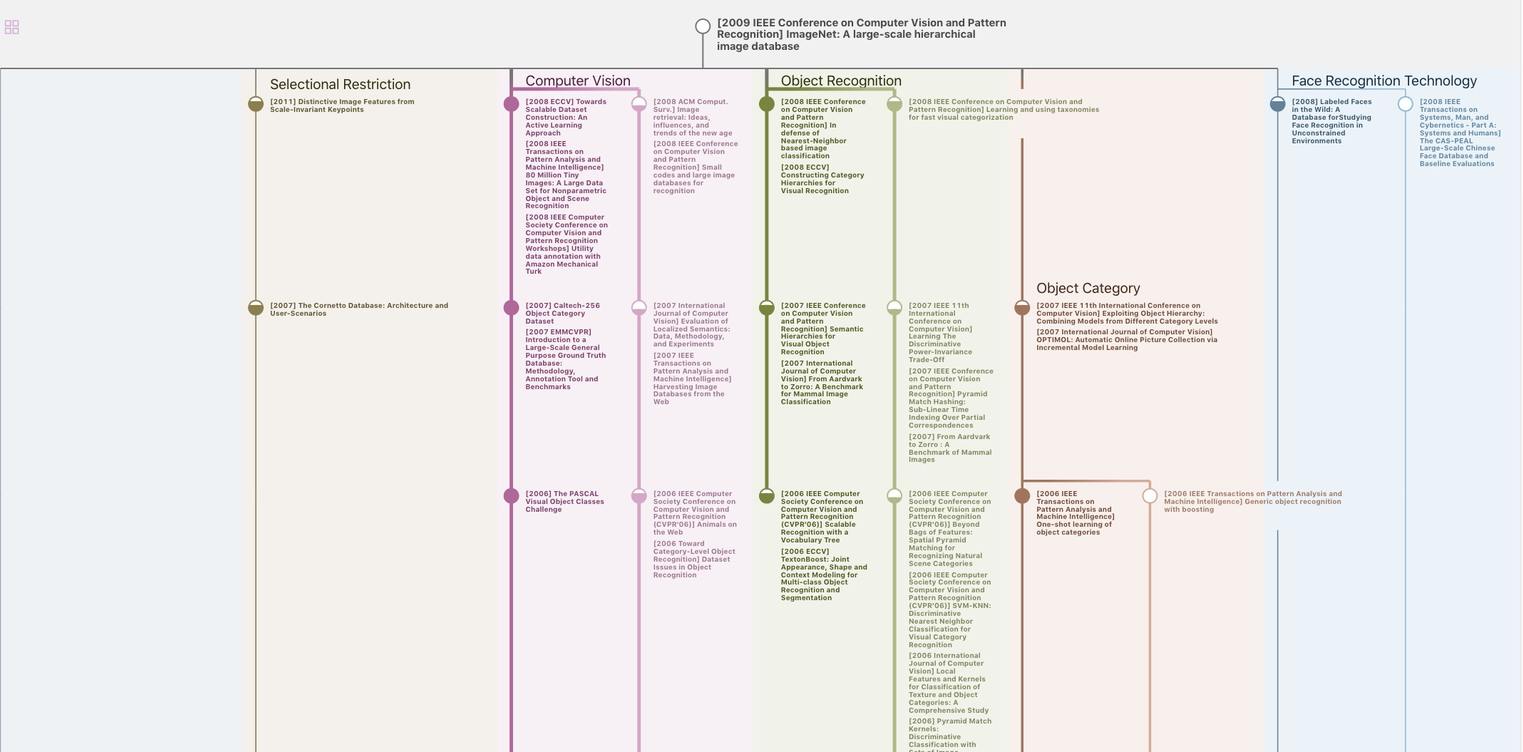
生成溯源树,研究论文发展脉络
Chat Paper
正在生成论文摘要