Histogram-Equalized Quantization for logic-gated Residual Neural Networks.
ISCAS(2022)
摘要
Adjusting the quantization according to the data or to the model loss seems mandatory to enable a high accuracy in the context of quantized neural networks. This work presents Histogram-Equalized Quantization (HEQ), an adaptive framework for linear and symmetric quantization. HEQ automatically adapts the quantization thresholds using a unique step size optimization. We empirically show that HEQ achieves state-of-the-art performances on CIFAR-10. Experiments on the STL-10 dataset even show that HEQ enables a proper training of our proposed logic-gated (OR, MUX) residual networks with a higher accuracy at a lower hardware complexity than previous work.
更多查看译文
关键词
CNN, quantized neural networks, histogram equalization, skip connections, logic-gated CNN
AI 理解论文
溯源树
样例
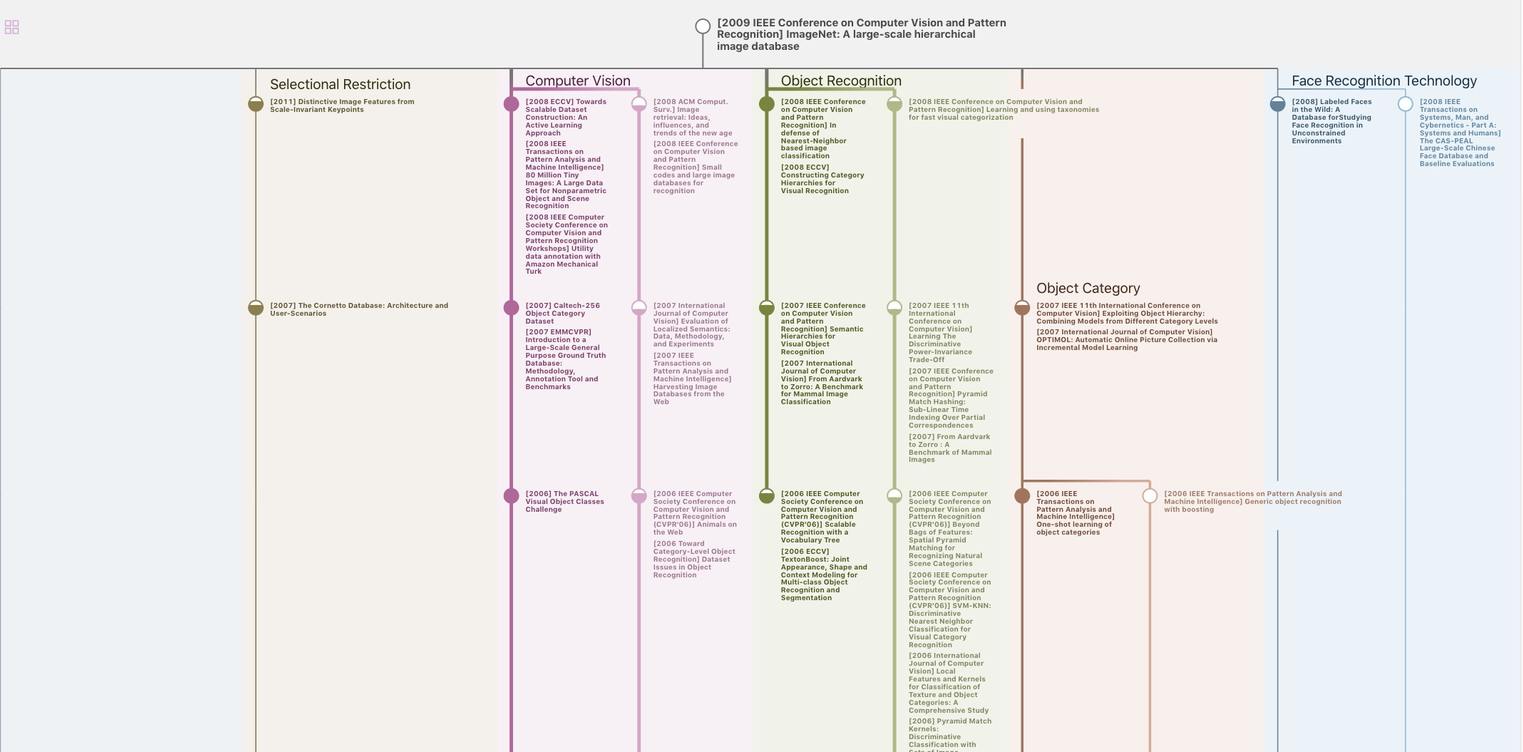
生成溯源树,研究论文发展脉络
Chat Paper
正在生成论文摘要